Quantifying Human Resources
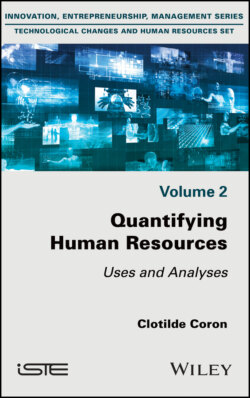
Реклама. ООО «ЛитРес», ИНН: 7719571260.
Оглавление
Clotilde Coron. Quantifying Human Resources
Table of Contents
List of Tables
List of Illustrations
Guide
Pages
Quantifying Human Resources. Uses and Analyses
Acknowledgments
Introduction
I.1. The omnipresence of quantification in Western societies
I.2. The specific challenges of human resources quantification: quantifying the human being
Box I.1.Two incarnations of the psychotechnical approach: the IQ test and the theory of the g factor (sources: Gould 1997; Huteau and Lautrey 2006)
I.3. HR quantification: effective solution or myth? Two lines of research
Box I.2.Quantification as a source of improvement in the definition of HR policies (source: Rasmussen and Ulrich 2015)
Box I.3.Example of the introduction of activity-based pricing in French hospitals (source: Juven 2016)
I.4. The positioning of this work
I.5. Structure of the book
1. From the Statisticalization of Labor to Human Resources Algorithms: The Different Uses of Quantification
1.1. Quantifying reality: quantifying individuals or positions
1.1.1. The statisticalization of individuals and work
1.1.1.1. Different tools for the quantified assessment of individuals
Box 1.1.Psychological tests and recruitment (source: Piotrowski and Armstrong 2006)
Box 1.2.The difficult division of a position into skills (source: Marchal 2015)
Box 1.3.The development of rating systems by a third party
1.1.1.2. Quantification of work and positions
Box 1.4.Taylorism or measuring work to improve productivity (source: Taylor 1919)
Box 1.5.The Hay method or measuring work to prioritize positions (source: Lemière and Silvera 2010)
1.1.2. Informing and justifying decisions concerning individuals
1.1.2.1. Enabling comparison by quantification: commensurability and classification
1.1.2.2. Justifying decisions
Box 1.6.Use of selection tests and legal remedies in the United States (source: Dobbin 2009)
1.2. From reporting to HR data analysis
1.2.1. HR reports and dashboards: definitions and examples
1.2.1.1. Legalreporting
Box 1.7.Legal reporting obligations in France
Box 1.8.GRI indicators as a means of ensuring the reliability of international comparisons (source: GRI official website)
1.2.1.2. HR dashboards
Box 1.9.The Gallup engagement measurement scale (source: Gallup official website; Little and Little 2006)
1.2.1.3. Reporting and dashboards, characterized by a bivariate vision and an objective of compliance
Box 1.10.Bivariate indicators of equal pay (source: Actual data from a large French company)
Box 1.11.Indicators produced to ensure compliance but not used (source: Coron 2018a)
1.2.2. HR analytics and statistical studies
1.2.2.1. HR analytics: definitions and examples
Box 1.12.Analyzing the determinants of occupational accidents (source: Pertinant et al. 2017)
Box 1.13.Analyzing the main lines of well-being at work (source: Salanova et al. 2014)
1.2.2.2. A multivariate approach, for an analytical, decision-making and argumentative purpose
Box 1.14.The business case for gender diversity
1.3. Big Data and the use of HR algorithms
1.3.1. Big Data in HR: definitions and examples
1.3.1.1. Big Data: generic definitions
Box 1.15.Big Data and the renewed interest in correlation (source: Mayer-Schönerger and Cukier 2014)
Box 1.16.The omnipresence of algorithms, a risk for equality? (source: O’Neil, 2016)
1.3.1.2. Big Data and the use of HR algorithms
Box 1.17.Examples of how to use Big Data in HR (source: O'Neil 2016; specialized HR websites)
Box 1.18.Examples of management by algorithms (source: Lee et al. 2015; Paye 2017)
1.3.2. The breaks introduced by Big Data in HR
1.3.2.1. Automation
1.3.2.2. Prediction
1.3.2.3. Customization
1.3.2.4. HR algorithms: potential, dangers and issues
2. Quantification and Decision-making
2.1. In search of objectivity
2.1.1. The myth of objective quantification
2.1.1.1. The origins of the myth
Box 2.1.From 1275 to 1325, the incarnations of the rise of quantification (source: Crosby 2003)
Box 2.2.“Realistic” discourses on quantification (source: Desrosières 2008a)
