Applied Smart Health Care Informatics
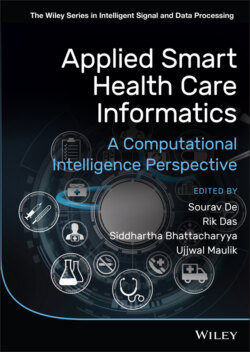
Реклама. ООО «ЛитРес», ИНН: 7719571260.
Оглавление
Группа авторов. Applied Smart Health Care Informatics
Table of Contents
List of Tables
List of Illustrations
Guide
Pages
Series Preface Dr. Siddhartha Bhattacharyya, CHRIST (Deemed to be University), Bengaluru, India (Series Editor)
Applied Smart Health Care Informatics. A Computational Intelligence Perspective
Preface
About the Editors
List of Contributors
1 An Overview of Applied Smart Health Care Informatics in the Context of Computational Intelligence
1.1 Introduction
1.2 Big Data Analytics in Healthcare
1.3 AI in Healthcare
1.4 Cloud Computing in Healthcare
1.5 IoT in Healthcare
1.6 Conclusion
References
Note
2 A Review on Deep Learning Method for Lung Cancer Stage Classification Using PET‐CT
2.1 Introduction
2.1.1 Scope of the Research
2.1.2 TNM Staging
2.1.2.1 TNM Descriptors for Staging per IASLC Guidelines
2.1.2.2 PET‐CT Scan in Lung Cancer Imaging
2.2 Related Works
2.2.1 Artificial Intelligence in Medical Imaging
2.2.2 Classification for Medical Imaging
2.2.2.1 Deep Learning
2.2.2.2 Image Classification Using Deep‐learning Techniques
2.3 Methods. 2.3.1 Transfer Learning
2.3.2 AlexNet
2.3.3 AlexNet Architecture
2.3.4 Experimental Setup
2.3.4.1 Image Processing
2.3.4.2 Data Augmentation
2.3.4.3 Training and Validation
2.4 Results and Discussion. 2.4.1 Primary Tumor (T)
2.4.2 Metastasis (M)
2.4.3 Lymph Node (N)
2.4.4 Classification Accuracy of AlexNet
2.4.5 Comparative Analysis
2.4.6 Limitations
2.5 Conclusion
References
Note
3 Formal Methods for the Security of Medical Devices 1
3.1 Introduction
3.1.1 Pacemaker Security
3.1.2 Overview
3.2 Background: Cardiac Pacemakers
3.2.1 Pacemakers
3.2.1.1 Operation of a DDD Mode Pacemaker
3.2.2 The Cardiac System
3.2.2.1 Electrograms and Electrocardiograms
3.3 State of the Art, Formal Verification Techniques
3.3.1 Formal Verification Techniques
3.3.1.1 Static Verification Techniques
3.3.1.2 Dynamic Verification Techniques
3.3.2 Runtime Verification
3.3.2.1 A Brief Overview of Some Runtime Verification Frameworks
3.3.3 Correcting Execution of a System at Runtime (Runtime Enforcement)
3.3.3.1 Runtime Enforcement of Untimed Properties
3.3.3.2 Runtime Enforcement Approaches for Timed Properties
3.4 Formal Runtime‐Based Approaches for Medical Device Security
3.4.1 Overview of the Approach
3.4.2 Mapping EGM Properties to ECG Properties
3.4.3 Security of Pacemakers Using Runtime Verification
3.4.3.1 Timed Words, Timed Languages, and Defining Timed Properties
3.4.3.2 Runtime Verification Monitor
3.4.3.3 Architecture of the Monitoring System
Remark 3.1
3.4.3.4 Implementation of the ECG Processing and RV Monitor Modules
3.4.3.5 Summary of Experiments and Results
3.4.4 Securing Pacemakers with Runtime Enforcement Hardware
3.4.4.1 Preliminaries: Words, Languages, and Defining Properties as DTA
Remark 3.2
3.4.4.2 Runtime Enforcement Monitor
3.4.4.3 Verification of the Enforcer Hardware
3.4.4.4 How Does the Enforcer Prevent Security Attacks?
3.4.4.5 Summary of Experiments and Results
3.5 Summary
References
Notes
4 Integrating Two Deep Learning Models to Identify Gene Signatures in Head and Neck Cancer from Multi‐Omics Data
4.1 Introduction
4.2 Related Work
4.3 Materials and Methods
4.3.1 A Brief Introduction of the Capsule Network
4.3.2 An Introduction to Autoencoders
4.4 Results
4.4.1 Data Set Details
4.4.1.1 Gene Expression Data (Illumina Hiseq)
4.4.1.2 Human Methylation 450K
4.4.2 Architecture of Autoencoder Model
4.4.3 Architecture of the Proposed Capsule Network Model
4.4.4 Validation of Two Deep Learning Models
4.4.5 Gene Signatures from Primary Capsules
4.5 Discussion
Acknowledgments
References
Note
5 A Review of Computational Learning and IoT Applications to High‐Throughput Array‐Based Sequencing and Medical Imaging Data in Drug Discovery and Other Health Care Systems
5.1 Introduction
5.2 Biological Terms
5.3 Single‐Cell Sequencing (scRNA‐seq) Data
5.3.1 Computational Methods for Interpreting scRNA‐seq Data. 5.3.1.1 Visualizing and Clustering Cells
