End-to-end Data Analytics for Product Development
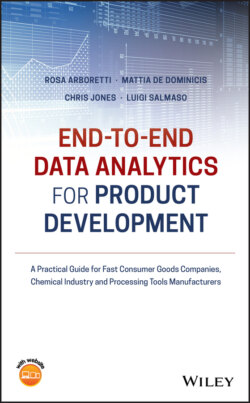
Реклама. ООО «ЛитРес», ИНН: 7719571260.
Оглавление
Chris Jones. End-to-end Data Analytics for Product Development
Table of Contents
List of Tables
List of Illustrations
Guide
Pages
End‐to‐End Data Analytics for Product Development. A Practical Guide for Fast Consumer Goods Companies, Chemical Industry and Processing Tools Manufacturers
Biographies
Preface
About the Companion Website
1 Basic Statistical Background. 1.1 Introduction
Learning Objectives and Outcomes
Stat Tool 1.1 Statistical Variables and Types of Data
Stat Tool 1.2 Statistical Unit, Population, Sample
Stat Tool 1.3 Descriptive and Inferential Analysis
Stat Tool 1.4 Shapes of Data Distributions
Stat Tool 1.5 Shapes of Data Distributions for Quantitative Variables
Stat Tool 1.6 Measures of Central Tendency: Mean and Median
Stat Tool 1.7 Measures of Non‐Central Tendency: Quartiles
Stat Tool 1.8 Measures of Variability: Range and Interquartile Range
Stat Tool 1.9 Measures of Variability: Variance and Standard Deviation
Stat Tool 1.10 Measures of Variability: Coefficient of Variation
Stat Tool 1.11 Boxplots
Stat Tool 1.12 Basic Concepts of Statistical Inference
Stat Tool 1.13 Inferential Problems
Stat Tool 1.14 Estimation of Population Parameters and Confidence Intervals
Stat Tool 1.15 Hypothesis Testing
Stat Tool 1.16 The p‐Value
2 The Screening Phase. 2.1 Introduction
Learning Objectives and Outcomes
2.2 Case Study: Air Freshener Project
2.2.1 Plan of the Screening Experiment
Stat Tool 2.1 Experiments, Factors, Responses
Stat Tool 2.2 DOE, Factorial Designs, Screening Experiments
Stat Tool 2.3 Basic Principles of Factorial Designs: Randomization
Stat Tool 2.4 Basic Principles of Factorial Designs: Blocking
Stat Tool 2.5 Basic Principles of Factorial Designs: Replication
2.2.1.1 Step 1 – Create a Full Factorial Design
2.2.1.2 Step 2 – Alternately, Choose the Desired Fractional Design
2.2.1.3 Step 3 – Assign the Designed Factor Level Combinations to the Experimental Units and Collect Data for the Response Variable
Stat Tool 2.6 Guidelines for Planning and Conducting Experiments
2.2.2 Plan of the Statistical Analyses
2.2.2.1 Step 1 – Perform a Descriptive Analysis of the Response Variable
2.2.2.1.1 Interpret the Results of Step 1
2.2.2.2 Step 2 – Apply the Analysis of Variance to Estimate the Effects and Determine the Significant Ones
Stat Tool 2.7 ANOVA, Analysis Of VAriance
Stat Tool 2.8 Model assumptions for ANOVA
2.2.2.3 Step 3 – If Required, Reduce the Model to Include the Significant Terms
2.2.2.3.1 Interpret the Results of Step 3
Stat Tool 2.9 Residual Analysis
3 Product Development and Optimization. 3.1 Introduction
Learning Objectives and Outcomes
3.2 Case Study for Single Sample Experiments: Throat Care Project
3.2.1 Comparing the Mean to a Specified Value
3.2.1.1 Step 1 – Perform a Descriptive Analysis of the Variable “Ingredient”
3.2.1.2 Step 2 – Assess the Null and the Alternative Hypotheses and Apply the One‐Sample t‐Test
Stat Tool 3.1 One‐Sample t‐Test
3.2.2 Comparing a Proportion to a Specified Value
3.2.2.1 Step 1 – Calculate the Sample Proportion of Lozenges with Total Weight Greater than 2.85 mg
3.2.2.2 Step 2 – Assess the Null and the Alternative Hypotheses and Apply the One Proportion Test
Stat Tool 3.2 One Proportion Test
3.3 Case Study for Two‐Sample Experiments: Condom Project
3.3.1 Comparing Variability Between Two Groups
3.3.1.1 Step 1 – Perform a Descriptive Analysis of the Variable “Open_end” Stratifying by Formulations
3.3.1.2 Step 2 – Assess the Null and the Alternative Hypotheses and Apply the Two Variances Test
Stat Tool 3.3 Two‐Sample Inferential Problems
Stat Tool 3.4 Two Variances Test
3.3.2 Comparing Means Between Two Groups