2.1.1.2. What are the characteristics of “objective quantification”?
2.1.2. Limited objectivity
2.1.2.1. Quantification conventions and the statisticalization of reality
Box 2.3.The intervention of the association “Vaincre la mucoviscidose” in the statisticalization of hospital costs (source: Juven 2016)
Box 2.4.Gender biases in job classification methods (source: Acker 1989; Lemière and Silvera 2010)
2.1.2.2. The biases of quantified evaluation
Box 2.5.Different types of bias most common in quantified evaluations (source: Kahneman 2015; Gilbert and Yalenios 2017)
2.1.3. Objectivity, a central issue in HR
2.1.3.1. The myth of objective quantification in the HR field
Box 2.6.Trust in figures as a guarantee of objectivity (source: Coron and Pigeyre 2018)
2.1.3.2. The importance of (the illusion of) objectivity in the world of work
2.1.3.3. Quantification and reduction of the possibility of criticism
Box 2.7.When a complex statistical tool prevents criticism (source: Study by the author; Coron 2018b)
2.2. In search of personalization
2.2.1. Are we reaching the end of the positioning of statistics as a science of large numbers?
2.2.1.1. Statistics, the science of the collective and large numbers?
Box 2.8.The law of large numbers
Box 2.9.The notion of representativeness (source: Fox, 1999; Didier, 2011)
2.2.1.2. Using statistics to customize
Box 2.10.Targeted advertising (source: Peyrat, 2009; Kessous, 2012a)
Box 2.11.Taking into account user feedback
2.2.2. Personalization: a challenge for the HR function
2.2.2.1. A model from marketing
Box 2.12.From segmentation to personalization in marketing
2.2.2.2. The misleading horizon of an individualizing HRM?
2.3. In search of predictability
2.3.1. Are we heading toward a rise in predictability at the expense of understanding?
2.3.1.1. Statistics, the science of description and explanation, but also of prediction?
Box 2.13.So-called predictive analysis (source: Mayer-Schönberger and Cukier 2014)
Box 2.14.Predictive analysis, a sign of a lack of interest in the meaning and interpretation of models or the total disappearance of models (Meyer-Schönberger and Cukier, 2014; Kitchin, 2014; Cardon, 2018, and study by the author)
Box 2.15.Measuring the relevance of predictive analysis (source: Study by the author)
2.3.1.2. Prediction or performativity?
2.3.2. The predictive approach: an issue for the HR function
2.3.2.1. An issue in the relationship with employees
Box 2.16.Setting up a system of training suggestions for employees (source: Study by the author)
2.3.2.2. An issue in the relationship with the company management
Box 2.17.Predicting resignations (source: Press articles3; Yang et al. 2018)
3. How are Quantified HR Management Tools Appropriated by Different Agents?
3.1. The different avatars of the link between managerial rationalization and quantification
3.1.1. Bureaucracy
3.1.1.1. The Weberian ideal type of bureaucracy and its extensions
3.1.1.2. The rational-legal authority of quantification
3.1.2. New Public Management
3.1.2.1. Rationalization over time
3.1.2.2. The role of quantification in the institutionalization and definition of NPM
Box 3.1.Measuring the activity of research institutions (source: Altbach 2015; Dehon et al. 2010; Espeland and Sauder 2007)
Box 3.2.Evaluation of the anonymous CV (source: Behaghel et al. 2011)
Box 3.3.An example of benchmarking at the European level, “social benchmarking” (source: Bruno 2010)
