Communication Networks and Service Management in the Era of Artificial Intelligence and Machine Learning
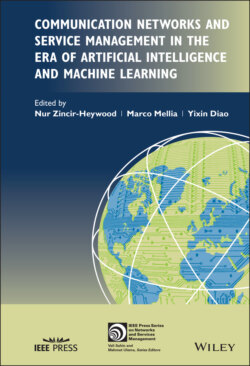
Реклама. ООО «ЛитРес», ИНН: 7719571260.
Оглавление
Группа авторов. Communication Networks and Service Management in the Era of Artificial Intelligence and Machine Learning
Table of Contents
List of Tables
List of Illustrations
Guide
Pages
Communication Networks and Service Management in the Era of Artificial Intelligence and Machine Learning
Editor Biographies
List of Contributors
Preface
Acknowledgments
Acronyms
1 Overview of Network and Service Management
1.1 Network and Service Management at Large
1.2 Data Collection and Monitoring Protocols
1.2.1 SNMP Protocol Family
1.2.2 Syslog Protocol
1.2.3 IP Flow Information eXport (IPFIX)
1.2.4 IP Performance Metrics (IPPM)
1.2.5 Routing Protocols and Monitoring Platforms
1.3 Network Configuration Protocol
1.3.1 Standard Configuration Protocols and Approaches
1.3.2 Proprietary Configuration Protocols
1.3.3 Integrated Platforms for Network Monitoring
1.4 Novel Solutions and Scenarios
1.4.1 Software‐Defined Networking – SDN
1.4.2 Network Functions Virtualization – NFV
Bibliography
2 Overview of Artificial Intelligence and Machine Learning
2.1 Overview
2.2 Learning Algorithms
2.2.1 Supervised Learning
2.2.2 Unsupervised Learning
2.2.3 Reinforcement Learning
2.3 Learning for Network and Service Management
Bibliography
Note
3 Managing Virtualized Networks and Services with Machine Learning
3.1 Introduction
3.2 Technology Overview
3.2.1 Virtualization of Network Functions
3.2.1.1 Resource Partitioning
3.2.1.2 Virtualized Network Functions
3.2.2 Link Virtualization
3.2.2.1 Physical Layer Partitioning
3.2.2.2 Virtualization at Higher Layers
3.2.3 Network Virtualization
3.2.4 Network Slicing
3.2.5 Management and Orchestration
3.3 State‐of‐the‐Art. 3.3.1 Network Virtualization
3.3.2 Network Functions Virtualization. 3.3.2.1 Placement
3.3.2.2 Scaling
3.3.3 Network Slicing. 3.3.3.1 Admission Control
3.3.3.2 Resource Allocation
3.4 Conclusion and Future Direction
3.4.1 Intelligent Monitoring
3.4.2 Seamless Operation and Maintenance
3.4.3 Dynamic Slice Orchestration
3.4.4 Automated Failure Management
3.4.5 Adaptation and Consolidation of Resources
3.4.6 Sensitivity to Heterogeneous Hardware
3.4.7 Securing Machine Learning
Bibliography
4 Self‐Managed 5G Networks1
4.1 Introduction
4.2 Technology Overview
4.2.1 RAN Virtualization and Management
4.2.2 Network Function Virtualization
4.2.3 Data Plane Programmability
4.2.4 Programmable Optical Switches
4.2.5 Network Data Management
4.3 5G Management State‐of‐the‐Art
4.3.1 RAN resource management. 4.3.1.1 Context‐Based Clustering and Profiling for User and Network Devices
4.3.1.2 ‐Learning Based RAN Resource Allocation
4.3.1.3 vrAIn: AI‐Assisted Resource Orchestration for Virtualized Radio Access Networks
4.3.2 Service Orchestration
4.3.3 Data Plane Slicing and Programmable Traffic Management
4.3.4 Wavelength Allocation
4.3.5 Federation
4.4 Conclusions and Future Directions
Bibliography
Notes
5 AI in 5G Networks: Challenges and Use Cases
5.1 Introduction
5.2 Background
5.2.1 ML in the Networking Context
5.2.2 ML in Virtualized Networks
5.2.3 ML for QoE Assessment and Management
5.3 Case Studies
5.3.1 QoE Estimation and Management
5.3.1.1 Main Challenges
5.3.1.2 Methodology
