Surface Displacement Measurement from Remote Sensing Images
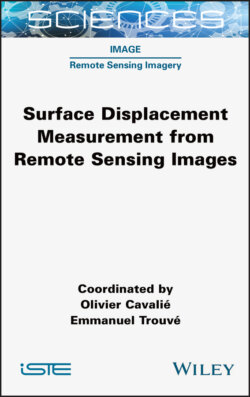
Реклама. ООО «ЛитРес», ИНН: 7719571260.
Оглавление
Olivier Cavalie. Surface Displacement Measurement from Remote Sensing Images
Table of Contents
List of Tables
List of Illustrations
Guide
Pages
Surface Displacement Measurement from Remote Sensing Images
Preface
1. Relevant Past, On-going and Future Space Missions
1.1. Some key parameters for space missions. 1.1.1. Parameters for both SAR and optical missions
1.1.2. Parameters specific to SAR missions
1.1.3. Parameters specific to optical missions
1.2. Past and on-going SAR missions
1.2.1. ERS-1, ERS-2 and Envisat
1.2.2. Canadian C-band satellites: Radarsat-1, Radarsat-2 and RCM
1.2.3. Japanese L-band satellites: JERS-1, ALOS and ALOS-2
1.2.4. SRTM and X-SAR
1.2.5. TerraSAR-X, TanDEM-X and PAZ
1.2.6. COSMO SkyMed constellations
1.2.7. SAOCOM1
1.2.8. Sentinel-1
1.2.9. ICEYE
1.3. Future SAR missions
1.3.1. TerraSAR-NG
1.3.2. ALOS-4
1.3.3. NISAR
1.3.4. Biomass
1.3.5. ROSE-L
1.4. Optical imaging missions. 1.4.1. Past optical missions
1.4.2. On-going optical missions
1.4.3. Future optical missions
1.5. Conclusion
1.6. Acknowledgments
1.7. References
2. Image Matching and Optical Sensors
2.1. Introduction, definition and applications
2.1.1. Problem definition
2.1.2. Geometry of matching
2.1.3. Radiometric and geometric hypothesis
2.2. Template matching. 2.2.1. Matching as a similarity measure
2.2.2. Basic template approach
2.2.3. Normalized template approach
2.2.3.1. Rank normalization
2.2.3.2. Normalized cross-correlation
2.2.3.3. Census coefficient
2.2.3.4. Other similarity measures
2.3. Handling “large” deformations. 2.3.1. Introduction
2.3.2. A posteriori filtering and regularization approach
2.3.3. Multi-resolution approach
2.4. Discrete nature of the image and sub-pixel matching
2.5. Optical imaging sensors
2.5.1. Sensor geometries
2.5.2. Sensor orientation in 3D space. 2.5.2.1. Physical model
2.5.2.2. Empirical model – rational polynomial coefficients
2.5.2.3. Epipolar model
2.5.2.4. Unmodeled sensor errors
2.6. Acknowledgments
2.7. References
3. SAR Offset Tracking
3.1. Basics of SAR imaging. 3.1.1. Imaging geometry and resolution of SAR systems
3.1.2. Radar speckle and speckle reduction with multi-looking
3.1.3. Spectral support of the backscatter intensity
3.1.4. Offsets due to radar penetration
3.2. SAR offset tracking
3.2.1. Cross-correlation. 3.2.1.1. Cross-correlation in the spatial domain
3.2.1.2. Confidence measure of offset estimation
3.2.1.3. Cross-correlation in the frequency domain
3.2.1.4. Sub-pixel estimation in the frequency domain
3.2.2. SAR offset tracking using image pairs
3.2.2.1. Coherence tracking
3.2.2.2. Speckle tracking
3.2.2.3. Intensity tracking
3.2.2.4. Filters for image pre- and post-processing
3.2.3. SAR offset tracking using image series
3.2.3.1. Cross-correlation stacking
3.2.3.2. Autofocusing motion blur in image stacks
3.2.4. Tracking from single and multiple orbits
3.2.4.1. Single-orbit tracking in slant-range coordinates
3.2.4.2. Tracking in map coordinates
3.2.4.3. Three-dimensional inversion
3.2.5. Summary
3.3. Acknowledgments
3.4. References
4. SAR Interferometry: Principles and Processing
