Artificial Intelligence Hardware Design
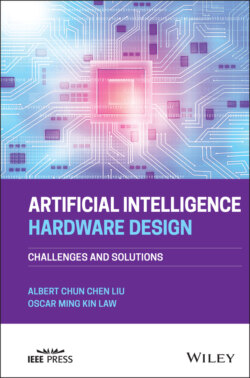
Реклама. ООО «ЛитРес», ИНН: 7719571260.
Оглавление
Albert Chun-Chen Liu. Artificial Intelligence Hardware Design
Table of Contents
List of Tables
List of Illustrations
Guide
Pages
Artificial Intelligence Hardware Design. Challenges and Solutions
Author Biographies
Preface
Acknowledgments
Table of Figures
1 Introduction
1.1 Development History
1.2 Neural Network Models
1.3 Neural Network Classification
1.3.1 Supervised Learning
1.3.2 Semi‐supervised Learning
1.3.3 Unsupervised Learning
1.4 Neural Network Framework
1.5 Neural Network Comparison
Exercise
References
Notes
2 Deep Learning
2.1 Neural Network Layer
2.1.1 Convolutional Layer
2.1.2 Activation Layer
2.1.3 Pooling Layer
2.1.4 Normalization Layer
2.1.5 Dropout Layer
2.1.6 Fully Connected Layer
2.2 Deep Learning Challenges
Exercise
References
Notes
3 Parallel Architecture
3.1 Intel Central Processing Unit (CPU)
3.1.1 Skylake Mesh Architecture
3.1.2 Intel Ultra Path Interconnect (UPI)
3.1.3 Sub Non‐unified Memory Access Clustering (SNC)
3.1.4 Cache Hierarchy Changes
3.1.5 Single/Multiple Socket Parallel Processing
3.1.6 Advanced Vector Software Extension
3.1.7 Math Kernel Library for Deep Neural Network (MKL‐DNN)
3.2 NVIDIA Graphics Processing Unit (GPU)
3.2.1 Tensor Core Architecture
3.2.2 Winograd Transform
3.2.3 Simultaneous Multithreading (SMT)
3.2.4 High Bandwidth Memory (HBM2)
3.2.5 NVLink2 Configuration
3.3 NVIDIA Deep Learning Accelerator (NVDLA)
3.3.1 Convolution Operation
3.3.2 Single Data Point Operation
3.3.3 Planar Data Operation
3.3.4 Multiplane Operation
3.3.5 Data Memory and Reshape Operations
3.3.6 System Configuration
3.3.7 External Interface
3.3.8 Software Design
3.4 Google Tensor Processing Unit (TPU)
3.4.1 System Architecture
3.4.2 Multiply–Accumulate (MAC) Systolic Array
3.4.3 New Brain Floating‐Point Format
3.4.4 Performance Comparison
3.4.5 Cloud TPU Configuration
3.4.6 Cloud Software Architecture
3.5 Microsoft Catapult Fabric Accelerator
3.5.1 System Configuration
3.5.2 Catapult Fabric Architecture
3.5.3 Matrix‐Vector Multiplier
3.5.4 Hierarchical Decode and Dispatch (HDD)
3.5.5 Sparse Matrix‐Vector Multiplication
Exercise
References
Notes
4 Streaming Graph Theory
4.1 Blaize Graph Streaming Processor. 4.1.1 Stream Graph Model
4.1.2 Depth First Scheduling Approach
4.1.3 Graph Streaming Processor Architecture
4.2 Graphcore Intelligence Processing Unit
4.2.1 Intelligence Processor Unit Architecture
4.2.2 Accumulating Matrix Product (AMP) Unit
4.2.3 Memory Architecture
4.2.4 Interconnect Architecture
4.2.5 Bulk Synchronous Parallel Model
Exercise
References
5 Convolution Optimization
5.1 Deep Convolutional Neural Network Accelerator
5.1.1 System Architecture
5.1.2 Filter Decomposition
5.1.3 Streaming Architecture
5.1.3.1 Filter Weights Reuse
5.1.3.2 Input Channel Reuse
5.1.4 Pooling
5.1.4.1 Average Pooling
5.1.4.2 Max Pooling
5.1.5 Convolution Unit (CU) Engine
5.1.6 Accumulation (ACCU) Buffer
5.1.7 Model Compression
5.1.8 System Performance
5.2 Eyeriss Accelerator
5.2.1 Eyeriss System Architecture
5.2.2 2D Convolution to 1D Multiplication
5.2.3 Stationary Dataflow
5.2.3.1 Output Stationary
5.2.3.2 Weight Stationary
5.2.3.3 Input Stationary
5.2.4 Row Stationary (RS) Dataflow
5.2.4.1 Filter Reuse