5.3.1.3 Results and Guidelines
5.3.2 Proactive VNF Deployment
5.3.2.1 Problem Statement and Main Challenges
5.3.2.2 Methodology
5.3.2.3 Evaluation Results and Guidelines
5.3.3 Multi‐service, Multi‐domain Interconnect
5.4 Conclusions and Future Directions
Bibliography
Note
6 Machine Learning for Resource Allocation in Mobile Broadband Networks
6.1 Introduction
6.2 ML in Wireless Networks
6.2.1 Supervised ML
6.2.1.1 Classification Techniques
6.2.1.2 Regression Techniques
6.2.2 Unsupervised ML
6.2.2.1 Clustering Techniques
6.2.2.2 Soft Clustering Techniques
6.2.3 Reinforcement Learning
6.2.4 Deep Learning
6.2.5 Summary
6.3 ML‐Enabled Resource Allocation
6.3.1 Power Control. 6.3.1.1 Overview
6.3.1.2 State‐of‐the‐Art
6.3.1.3 Lessons Learnt
6.3.2 Scheduling. 6.3.2.1 Overview
6.3.2.2 State‐of‐the‐Art
6.3.2.3 Lessons Learnt
6.3.3 User Association. 6.3.3.1 Overview
6.3.3.2 State‐of‐the‐Art
6.3.3.3 Lessons Learnt
6.3.4 Spectrum Allocation. 6.3.4.1 Overview
6.3.4.2 State‐of‐the‐Art
6.3.4.3 Lessons Learnt
6.4 Conclusion and Future Directions
6.4.1 Transfer Learning
6.4.2 Imitation Learning
6.4.3 Federated‐Edge Learning
6.4.4 Quantum Machine Learning
Bibliography
Note
7 Reinforcement Learning for Service Function Chain Allocation in Fog Computing
7.1 Introduction
7.2 Technology Overview
7.2.1 Fog Computing (FC)
7.2.2 Resource Provisioning
7.2.3 Service Function Chaining (SFC)
7.2.4 Micro‐service Architecture
7.2.5 Reinforcement Learning (RL)
7.3 State‐of‐the‐Art
7.3.1 Resource Allocation for Fog Computing
7.3.2 ML Techniques for Resource Allocation
7.3.3 RL Methods for Resource Allocation
7.4 A RL Approach for SFC Allocation in Fog Computing
7.4.1 Problem Formulation
7.4.2 Observation Space
7.4.3 Action Space
7.4.4 Reward Function
7.4.5 Agent
7.5 Evaluation Setup
7.5.1 Fog–Cloud Infrastructure
7.5.2 Environment Implementation
7.5.3 Environment Configuration
7.6 Results
7.6.1 Static Scenario
7.6.2 Dynamic Scenario
7.7 Conclusion and Future Direction
Bibliography
Note
8 Designing Algorithms for Data‐Driven Network Management and Control: State‐of‐the‐Art and Challenges1
8.1 Introduction
8.1.1 Contributions
8.1.2 Exemplary Network Use Case Study
8.2 Technology Overview
8.2.1 Data‐Driven Network Optimization
8.2.2 Optimization Problems over Graphs
8.2.3 From Graphs to ML/AI Input
8.2.4 End‐to‐End Learning
8.3 Data‐Driven Algorithm Design: State‐of‐the Art
8.3.1 Data‐Driven Optimization in General
8.3.2 Data‐Driven Network Optimization
8.3.3 Non‐graph Related Problems
8.4 Future Direction
8.4.1 Data Production and Collection
8.4.2 ML and AI Advanced Algorithms for Network Management with Performance Guarantees
8.5 Summary
Acknowledgments
Bibliography
Note
9 AI‐Driven Performance Management in Data‐Intensive Applications
9.1 Introduction
9.2 Data‐Processing Frameworks
9.2.1 Apache Storm
9.2.2 Hadoop MapReduce
9.2.3 Apache Spark
9.2.4 Apache Flink
9.3 State‐of‐the‐Art
9.3.1 Optimal Configuration
9.3.1.1 Traditional Approaches
9.3.1.2 AI Approaches
9.3.1.3 Example: AI‐Based Optimal Configuration
9.3.2 Performance Anomaly Detection
9.3.2.1 Traditional Approaches
9.3.2.2 AI Approaches
9.3.2.3 Example: ANNs‐Based Anomaly Detection
9.3.3 Load Prediction
9.3.3.1 Traditional Approaches
9.3.3.2 AI Approaches
9.3.4 Scaling Techniques
9.3.4.1 Traditional Approaches
9.3.4.2 AI Approaches
9.3.5 Example: RL‐Based Auto‐scaling Policies