4.1. Introduction
4.2. Principle and limits of SAR interferometry
4.2.1. Geometrical information
4.2.2. How to choose an interferometric pair?
4.2.3. Phase and coherence estimation
4.2.4. Loss of coherency: several reasons
4.2.5. Other InSAR limitations
4.3. Atmospheric corrections. 4.3.1. Compensation for tropospheric delays
4.3.2. Estimation of and compensation for ionospheric propagation delays. 4.3.2.1. Introduction
4.3.2.2. Ionosphere estimation and compensation
4.4. InSAR processing chains
4.4.1. Main steps in InSAR processing
4.4.1.1. Coregistration
4.4.1.2. Coherence and interferogram computation
4.4.1.3. Topographic fringe removal
4.4.1.4. Geocoding and mosaicking
4.4.1.5. Filtering and unwrapping
4.4.2. Illustration of the DInSAR processing chain: a case study with DiapOTB
4.4.2.1. Detailed description of steps in the DiapOTB chain
4.4.2.2. Example of processing for the Le Teil earthquake
4.4.3. Available InSAR software: a quick overview
4.5. Conclusion
4.6. Acknowledgments
4.7. References
5. Advanced Methods for Time-series InSAR
5.1. Introduction
5.2. Background of time-series InSAR analysis
5.3. A review. 5.3.1. Small baseline methods for time-series analysis
5.3.2. From PS to PSDS
5.4. The SBAS technique. 5.4.1. Principle and definition of a “small baseline” network of interferograms
5.4.2. Multi-looking and filtering, atmospheric or DEM error corrections and unwrapping
5.4.3. Reconstruction of the time series
5.4.4. Source separation, atmosphere versus deformation
5.5. The PSDS technique. 5.5.1. The PS algorithm
5.5.2. PS selection
5.5.3. DS selection
5.5.4. Phase linking
5.5.5. Tools supporting PS and PSDS processing
Author contributions
5.6. Acknowledgments
5.7. References
6. The Interferometric Phase: Unwrapping and Closure Phase
6.1. Introduction
6.2. Phase unwrapping algorithms and limitation of phase unwrapping errors. 6.2.1. The problem of phase unwrapping and some prerequisites. 6.2.1.1. What is phase unwrapping?
6.2.1.2. Wrapped and unwrapped phase gradients
6.2.1.3. Notions of the rotational, residue and congruency
6.2.1.4. Other useful prerequisites
6.2.2. A short history of unwrapping methods in InSAR
6.2.3. Residue-cut tree algorithm, variants and 3D generalization
6.2.4. Least-squares method
6.2.5. Network-based approaches. 6.2.5.1. The MCF algorithm and its 3D generalization
6.2.5.2. Redundant finite difference integration and phase unwrapping algorithm
6.2.5.3. Stepwise 3D algorithm and its adaptation in StaMPS software
6.2.5.4. SNAPHU
6.2.5.5. Edgelist phase unwrapping
6.2.6. Methods for correcting unwrapping errors
6.2.6.1. Bridging scheme applicable to single interferograms
6.2.6.2. Error correction applied to InSAR time series
6.2.7. Summary and comparison
6.3. The (re)discovery of closure phases and their implications for SAR interferometry
6.3.1. Introduction to closure phases
6.3.2. Mathematical properties of closure phases
6.3.3. Physical misclosures
6.3.3.1. A volumetric scatterer observed at different angles
6.3.3.2. A simple interferometric model for soil moisture
6.3.3.3. Other reasons for differential motion in a resolution cell
6.3.4. Implications for interferometric phase estimation. 6.3.4.1. Statistical misclosure and phase triangulation algorithms
