Spatial Analysis
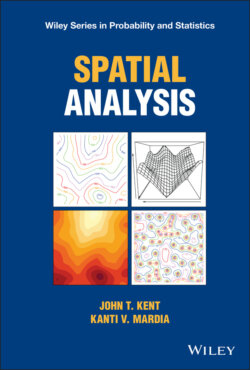
Реклама. ООО «ЛитРес», ИНН: 7719571260.
Оглавление
Kanti V. Mardia. Spatial Analysis
Table of Contents
List of Tables
List of Illustrations
Guide
Pages
Spatial Analysis
List of Figures
List of Tables
Preface
List of Notation and Terminology
1 Introduction. 1.1 Spatial Analysis
1.2 Presentation of the Data
1.3 Objectives
1.4 The Covariance Function and Semivariogram
1.4.1 General Properties
1.4.2 Regularly Spaced Data
1.4.3 Irregularly Spaced Data
1.5 Behavior of the Sample Semivariogram
1.6 Some Special Features of Spatial Analysis
Exercises
2 Stationary Random Fields. 2.1 Introduction
2.2 Second Moment Properties
2.3 Positive Definiteness and the Spectral Representation
2.4 Isotropic Stationary Random Fields
2.5 Construction of Stationary Covariance Functions
2.6 Matérn Scheme
2.7 Other Examples of Isotropic Stationary Covariance Functions
2.8 Construction of Nonstationary Random Fields
2.8.1 Random Drift
2.8.2 Conditioning
2.9 Smoothness
2.10 Regularization
2.11 Lattice Random Fields
2.12 Torus Models
2.12.1 Models on the Continuous Torus
2.12.2 Models on the Lattice Torus
2.13 Long‐range Correlation
2.14 Simulation. 2.14.1 General Points
2.14.2 The Direct Approach
2.14.3 Spectral Methods
2.14.4 Circulant Methods
Exercises
3 Intrinsic and Generalized Random Fields. 3.1 Introduction
3.2 Intrinsic Random Fields of Order
3.3 Characterizations of Semivariograms
3.4 Higher Order Intrinsic Random Fields
3.5 Registration of Higher Order Intrinsic Random Fields
3.6 Generalized Random Fields
3.7 Generalized Intrinsic Random Fields of Intrinsic Order
3.8 Spectral Theory for Intrinsic and Generalized Processes
3.9 Regularization for Intrinsic and Generalized Processes
3.10 Self‐Similarity
3.11 Simulation
3.11.1 General Points
3.11.2 The Direct Method
3.11.3 Spectral Methods
3.12 Dispersion Variance
Exercises
4 Autoregression and Related Models. 4.1 Introduction
4.2 Background
4.3 Moving Averages
4.3.1 Lattice Case
4.3.2 Continuously Indexed Case
4.4 Finite Symmetric Neighborhoods of the Origin in
4.5 Simultaneous Autoregressions (SARs) 4.5.1 Lattice Case
4.5.2 Continuously Indexed Random Fields
4.6 Conditional Autoregressions (CARs)
4.6.1 Stationary CARs
4.6.2 Iterated SARs and CARs
4.6.3 Intrinsic CARs
4.6.4 CARs on a Lattice Torus
4.6.5 Finite Regions
4.7 Limits of CAR Models Under Fine Lattice Spacing
4.8 Unilateral Autoregressions for Lattice Random Fields
4.8.1 Half‐spaces in
4.8.2 Unilateral Models
4.8.3 Quadrant Autoregressions
4.9 Markov Random Fields (MRFs) 4.9.1 The Spatial Markov Property
4.9.2 The Subset Expansion of the Negative Potential Function
4.9.3 Characterization of Markov Random Fields in Terms of Cliques
4.9.4 Auto‐models
4.10 Markov Mesh Models
4.10.1 Validity
4.10.2 Marginalization
4.10.3 Markov Random Fields
4.10.4 Usefulness
Exercises
5 Estimation of Spatial Structure. 5.1 Introduction
5.2 Patterns of Behavior
5.2.1 One‐dimensional Case
5.2.2 Two‐dimensional Case
5.2.3 Nugget Effect
5.3 Preliminaries
5.3.1 Domain of the Spatial Process
5.3.2 Model Specification
5.3.3 Spacing of Data
5.4 Exploratory and Graphical Methods
5.5 Maximum Likelihood for Stationary Models
5.5.1 Maximum Likelihood Estimates – Known Mean
5.5.2 Maximum Likelihood Estimates – Unknown Mean
5.5.3 Fisher Information Matrix and Outfill Asymptotics
5.6 Parameterization Issues for the Matérn Scheme
5.7 Maximum Likelihood Examples for Stationary Models
5.8 Restricted Maximum Likelihood (REML)
5.9 Vecchia's Composite Likelihood
5.10 REML Revisited with Composite Likelihood
5.11 Spatial Linear Model
5.11.1 MLEs
5.11.2 Outfill Asymptotics for the Spatial Linear Model
5.12 REML for the Spatial Linear Model
5.13 Intrinsic Random Fields
5.14 Infill Asymptotics and Fractal Dimension
Exercises
6 Estimation for Lattice Models
6.1 Introduction
6.2 Sample Moments
6.3 The AR(1) Process on
6.4 Moment Methods for Lattice Data
6.4.1 Moment Methods for Unilateral Autoregressions (UARs)
6.4.2 Moment Estimators for Conditional Autoregression (CAR) Models
6.5 Approximate Likelihoods for Lattice Data
