Pedestrian Inertial Navigation with Self-Contained Aiding
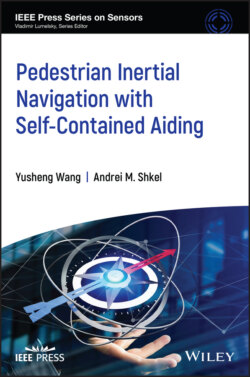
Реклама. ООО «ЛитРес», ИНН: 7719571260.
Оглавление
Andrei M. Shkel. Pedestrian Inertial Navigation with Self-Contained Aiding
Table of Contents
List of Tables
List of Illustrations
Guide
Pages
Pedestrian Inertial Navigation with Self‐Contained Aiding
Author Biographies
List of Figures
List of Tables
1 Introduction. 1.1 Navigation
1.2 Inertial Navigation
1.3 Pedestrian Inertial Navigation
1.3.1 Approaches
1.3.2 IMU Mounting Positions
1.3.3 Summary
1.4 Aiding Techniques for Inertial Navigation
1.4.1 Non‐self‐contained Aiding Techniques
1.4.1.1 Aiding Techniques Based on Natural Signals
1.4.1.2 Aiding Techniques Based on Artificial Signals
1.4.2 Self‐contained Aiding Techniques
1.5 Outline of the Book
References
2 Inertial Sensors and Inertial Measurement Units
2.1 Accelerometers
2.1.1 Static Accelerometers
2.1.2 Resonant Accelerometers
2.2 Gyroscopes
2.2.1 Mechanical Gyroscopes
2.2.2 Optical Gyroscopes
2.2.2.1 Ring Laser Gyroscopes
2.2.2.2 Fiber Optic Gyroscopes
2.2.3 Nuclear Magnetic Resonance Gyroscopes
2.2.4 MEMS Vibratory Gyroscopes
2.2.4.1 Principle of Operation
2.2.4.2 Mode of Operation
Open‐Loop Mode
Force‐to‐Rebalance Mode
Whole Angle Mode
2.2.4.3 Error Analysis
2.3 Inertial Measurement Units
2.3.1 Multi‐sensor Assembly Approach
2.3.2 Single‐Chip Approach
2.3.3 Device Folding Approach
2.3.4 Chip‐Stacking Approach
2.4 Conclusions
References
3 Strapdown Inertial Navigation Mechanism
3.1 Reference Frame
3.2 Navigation Mechanism in the Inertial Frame
3.3 Navigation Mechanism in the Navigation Frame
3.4 Initialization
3.4.1 Tilt Sensing
3.4.2 Gyrocompassing
3.4.3 Magnetic Heading Estimation
3.5 Conclusions
References
4 Navigation Error Analysis in Strapdown Inertial Navigation
4.1 Error Source Analysis
4.1.1 Inertial Sensor Errors
4.1.2 Assembly Errors
4.1.3 Definition of IMU Grades
4.1.3.1 Consumer Grade
4.1.3.2 Industrial Grade
4.1.3.3 Tactical Grade
4.1.3.4 Navigation Grade
4.2 IMU Error Reduction. 4.2.1 Six‐Position Calibration
4.2.2 Multi‐position Calibration
4.3 Error Accumulation Analysis
4.3.1 Error Propagation in Two‐Dimensional Navigation
4.3.2 Error Propagation in Navigation Frame
4.4 Conclusions
References
5 Zero‐Velocity Update Aided Pedestrian Inertial Navigation
5.1 Zero‐Velocity Update Overview
5.2 Zero‐Velocity Update Algorithm. 5.2.1 Extended Kalman Filter
5.2.2 EKF in Pedestrian Inertial Navigation
5.2.3 Zero‐Velocity Update Implementation
5.3 Parameter Selection
5.4 Conclusions
References
6 Navigation Error Analysis in the ZUPT‐Aided Pedestrian Inertial Navigation
6.1 Human Gait Biomechanical Model
6.1.1 Foot Motion in Torso Frame
6.1.2 Foot Motion in Navigation Frame
6.1.3 Parameterization of Trajectory
6.2 Navigation Error Analysis
6.2.1 Starting Point
6.2.2 Covariance Increase During Swing Phase
6.2.3 Covariance Decrease During the Stance Phase
6.2.4 Covariance Level Estimation
6.2.5 Observations
6.3 Verification of Analysis
6.3.1 Numerical Verification
6.3.1.1 Effect of ARW
6.3.1.2 Effect of VRW
6.3.1.3 Effect of RRW
6.3.2 Experimental Verification
6.4 Limitations of the ZUPT Aiding Technique
6.5 Conclusions
References
7 Navigation Error Reduction in the ZUPT‐Aided Pedestrian Inertial Navigation
7.1 IMU‐Mounting Position Selection
7.1.1 Data Collection
7.1.2 Data Averaging
7.1.3 Data Processing Summary
7.1.4 Experimental Verification
7.2 Residual Velocity Calibration
7.3 Gyroscope G‐Sensitivity Calibration
7.4 Navigation Error Compensation Results
7.5 Conclusions
References
8 Adaptive ZUPT‐Aided Pedestrian Inertial Navigation
8.1 Floor Type Detection
8.1.1 Algorithm Overview
8.1.2 Algorithm Implementation
8.1.2.1 Data Partition
8.1.2.2 Principal Component Analysis
8.1.2.3 Artificial Neural Network
8.1.2.4 Multiple Model EKF
8.1.3 Navigation Result
8.1.4 Summary