6.3.4.2. Biases everywhere
6.3.4.3. Outlook: multi-frequency and polarimetry
6.4. Acknowledgments
6.5. References
7. Remote Sensing of the Earthquake Deformation Cycle
7.1. Introduction
7.2. What have we learned about faults from nearly three decades of tectonic InSAR?
7.2.1. Coseismic deformation. 7.2.1.1. History and recent progress in coseismic InSAR studies
7.2.1.2. What have we learned about faults from InSAR studies of coseismic deformation
7.2.2. Inter-seismic deformation. 7.2.2.1. History and recent progress in inter-seismic InSAR studies
7.2.2.2. What have we learned about faults from InSAR studies of inter-seismic deformation
7.2.3. Post-seismic deformation and aseismic deformation transients. 7.2.3.1. History and recent progress in the study of aseismic deformation transients
7.2.3.2. What have we learned about faults from InSAR studies of aseismic deformation transients
7.3. Investigating earthquake surface ruptures with optical image correlation. 7.3.1. A history of the optical correlation technique in the study of earthquakes
7.3.2. Measuring earthquake surface displacements from optical images: methodology
7.3.2.1. Optical image correlation: general workflow
7.3.2.2. Measuring the 3D displacement field of earthquakes from stereo optical images
7.3.2.3. Limitations and sources of error
7.3.2.4. Perspectives and future developments
7.3.3. Some examples of near-fault displacement fields from recent and historical earthquakes
7.3.3.1. Horizontal surface displacement of the 1992 Mw 7.3 Landers earthquake from high-resolution aerial images
7.3.3.2. Three-dimensional surface displacement of the 2016 Mw 7.8 Kaikoura earthquake from Worldview satellite images
7.3.3.3. Horizontal surface displacement field of the 1979 Mw 7.1 Khuli-Boniabad, Iran, earthquake from KH-9 spy satellite images
7.3.4. New insights from high-resolution near-fault displacement maps
7.3.4.1. Characterization of off-fault deformation
7.3.4.2. Improvement of fault slip model
7.3.4.3. Impact of the fault structural properties on the coseismic slip distribution
7.4. Conclusion
7.5. Acknowledgments
7.6. References
8. Volcanology: The Crucial Contribution of Surface Displacement Measurements from Space for Understanding and Monitoring Volcanoes
8.1. Introduction
8.2. Origin of surface displacement and topographic changes in a volcano
8.2.1. Typical deformations in a basaltic system
8.2.2. Typical deformations in an andesitic stratovolcano
8.3. Techniques used to measure displacements and topographic changes in volcanoes
8.3.1. Pixel offset tracking
8.3.2. Measurement by interferometry (phase difference)
8.3.3. Measurement of topographic changes from differences in digital elevation models
8.3.3.1. Building a digital elevation model
8.3.3.2. Differential DEM
8.4. Main limitations of measurements obtained by remote sensing. 8.4.1. Temporal resolution
8.4.2. Cloud cover (optical imagery)
8.4.3. Atmospheric artifacts (radar imagery)
8.5. Main contributions of spatial geodesy for monitoring and studying volcanoes
8.5.1. The development of global and statistical studies
8.5.2. Improving knowledge of the magma supply system
8.5.3. Growth and stability of volcanic edifices
8.6. Recent progress. 8.6.1. Integrating satellite imagery with field data
8.6.2. Automating processing chains for real-time detection
8.6.3. Integration of all satellite sources in multi-parameter studies
8.7. Volcanic crisis management: the contribution of displacement measurements obtained using spatial imagery
8.7.1. Piton de la Fournaise, Réunion: benefits of spatial imagery for a highly active, closely monitored volcano
8.7.2. Mount Agung, Indonesia: using InSAR data in real time in a crisis management situation
8.7.3. Taal, Philippines: innovative use of InSAR information in real time
8.8. Conclusion
8.9. Acknowledgments
8.10. References
9. Anthropogenic Activity: Monitoring Surface-Motion Consequences of Human Activities with Spaceborne InSAR
9.1. Introduction
9.2. Characteristics of subsidence/uplift phenomena associated with human activity. 9.2.1. Underground activities
9.2.2. Cavities
9.2.3. Suitability of the interferometric SAR techniques
9.2.4. Optimum SAR data properties for monitoring anthropogenic activities
9.2.4.1. Satellite repeat cycle
9.2.4.2. Spatial resolution
9.2.4.3. Archive continuity
9.2.4.4. Radar wavelength
9.3. Application examples: urban underground activities. 9.3.1. Urban underground construction works
9.3.2. Urban groundwater extraction
9.3.2.1. Mexico case study