3.3.2.1 Step 1 – Perform a Descriptive Analysis of the Variable “Open_end” stratifying by Formulations
3.3.2.2 Step 2 – Assess the Null and the Alternative Hypotheses and Apply the Two‐Sample t‐Test
Stat Tool 3.5 Two‐Sample t‐Test
3.3.2.2.1 Interpret the Results of Step 2
3.3.3 Comparing Two Proportions
3.3.3.1 Step 1 – Calculate the Sample Proportions of Condoms with Thickness Less than or Equal to 0.045 mm
3.3.3.2 Step 2 – Assess the Null and the Alternative Hypotheses and Apply the Two Proportions Test
Stat Tool 3.6 Two Proportions Test
3.4 Case Study for Paired Data: Fragrance Project
3.4.1.1 Step 1 – Descriptive Analysis of “Appropriateness” Stratified by “Fragrance”
3.4.1.2 Step 2 – Descriptive Analysis of “Difference:A_B”
3.4.1.3 Step 3 – Paired t‐Test on Mean Difference
3.4.1.3.1 Interpret the Results of Step 3
Stat Tool 3.7 Paired t‐Test
3.5 Case Study: Stain Removal Project
3.5.1 Plan of the General Factorial Experiment
3.5.1.1 Step 1 – Create a Full Factorial Design
3.5.1.2 Step 2 – Reduce the Full Factorial Design to an Optimal Design
3.5.1.3 Step 3 – Assign the Designed Factor Level Combinations to the Experimental Units and Collect Data for the Response Variable
3.5.2 Plan of the Statistical Analyses
3.5.2.1 Step 1 – Perform a Descriptive Analysis of the Response Variables
3.5.2.2 Step 2 – Fit a Response Surface Model
3.5.2.2.1 Interpret the Results of Step 2
3.5.2.3 Step 3 – If Need Be, Reduce the Model to Include the Significant Terms
3.5.2.4 Step 4 – Optimize the Responses
Stat Tool 3.8 Response Optimization
3.5.2.5 Step 5 – Examine the Shape of the Response Surface and Locate the Optimum
4 Other Topics in Product Development and Optimization: Response Surface and Mixture Designs. 4.1 Introduction
Learning Objectives and Outcomes
4.2 Case Study for Response Surface Designs: Polymer Project
4.2.1 Plan of the Experimental Design
4.2.1.1 Step 1 – Create a CCD
Stat Tool 4.1 The Central Composite Design (CCD)
4.2.1.2 Step 2 – Alternatively, Create a Face‐Centered CCD
4.2.1.3 Step 3 – Alternatively, Create a Box‐Behnken Design
Stat Tool 4.2 The Box‐Behnken Design
4.2.1.4 Step 4 – Assign the Designed Factor Level Combinations to the Experimental Units and Collect Data for the Response Variable
4.2.2 Plan of the Statistical Analyses
4.2.2.1 Step 1 – Perform a Descriptive Analysis of the Response Variables
4.2.2.1.1 Interpret the Results of Step 1
4.2.2.2 Step 2 – Fit a Second‐Order Model to Estimate the Effects and Determine the Significant Ones
4.2.2.2.1 Interpret the Results of Step 2
4.2.2.3 Step 3 – If Need Be, Reduce the Model to Include the Significant Terms
4.2.2.3.1 Interpret the Results of Step 3
4.2.2.4 Step 4 – Optimize the Response
4.2.2.4.1 Interpret the Results of Step 4
4.2.2.5 Step 5 – Examine the Shape of the Response Surface and Locate the Optimum
4.2.2.5.1 Interpret the Results of Step 5
4.3 Case Study for Mixture Designs: Mix‐Up Project
4.3.1 Plan of the Experimental Design
4.3.1.1 Step 1 – Create a Simplex Centroid Design for a Simple Mixture Experiment
Stat Tool 4.3 Mixture Experiments
Stat Tool 4.4 Simplex Centroid Designs
4.3.1.2 Step 2 – Alternatively, Create a Simplex Centroid Design for a Simple Mixture Experiment with Lower Limits for Components
4.3.1.3 Step 3 – Alternatively, Create a Simplex Centroid Design for a Mixture‐Process Variable Experiment
4.3.1.4 Step 4 – Alternatively, Create a Simplex Centroid Design for a Mixture‐Amount Experiment
4.3.1.5 Step 5 – Alternatively, Create a Simplex Lattice Design for a Simple Mixture Experiment
Stat Tool 4.5 Simplex Lattice Designs
4.3.1.6 Step 6 – Alternatively, Create an Extreme Vertices Design with Lower and Upper Limits for Components
Stat Tool 4.6 Constrained Simplex Designs
Stat Tool 4.7 Extreme Vertices Designs