5.3.1.2 Inference and Branching Analysis of Cellular Trajectory
5.3.1.3 Identifying Highly Variable Genes
5.3.1.4 Identifying Marker and Differentially Expressed Genes
5.4 Methods of Multi‐Omic Data Integration
5.4.1 Unsupervised Data Integration Methods
5.4.1.1 Matrix Factorization Methods
5.4.1.2 Bayesian Methods
5.4.1.3 Network‐Based Methods
5.4.1.4 Multi‐Step Analysis and Multiple Kernel Learning
5.4.2 Supervised Data Integration
5.4.2.1 Network‐Based Methods
5.4.2.2 Multiple Kernel Learning
5.4.2.3 Multi‐Step Analysis
5.4.3 Semi‐Supervised Data Integration
5.4.3.1 GeneticInterPred
5.5 AI Drug Discovery
5.5.1 AI Primary Drug Screening. 5.5.1.1 Cell Sorting and Classification with Image Analysis
5.5.2 AI Secondary Drug Screening. 5.5.2.1 Physical Properties Predictions
5.5.2.2 Predictions of Bio‐Activity
5.5.2.3 Prediction of Toxicity
5.5.3 AI in Drug Design. 5.5.3.1 Prediction of Target Protein 3D Structures
5.5.3.2 Predicting Drug‐Protein Interactions
5.5.4 Planning Chemical Synthesis with AI. 5.5.4.1 Retro‐Synthesis Pathway Prediction
5.5.4.2 Reaction Yield Predictions and Reaction Mechanism Insights
5.6 Medical Imaging Data Analysis
5.6.1 Analysis: Radio‐Mic Quantification
5.6.2 Analysis: Bio‐Marker Identification
5.7 Applying IoT (Internet of Things) to Biomedical Research. 5.7.1 IoT and IoMT Applications for Healthcare and Well‐Being. 5.7.1.1 Wireless Medical Devices
5.8 Conclusions
Acknowledgments
References
Note
6 Association Rule Mining Based on Ethnic Groups and Classification using Super Learning1
6.1 Introduction
6.2 Background
6.3 Motivation and Contribution
6.4 Data Analysis
6.4.1 Data Description
6.4.2 Data Preprocessing
6.4.3 Further Preprocessing for Ethnic Group Rule Discovery with Multiple Consequences
6.4.3.1 Transaction‐Like Database for Association Rule
6.4.4 Classification Data Set
6.5 Methodology
6.5.1 Association Rule Mining
6.5.2 Super Learning
6.5.2.1 Ensemble or Super Learner Set‐Up
6.6 Experiments and Results
6.6.1 Rules Discovery
6.6.1.1 Rules of Breast Cancer Patients Based on Ethnic Groups
6.6.1.2 Interpreting Rules
6.6.2 Evaluation Criteria of Classification Model
6.6.2.1 Super Learner Results
6.6.3 Discussion
6.7 Conclusion and Future Work
References
Notes
7 Neuro‐Rough Hybridization for Recognition of Virus Particles from TEM Images1
7.1 Introduction
7.2 Existing Approaches for Virus Particle Classification
7.3 Proposed Algorithm
7.3.1 Extraction of Local Textural Features
7.3.2 Selection of Class‐Pair Relevant Features
7.3.3 Extraction of Discriminating Features
7.3.4 Classification
7.4 Experimental Results and Discussion
7.4.1 Experimental Setup
7.4.2 Methods Compared
7.4.3 Database Considered
7.4.4 Effectiveness of Proposed Approach
7.4.5 Comparative Performance Analysis
7.4.5.1 Comparison with Deep Architectures
7.4.5.2 Comparison with Existing Approaches
7.5 Conclusion
References
Notes
8 Neural Network Optimizers for Brain Tumor Image Detection
8.1 Introduction
8.2 Related Works
8.3 Background. 8.3.1 Types of Neural Networks
8.3.2 Tunable Elements of Neural Networks
8.3.2.1 Basic Parameters
8.3.2.2 Hyperparameters
8.3.2.3 Regularization Techniques
8.3.2.4 Neural Network Optimizers
8.4 Case Study ‐ Brain Tumor Detection. 8.4.1 Methodology
8.4.2 Data Sets and Metrics
8.4.3 Results and Discussion
8.5 Conclusion
References
Note
9 Abnormal Slice Classification from MRI Volumes using the Bilateral Symmetry of Human Head Scans
9.1 Introduction. 9.1.1 MRIs of the Human Brain
9.1.2 Normal and Abnormal Slices
9.1.3 Background
9.1.3.1 Decision Tree Classifiers
9.1.3.2 K‐Nearest Neighbours (KNN) Classifiers
9.1.3.3 Support Vector Machine (SVM)
9.1.3.4 Naive Bayes
9.1.3.5 Artificial Neural Network (ANN)
9.1.3.6 Back‐Propagation Neural Network (BPN)
9.1.3.7 Random Forest Classifiers
9.2 Literature Review
9.3 Methodology
9.3.1 Preprocessing
9.3.2 Feature Extraction
9.3.3 Feature Selection
9.3.4 Classification
9.3.5 Cross‐Validation
9.3.6 Training Validation and Testing
9.4 Materials and Metrics
9.4.1 Confusion Matrix
9.5 Results and Discussion
9.6 Conclusion
References
Note
10 Conclusion
References
Note
Index. a
b
c
d
e
f
g
h
i
k
l
m
n
o
p
r
s
t