9.3.2.2. Hanoi case study
9.4. Applications in mineral resources extractive activities. 9.4.1. Brine, oil and gas extraction/injection. 9.4.1.1. Brine extraction
9.4.1.2. GAS injection/extraction
9.4.1.3. Oil extraction
9.4.2. Mining activities
9.5. Conclusion
9.6. Acknowledgments
9.7. References
10. Measuring Kinematics of Slow-Moving Landslides from Satellite Images
10.1. Introduction
10.2. Image correlation applied to satellite optical images
10.2.1. Landslide detection with optical remote sensing
10.2.2. Landslide characterization with optical remote sensing
10.2.3. Landslide monitoring with optical remote sensing
10.3. Offset tracking of SAR images applied to landslides
10.4. InSAR for landslide studies
10.4.1. Standard versus multi-temporal InSAR analyses
10.4.2. Limitations in the use of SAR interferometry for landslide applications
10.4.3. Landslide detection with InSAR
10.4.4. Landslide characterization with InSAR
10.4.5. Landslide monitoring with InSAR
10.5. Conclusion
10.6. References
11. Remote Sensing of Glacier Motion
11.1. Introduction
11.2. What is glacier motion? 11.2.1. Physical basis of ice flow
11.2.2. Scales and processes of displacement in glaciology
11.3. Measuring glacier displacement from satellite data
11.3.1. Image preprocessing
11.3.1.1. Spectral band selection
11.3.1.2. High-pass filtering
11.3.1.3. Image striping
11.3.1.3.1. Missing data
11.3.2. Offset-tracking algorithms
11.3.3. InSAR
11.3.3.1. Temporal decorrelation
11.3.3.2. Phase unwrapping
11.3.3.3. Reprojection of the line-of-sight displacement vector
11.3.3.4. Sources of uncertainty
11.3.4. Filtering and corrections of displacement fields. 11.3.4.1. Filtering
11.3.4.2. Coregistration, terrain and sensor corrections
11.3.4.3. Comparison with ancillary observations and validation
11.3.5. Working with time series
11.3.5.1. Average flow (degree 0)
11.3.5.2. Pluri-annual trends (degree 1)
11.3.5.3. Sub-annual fluctuations (higher degree)
11.3.6. Summary of benefits and drawbacks of the different methods
11.4. What can we learn from glacier displacement?
11.4.1. From a topological perspective
11.4.2. From a climatological perspective
11.4.3. From a mechanical perspective
11.4.4. From a hydrological perspective
11.4.5. From a geomorphological perspective
11.4.6. From a hazards perspective
11.5. Perspectives and future directions. 11.5.1. What will we learn from future missions?
11.5.2. What future sensors are needed for glaciology?
11.6. Acknowledgments
11.7. References
12. New Applications of Spaceborne Optical Image Cross-Correlation: Digital Elevation Models of Volcanic Clouds and Shallow Bathymetry from Space
12.1. Introduction. 12.1.1. General introduction
12.2. Digital elevation models of volcanic ash clouds. 12.2.1. Introduction: can we precisely measure the height of a volcanic ash cloud and what physical process controls the injection height and the speed of a volcanic ash plume?
12.2.2. Principles
12.2.3. Applications
12.2.3.1. Holuhraun (Landsat 8)
12.2.3.2. Ubinas 2019 (Pléiades)
12.2.3.3. Etna (SPOT-1)
12.3. Shallow bathymetry: measuring wave characteristics from space. 12.3.1. Introduction: can we map tectonic fault motion under shallow water and can we measure differential bathymetry?
12.3.2. Principles
12.3.3. Applications
12.4. Concluding remarks
12.5. Acknowledgments
12.6. References
List of Authors
Index
WILEY END USER LICENSE AGREEMENT
Отрывок из книги
Image, Field Director – Laure Blanc-Feraud
.....
In April 2014, ALOS-2 followed the ALOS program with an L-band radar payload, without the optical part later put on ALOS-3. Compared to ALOS, the ALOS-2 PALSAR instrument improved the resolution (tunable between 14 and 84 MHz), the revisit cycle (14 days instead of 46 for ALOS) and right and left imaging capacities and provided new modes, such as spotlight and ScanSAR with burst synchronization for ScanSAR interferometry.
Data access: We can note that the background mission of these satellites was orchestrated by a basic operational scenario, i.e. a long-term homogeneous and consistent programming of the whole Earth in different modes, with regular updates. It is possible to freely access JERS-1 and ALOS data through the ESA EO portal at https://earth.esa.int/web/guest/-/jers-1-sar-level-1-single-look-complex-image and https://earth.esa.int/web/guest/-/alos-palsar-fbs-fbd-and-plr-products, respectively. The datasets contain all ESA acquisitions over the ADEN zone (Europe, Africa and the Middle East), plus some worldwide products received from JAXA.
.....