4.3.1.7 Step 7 – Alternatively, Create an Extreme Vertices Design with Linear Constraints for Components
4.3.1.8 Step 8 – Assign the Designed Factor Level Combinations (Design Points) to the Experimental Units and Collect Data for the Response Variable
Stat Tool 4.8 Mixture Models
4.3.2 Plan of the Statistical Analyses
4.3.2.1 Step 1 – Perform a Descriptive Analysis of the Response Variables
4.3.2.1.1 Interpret the Results of Step 1
4.3.2.2 Step 2 – Fit a Second‐Order Model to Estimate the Effects and Determine the Significant Ones
4.3.2.2.1 Interpret the Results of Step 2
4.3.2.3 Step 3 – Optimize the Response
4.3.2.3.1 Interpret the Results of Step 3
4.3.2.4 Step 4 – Examine the Shape of the Response Surface and Locate the Optimum
4.3.2.4.1 Interpret the Results of Step 4
5 Product Validation. 5.1 Introduction
Learning Objectives and Outcomes
5.2 Case Study: GERD Project
5.2.1 Evaluation of the Relationship among Quantitative Variables
5.2.1.1 Step 1 – Perform an Exploratory Analysis through Scatterplots and Calculate the Correlation Coefficients
Stat Tool 5.1 Correlation and Regression Analysis
Stat Tool 5.2 Scatterplot
Stat Tool 5.3 Correlation Coefficient
5.2.1.2 Step 2 – Build a Multiple Linear Regression Model
5.2.1.3 Step 3 – If Required, Reduce the Model to Include the Significant Terms
5.2.1.3.1 Interpret the Results of Step 3
Regression Analysis: Heartburn versus Regurgitation; Dyspepsia
5.2.1.4 Step 4 – Predict Response Values
5.2.1.5 Step 5 – Explore the Response Surface in Multiple Linear Regression
Stat Tool 5.4 Regression Models
Stat Tool 5.5 Simple Linear Regression Models
Stat Tool 5.6 Goodness of Fit
Stat Tool 5.7 Residual Analysis
Stat Tool 5.8 Multiple Linear Regression Models
5.3 Case Study: Shelf Life Project (Fixed Batch Factor)
5.3.1.1 Step 1 – Create a Data Collection Worksheet
5.3.1.2 Step 2 – Apply a Stability Analysis to Estimate the Shelf Life
5.3.1.3 Step 3 – Predict Response Values
5.4 Case Study: Shelf Life Project (Random Batch Factor)
5.4.1.1 Step 1 − Create a Data Collection Worksheet
5.4.1.2 Step 2 – Apply a Stability Analysis to Estimate the Shelf Life
6 Consumer Voice. 6.1 Introduction
Learning Objectives and Outcomes
6.2 Case Study: “Top‐Two Box” Project
6.2.1.1 Step 1 – Perform an Exploratory Descriptive Analysis of Satisfaction Scores by Product, Through Frequency Tables and Charts
Stat Tool 6.1 Cross Tabulations
Stat Tool 6.2 Bar Charts and Pie Charts
6.2.1.2 Step 2 – Apply the χ2 Test to Evaluate the Presence of Association Between Product and Satisfaction Score
Stat Tool 6.3 Chi‐square Test
6.2.1.3 Step 3 – Perform an Exploratory Descriptive Analysis of Variable “Satisfaction” by Temperature and Calculate the Correlation Coefficient
6.2.1.3.1 Interpret the Results of Step 3
Stat Tool 6.4 Spearman Rank Correlation
Stat Tool 6.5 Logistic Regression Models
6.2.1.4 Step 4 – Fit a Binary Logistic Regression Model of Satisfaction Score vs. Temperature and Product
Stat Tool 6.6 Odds Ratios
6.3 Case Study: DOE – Top Score Project
6.3.1 Plan of the Factorial Design
6.3.2 Plan of the Statistical Analyses
6.3.2.1 Step 1 – Perform a Descriptive Analysis of the Binary Response Variable Stratifying by Formulations
6.3.2.2 Step 2 – Fit a Binary Logistic Regression Model
6.3.2.3 Step 3 – If Need be Reduce the Model to Include the Significant Terms and Estimate the Odds Ratios
6.4 Final Remarks
References
Index
WILEY END USER LICENSE AGREEMENT
Отрывок из книги
Rosa Arboretti University of Padova, Italy
Mattia De Dominicis Reckitt Benckiser, Italy
.....
Considering the mean and the standard deviation together and computing the range: mean ± S, we can say that data values vary on average from (mean − S) to (mean + S).
From the previous example the average range is:
.....