v
WILEY END USER LICENSE AGREEMENT
Отрывок из книги
The Intelligent Signal and Data Processing (ISDP) book series is aimed at fostering the field of signal and data processing, which encompasses the theory and practice of algorithms and hardware that convert signals produced by artificial or natural means into a form useful for a specific purpose. The signals might be speech, audio, images, video, sensor data, telemetry, electrocardiograms, or seismic data, among others. The possible application areas include transmission, display, storage, interpretation, classification, segmentation, or diagnosis. The primary objective of the ISDP book series is to evolve future‐generation scalable intelligent systems for faithful analysis of signals and data. ISDP is mainly intended to enrich the scholarly discourse on intelligent signal and image processing in different incarnations. ISDP will benefit a wide range of learners, including students, researchers, and practitioners. The student community can use the volumes in the series as reference texts to advance their knowledge base. In addition, the monographs will also come in handy to the aspiring researcher because of the valuable contributions both have made in this field. Moreover, both faculty members and data practitioners are likely to grasp depth of the relevant knowledge base from these volumes.
The series coverage will contain, not exclusively, the following:
.....
The last two decades have witnessed unprecedented advancements in computational techniques and artificial intelligence. These new developments are going to greatly impact biological data analysis for the health care system. In fact, the availability of large scale high‐throughput biomedical data sets offers a fertile ground for application of these AI‐based techniques in to extract valuable information that can be harnessed in the diagnosis and treatment of various diseases. Chapter 5 provides a comprehensive review of computational tools and online resources for high throughput analyses of biomedical data. It focuses on single‐cell RNA sequencing data, multi‐omics data integration, drug design with AI, medical imaging data analysis, and IoT. After providing a brief overview of the fundamental biological terms, a variety of research problems are described in the health care system and how various high throughput data can help solving them. Next, an in depth overview of machine learning techniques of computing and learning methods that can be used in a variety of sequencing data analyses is provided.
Cancer is one of the most devastating diseases worldwide. It affects nearly every household, although cancer types are prevalent in different geographical regions. One example is breast cancer, which is the most common type of cancer in women worldwide. Therefore, prevention strategies are needed to address this issue. Identifying risk factors of breast cancer is crucial since it allows physicians to acquaint them with the risks. Accordingly, physicians can recommend precautionary actions. In the first part of Chapter 6, the authors detail the discovery of significant rules for breast cancer patients, focusing on different ethnic groups. Predicting the risk of the occurrence of breast cancer is an essential issue for clinical oncologists. A reliable prediction will help oncologists and other clinicians in their decision‐making process and allow clinicians to choose the most reliable and evidence‐based treatment. In the second part of the chapter, a super learner or stacked ensemble technique is employed to the breast cancer data set obtained from the Breast Cancer Surveillance Consortium (BCSC) database. A comparison of the performance of the super learner and the individual base learners is conducted. The results of the first part of this study (rule extraction from breast cancer patients in distinct ethnic groups) found well‐known ethnic disparities in cancer prevalence. The experimental results revealed that the produced rules hold the highest confidence level. The crucial rules, which can be easily understood, are also interpreted.
.....