3.1.3. Algorithmic management
3.1.3.1. Algorithmic management and its challenges
Box 3.4.The notion of algorithmic management and the questions it raises
3.1.3.2. Extreme rationalization?
3.2. Distrust of data collection and processing
3.2.1. Providing data, not such a harmless approach for employees
3.2.1.1. One observation: employees hesitate to provide their data to the company
Box 3.5.Sharing photos on Flickr (source: Beuscart et al. 2009)
Box 3.6.The data deluge in a few figures (source: Domo 20181)
Box 3.7.The difficulties for a company in getting employees to declare their skills on the internal social network (source: study by the author)
3.2.1.2. Suggestions for an explanation
Box 3.8.Using data from social networks for disciplinary purposes (sources: press articles, blog articles4)
Box 3.9.Unions distrustful of employer data collection
3.2.2. Can numbers be made to reflect whatever we like?
3.2.2.1. The other side of the myth of objective quantification: the myth of instrumentalized quantification
Box 3.10.Two opposing interpretations of the same number (source: Coron 2018a)
3.2.2.2. How do we limit the instrumentalization of quantification?
3.3. Distrust of a disembodied decision
3.3.1. Decisions made solely on the basis of figures
3.3.1.1. Has the employee been silenced?
Box 3.11.The impossibility of making individual adjustments when the decision is based on figures in order for it to be more objective (source: Bourguignon and Chiapello 2005; Pichault and Nizet 2000)
3.3.1.2. The difficulty in taking into account particular circumstances
Box 3.12.Work performance and special circumstances
3.3.1.3. Decision-making without accountability
3.3.2. Decisions made solely by algorithms
3.3.2.1. The question of liability
Box 3.13.The “Moral Machine” study and the great variability of human decisions (source: official websites5 and press articles)
3.3.2.2. Algorithms perceived as black boxes: an impossibleempowerment?
4. What Effects are the Effects of Quantification on the Human Resources Function?
4.1. Quantification for HR policy evaluation?
4.1.1. Measuring the implementation of HR policies
4.1.1.1. The definition of monitoring indicators
Box 4.1.Alignment between policy objectives and follow-up indicators (source: Study by the author)
Box 4.2.From the planned policy to the implemented policy, a gap that is sometimes significant (sources: Khilji and Wang 2006; Guest 2011)
4.1.1.2. Monitoring indicators versus appropriation by local actors
Box 4.3.From selective policy appropriation to selective indicator appropriation appropriation
4.1.2. Measuring the effects of HR policies
4.1.2.1. The definition of quantified commitments
Box 4.4.The quantified commitments of disability policies, retention of older workers in employment, and gender equality policies (source: Online agreements; Claisse et al. 2011; Rabier 2009)
Box 4.5.Defining unattainable commitments to obtain a signature (source: Coron and Pigeyre 2018)
4.1.2.2. Is isolating the effects of HR policies an impossible task?
Box 4.6.Mobilizing projections to monitor structural effects (source: Study by the author)
4.2. Quantifying in order to legitimize the HR function?
4.2.1. Measuring the performance of the HR function
4.2.1.1. How can the performance of the HR function be defined?
Box 4.7.Proposals for indicators to measure performance of the HR function (source: Boudreau and Lawler 2015)
Box 4.8.Examples of effectiveness and efficiency indicators on HR processes (source: Cossette et al. 2014)
4.2.1.2. Choices of indicators according to the organizational context
4.2.2. Measuring the link between HR function performance and organizational performance
4.2.2.1. From HR function performance to employee behavior
4.2.2.2. From employee behaviors to organizational performance
Box 4.9.Example of switching from the first to the third step (source: Levenson et al. 2004)
4.3. The quantification and risk of HR business automation
4.3.1. HR professions with a high risk of automation
4.3.1.1. Automation risk factors
4.3.1.2. The HR professions concerned
Box 4.10.The automation of CV pre-selection, the promise of several companies (sources: Kuncel et al. 2014; company sites and study conducted by the author)
Box 4.11.HR administrative chatbots, a reality in the near future?