8.2 Adaptive Stance Phase Detection
8.2.1 Zero‐Velocity Detector
8.2.2 Adaptive Threshold Determination
8.2.3 Experimental Verification
8.2.4 Summary
8.3 Conclusions
References
9 Sensor Fusion Approaches
9.1 Magnetometry
9.2 Altimetry
9.3 Computer Vision
9.4 Multiple‐IMU Approach
9.5 Ranging Techniques
9.5.1 Introduction to Ranging Techniques
9.5.1.1 Time of Arrival
9.5.1.2 Received Signal Strength
9.5.1.3 Angle of Arrival
9.5.2 Ultrasonic Ranging
9.5.2.1 Foot‐to‐Foot Ranging
9.5.2.2 Directional Ranging
9.5.3 Ultrawide Band Ranging
9.6 Conclusions
References
10 Perspective on Pedestrian Inertial Navigation Systems
10.1 Hardware Development
10.2 Software Development
10.3 Conclusions
References
Index. a
b
c
d
e
f
g
h
i
k
l
m
n
o
p
q
r
s
t
u
v
w
y
z
IEEE Press Series on Sensors
WILEY END USER LICENSE AGREEMENT
Отрывок из книги
Yusheng Wang and Andrei M. Shkel
University of California, Irvine
.....
There are two general approaches in the pedestrian inertial navigation. One is the strapdown inertial navigation as introduced in Section 1.2, where IMU readouts are integrated into position and orientation. This approach is universally applicable, but the integral step makes the algorithm computationally expensive and the navigation error accumulates as time cubed due to the gyroscope bias. In order to limit the error propagation, the most commonly used method is to apply the Zero‐Velocity Updates (ZUPTs) when the velocity of the foot is close to zero (the foot is stationary on the ground) [12]. The stationary state can be used to limit the long‐term velocity and angular rate drift, thus greatly reduce the navigation error. In this implementation, IMU is fixed on the foot to perform the navigation and to detect the stance phase at the same time. Whenever the stance phase is detected, the zero‐velocity information of the foot is fed into the Extended Kalman Filter (EKF) as a pseudo‐measurement to compensate for IMU biases, thus reducing the navigation error growth in the system. In this architecture, not only the navigation errors but also IMU errors can be estimated by the EKF. The limitation of this approach is that the IMU needs to be mounted on the foot.
In order to avoid the integral step in the pedestrian inertial navigation and also relax the requirement of IMU mounting position, a Step‐and‐Heading System (SHS) is an alternative. It is composed of three main parts: step detection, step length estimation, and step heading angle estimation [13]. Unlike the first approach, this approach can only be applied in the pedestrian inertial navigation. In this approach, the step length of each stride is first estimated based on some features of motion obtained from the IMU readouts. Methods based on biomechanical models and statistical regression methods are popular for the estimation. Some commonly used features include the gait frequency, magnitude of angular rate, vertical acceleration, and variance of angular rate. Then, the heading angle is estimated by the gyroscope readout, which is typically mounted at the head. This step can also be aided by magnetometers to improve the accuracy. In this way, the total displacement can be estimated combining the traveled distance and the heading angle. However, two major challenges exist for this approach. First, the gazing direction needs to be aligned with the traveling direction, implying that the subject needs to look at the traveling direction all the time, which is not practical. Second, the step length estimation remains difficult. The average value of the estimated step length may be accurate when median value generally less than 2%, but the estimate precision is generally low, with the Root Mean Square Error (RMSE) about 5% [14]. With a wide adaption of hand‐held and fitness devices, this is currently an active area of research.
.....