5.2.4.2 Input Feature Maps Reuse
5.2.4.3 Partial Sums Reuse
5.2.5 Run‐Length Compression (RLC)
5.2.6 Global Buffer
5.2.7 Processing Element Architecture
5.2.8 Network‐on‐Chip (NoC)
5.2.9 Eyeriss v2 System Architecture
5.2.10 Hierarchical Mesh Network
5.2.10.1 Input Activation HM‐NoC
5.2.10.2 Filter Weight HM‐NoC
5.2.10.3 Partial Sum HM‐NoC
5.2.11 Compressed Sparse Column Format
5.2.12 Row Stationary Plus (RS+) Dataflow
5.2.13 System Performance
Exercise
References
Notes
6 In‐Memory Computation
6.1 Neurocube Architecture
6.1.1 Hybrid Memory Cube (HMC)
6.1.2 Memory Centric Neural Computing (MCNC)
6.1.3 Programmable Neurosequence Generator (PNG)
6.1.4 System Performance
6.2 Tetris Accelerator
6.2.1 Memory Hierarchy
6.2.2 In‐Memory Accumulation
6.2.3 Data Scheduling
6.2.4 Neural Network Vaults Partition
6.2.5 System Performance
6.3 NeuroStream Accelerator. 6.3.1 System Architecture
6.3.2 NeuroStream Coprocessor
6.3.3 4D Tiling Mechanism
6.3.4 System Performance
Exercise
References
7 Near‐Memory Architecture. 7.1 DaDianNao Supercomputer
7.1.1 Memory Configuration
7.1.2 Neural Functional Unit (NFU)
7.1.3 System Performance
7.2 Cnvlutin Accelerator
7.2.1 Basic Operation
7.2.2 System Architecture
7.2.3 Processing Order
7.2.4 Zero‐Free Neuron Array Format (ZFNAf)
7.2.5 The Dispatcher
7.2.6 Network Pruning
7.2.7 System Performance
7.2.8 Raw or Encoded Format (RoE)
7.2.9 Vector Ineffectual Activation Identifier Format (VIAI)
7.2.10 Ineffectual Activation Skipping
7.2.11 Ineffectual Weight Skipping
Exercise
References
Notes
8 Network Sparsity
8.1 Energy Efficient Inference Engine (EIE)
8.1.1 Leading Nonzero Detection (LNZD) Network
8.1.2 Central Control Unit (CCU)
8.1.3 Processing Element (PE)
8.1.4 Deep Compression
8.1.5 Sparse Matrix Computation
8.1.6 System Performance
8.2 Cambricon‐X Accelerator
8.2.1 Computation Unit
8.2.2 Buffer Controller
8.2.3 System Performance
8.3 SCNN Accelerator
8.3.1 SCNN PT‐IS‐CP‐Dense Dataflow
8.3.2 SCNN PT‐IS‐CP‐Sparse Dataflow
8.3.3 SCNN Tiled Architecture
8.3.4 Processing Element Architecture
8.3.5 Data Compression
8.3.6 System Performance
8.4 SeerNet Accelerator
8.4.1 Low‐Bit Quantization
8.4.2 Efficient Quantization
8.4.3 Quantized Convolution
8.4.4 Inference Acceleration
8.4.5 Sparsity‐Mask Encoding
8.4.6 System Performance
Exercise
References
Notes
9 3D Neural Processing
9.1 3D Integrated Circuit Architecture
9.2 Power Distribution Network
9.3 3D Network Bridge
9.3.1 3D Network‐on‐Chip
9.3.2 Multiple‐Channel High‐Speed Link
9.4 Power‐Saving Techniques. 9.4.1 Power Gating
9.4.2 Clock Gating
Exercise
References
Note
Appendix A Neural Network Topology
Note
Index. a
b
c
d
e
f
g
h
i
l
m
n
p
r
s
t
u
v
w
x
z
WILEY END USER LICENSE AGREEMENT
Отрывок из книги
IEEE Press 445 Hoes Lane Piscataway, NJ 08854
.....
Chapter 5 shows how to optimize convolution with the University of California, Los Angeles (UCLA) Deep Convolutional Neural Network (DCNN) accelerator filter decomposition and Massachusetts Institute of Technology (MIT) Eyeriss accelerator Row Stationary dataflow.
Chapter 6 illustrates in‐memory computation through Georgia Institute of Technologies Neurocube and Stanford Tetris accelerator using Hybrid Memory Cube (HMC) as well as University of Bologna Neurostream accelerator using Smart Memory Cubes (SMC).
.....