9.4 Conclusion and Future Direction
Bibliography
Notes
10 Datacenter Traffic Optimization with Deep Reinforcement Learning
10.1 Introduction
10.2 Technology Overview
10.2.1 Deep Reinforcement Learning (DRL)
10.2.2 Applying ML to Networks
10.2.3 Traffic Optimization Approaches in Datacenter
10.2.4 Example: DRL for Flow Scheduling
10.2.4.1 Flow Scheduling Problem
10.2.4.2 DRL Formulation
10.2.4.3 DRL Algorithm
10.3 State‐of‐the‐Art: AuTO Design
10.3.1 Problem Identified
10.3.2 Overview
10.3.3 Peripheral System
10.3.3.1 Enforcement Module
10.3.3.2 Monitoring Module
10.3.4 Central System
10.3.5 DRL Formulations and Solutions
10.3.5.1 Optimizing MLFQ Thresholds
10.3.5.2 Optimizing Long Flows
10.4 Implementation
10.4.1 Peripheral System
10.4.1.1 Monitoring Module (MM):
10.4.1.2 Enforcement Module (EM):
10.4.2 Central System
10.4.2.1 sRLA
10.4.2.2 lRLA
10.5 Experimental Results
10.5.1 Setting
10.5.2 Comparison Targets
10.5.3 Experiments. 10.5.3.1 Homogeneous Traffic
10.5.3.2 Spatially Heterogeneous Traffic
10.5.3.3 Temporally and Spatially Heterogeneous Traffic
10.5.4 Deep Dive
10.5.4.1 Optimizing MLFQ Thresholds using DRL
10.5.4.2 Optimizing Long Flows using DRL
10.5.4.3 System Overhead
10.6 Conclusion and Future Directions
Bibliography
Notes
11 The New Abnormal: Network Anomalies in the AI Era
11.1 Introduction
11.2 Definitions and Classic Approaches
11.2.1 Definitions
11.2.2 Anomaly Detection: A Taxonomy
11.2.3 Problem Characteristics
11.2.4 Classic Approaches
11.3 AI and Anomaly Detection
11.3.1 Methodology
11.3.2 Deep Neural Networks
11.3.3 Representation Learning
11.3.4 Autoencoders
11.3.5 Generative Adversarial Networks
11.3.6 Reinforcement Learning
11.3.7 Summary and Takeaways
11.4 Technology Overview
11.4.1 Production‐Ready Tools
11.4.2 Research Alternatives
11.4.3 Summary and Takeaways
11.5 Conclusions and Future Directions
Bibliography
Notes
12 Automated Orchestration of Security Chains Driven by Process Learning*
12.1 Introduction
12.2 Related Work
12.2.1 Chains of Security Functions
12.2.2 Formal Verification of Networking Policies
12.3 Background
12.3.1 Flow‐Based Detection of Attacks
12.3.2 Programming SDN Controllers
12.4 Orchestration of Security Chains
12.5 Learning Network Interactions
12.6 Synthesizing Security Chains
12.7 Verifying Correctness of Chains
12.7.1 Packet Routing
12.7.2 Shadowing Freedom and Consistency
12.8 Optimizing Security Chains
12.9 Performance Evaluation
12.9.1 Complexity of Security Chains
12.9.2 Response Times
12.9.3 Accuracy of Security Chains
12.9.4 Overhead Incurred by Deploying Security Chains
12.10 Conclusions
Bibliography
Notes
13 Architectures for Blockchain‐IoT Integration1
13.1 Introduction
13.1.1 Blockchain Basics
13.1.2 Internet‐of‐Things (IoT) Basics
13.2 Blockchain‐IoT Integration (BIoT)
13.2.1 BIoT Potentials
13.2.2 BIoT Use Cases
13.2.3 BIoT Challenges
13.2.3.1 Scalability
13.2.3.2 Security
13.2.3.3 Energy Efficiency
13.2.3.4 Manageability
13.3 BIoT Architectures
13.3.1 Cloud, Fog, and Edge‐Based Architectures
13.3.2 Software‐Defined Architectures
13.3.3 A Potential Standard BIoT Architecture
13.4 Summary and Considerations
Bibliography
Note
Index. a
b
c
d
e
f
g
h
i
j
k
l
m
n
o
p
q
r
s
t
u
v
w
y
z
WILEY END USER LICENSE AGREEMENT
Отрывок из книги
Edited by Nur Zincir–Heywood, Marco Mellia, and Yixin Diao
.....
Hong Kong SAR, China
Lina Magoula
.....