6.6 Accuracy of the Maximum Likelihood Estimator
6.7 The Moment Estimator for a CAR Model
Exercises
7 Kriging. 7.1 Introduction
7.2 The Prediction Problem
7.3 Simple Kriging
7.4 Ordinary Kriging
7.5 Universal Kriging
7.6 Further Details for the Universal Kriging Predictor
7.6.1 Transfer Matrices
7.6.2 Projection Representation of the Transfer Matrices
7.6.3 Second Derivation of the Universal Kriging Predictor
7.6.4 A Bordered Matrix Equation for the Transfer Matrices
7.6.5 The Augmented Matrix Representation of the Universal Kriging Predictor
7.6.6 Summary
7.7 Stationary Examples
7.8 Intrinsic Random Fields. 7.8.1 Formulas for the Kriging Predictor and Kriging Variance
7.8.2 Conditionally Positive Definite Matrices
7.9 Intrinsic Examples
7.10 Square Example
7.11 Kriging with Derivative Information
7.12 Bayesian Kriging. 7.12.1 Overview
7.12.2 Details for Simple Bayesian Kriging
7.12.3 Details for Bayesian Kriging with Drift
7.13 Kriging and Machine Learning
7.14 The Link Between Kriging and Splines
7.14.1 Nonparametric Regression
7.14.2 Interpolating Splines
7.14.3 Comments on Interpolating Splines
7.14.4 Smoothing Splines
7.15 Reproducing Kernel Hilbert Spaces
7.16 Deformations
Exercises
8 Additional Topics. 8.1 Introduction
8.2 Log‐normal Random Fields
8.3 Generalized Linear Spatial Mixed Models (GLSMMs)
8.4 Bayesian Hierarchical Modeling and Inference
8.5 Co‐kriging
8.6 Spatial–temporal Models. 8.6.1 General Considerations
8.6.2 Examples
8.7 Clamped Plate Splines
8.8 Gaussian Markov Random Field Approximations
8.9 Designing a Monitoring Network
Exercises
Appendix A Mathematical Background
A.1 Domains for Sequences and Functions
A.2 Classes of Sequences and Functions
A.2.1 Functions on the Domain
A.2.2 Sequences on the Domain
A.2.3 Classes of Functions on the Domain
A.2.4 Classes of Sequences on the Domain , Where
A.3 Matrix Algebra
A.3.1 The Spectral Decomposition Theorem
A.3.2 Moore–Penrose Generalized Inverse
A.3.3 Orthogonal Projection Matrices
A.3.4 Partitioned Matrices
A.3.5 Schur Product
A.3.6 Woodbury Formula for a Matrix Inverse
A.3.7 Quadratic Forms
A.3.8 Toeplitz and Circulant Matrices
A.3.9 Tensor Product Matrices
A.3.10 The Spectral Decomposition and Tensor Products
A.3.11 Matrix Derivatives
A.4 Fourier Transforms
A.5 Properties of the Fourier Transform
A.6 Generalizations of the Fourier Transform
A.7 Discrete Fourier Transform and Matrix Algebra
A.7.1 DFT in Dimension
A.7.2 Properties of the Unitary Matrix ,
A.7.3 Circulant Matrices and the DFT,
A.7.4 The Case
A.7.5 The Periodogram
A.8 Discrete Cosine Transform (DCT)
A.8.1 One‐dimensional Case
A.8.2 The Case
A.8.3 Indexing for the Discrete Fourier and Cosine Transforms
A.9 Periodic Approximations to Sequences
A.10 Structured Matrices in Dimension
A.11 Matrix Approximations for an Inverse Covariance Matrix
A.11.1 The Inverse Covariance Function
A.11.2 The Toeplitz Approximation to
A.11.3 The Circulant Approximation to
A.11.4 The Folded Circulant Approximation to
A.11.5 Comments on the Approximations
A.11.6 Sparsity
A.12 Maximum Likelihood Estimation
A.12.1 General Considerations
A.12.2 The Multivariate Normal Distribution and the Spatial Linear Model
A.12.3 Change of Variables
A.12.4 Profile Log‐likelihood
A.12.5 Confidence Intervals
A.12.6 Linked Parameterization
A.12.7 Model Choice
A.13 Bias in Maximum Likelihood Estimation. A.13.1 A General Result
A.13.2 The Spatial Linear Model
Appendix B A Brief History of the Spatial Linear Model and the Gaussian Process Approach
B.1 Introduction
B.2 Matheron and Watson
B.3 Geostatistics at Leeds 1977–1987. B.3.1 Courses, Publications, Early Dissemination
B.3.2 Numerical Problems with Maximum Likelihood
B.4 Frequentist vs. Bayesian Inference
References and Author Index
Index. a
b
c
d
e
f
g
h
i
k
l
m
n
o
p
q
r
s
t
u
v
w
WILEY END USER LICENSE AGREEMENT
Отрывок из книги
WILEY SERIES IN PROBABILITY AND STATISTICS
Established by Walter A. Shewhart and Samuel S. Wilks
.....
Figure 5.1 Bauxite data: Bubble plot and directional semivariograms.
Figure 5.2 Elevation data: Bubble plot and directional semivariograms.
.....