4.3.2. Support for the employees concerned
4.3.2.1. Professions that will disappear…
4.3.2.2. … or jobs that are likely to evolve?
5. The Ethical Issues of Quantification
5.1. Protection of personal data
5.1.1. Risks relating to personal data
5.1.1.1. Risks related to poor data integrity
Box 5.1.Data piracy suffered by Sony (sources: Press articles)
5.1.1.2. Risks related to the use of data
Box 5.2.The Facebook scandal – Cambridge Analytica (source: Press articles; CNIL 2017)
Box 5.3.Misappropriating video surveillance for disciplinary purposes (source: Baudoin et al. 2019)
Box 5.4.Misuse of sensitive data (source: UNESCO 2018)
5.1.2. Obligations and actions of companies with regard to the protection of personal data
5.1.2.1. The European regulation: employers’ obligations
Box 5.5.The main provisions of the GDPR that affect employers and the mobilization of HR algorithms
5.1.2.2. Other possible ways to ensure data security and accuracy: the example of the blockchain
Box 5.6.Using the blockchain to ensure integrity and reliability data in the HR field (source: Press articles; Baudoin et al. 2019)
5.2. Quantification and discrimination(s)
5.2.1. Quantification as a shield against discrimination
5.2.1.1. Quantification as a tool to reduce direct discrimination
Box 5.7.Unconscious discrimination and bias (source: Kahneman 2015; Amadieu 2016)
Box 5.8.Software that turns CVs into standardized information (source: Press articles)
Box 5.9.Using equations to recruit candidates (source: Kuncel et al. 2013, 2014)
5.2.1.2. Quantification as a tool for measuring discrimination
Box 5.10.Descriptive statistics and discrimination (source: Blendoor website)
Box 5.11.Testing methodologies (source: Amadieu 2016)
Box 5.12.The gender equality index in France and the commensurability of company situations
5.2.2. The risks of discrimination related to the use of quantification
5.2.2.1. The overvaluation of certain measurable criteria and the risk of indirect discrimination
5.2.2.2. The predictive approach and the risk of profile replication
Box 5.13.Recruitment algorithms, anti-discrimination and team diversity (source: Bender 2004)
5.3. Opening the “black box” of quantification
5.3.1. Training HR actors, employees and their representatives as well as data experts on HR quantification
5.3.1.1. Training the HR function to enable it to maintain informed control over quantification
Box 5.14.The black box of deep learning (source: CNIL 2017; Villani 2018)
5.3.1.2. Training employees to enable them to play with quantification tools
Box 5.15.Article 15.1 of the GDPR
5.3.1.3. Training staff representatives to establish a democratic debate on the subject
Box 5.16.A digital agreement providing for official information trade unions (source: Jeannin and Riche 2017)
5.3.1.4. Training data specialists on HR issues
5.3.2. Mobilizing organizational leverage
5.3.2.1. Creating new functions and responsibilities
Box 5.17.The role of the DPO (source: GDPR)
5.3.2.2. Formalizing the main principles
Conclusion
C.1. Summary of the book
C.2. Toward an analytical framework for HR quantification
References
Index. A
B
C
D
E
G, H, I, L
M, N
O, P
Q, R
S, T, U
WILEY END USER LICENSE AGREEMENT
Отрывок из книги
Technological Changes and Human Resources Set
.....
One, generally normative, tends to consider quantification as an effective solution to improve HR decision-making, whether in recruitment or other areas. This approach thus supports evidence-based management (EBM), in other words management based on evidence which is most often made up of figures and measurements. In the EBM approach, quantification is therefore proof and can cover a multiplicity of objects: quantifying to better evaluate individuals (in line with the psychotechnical approach), or to know them better, or to better understand global HR phenomena (absenteeism, gender equality), all in order to make better decisions. The EBM approach thus considers that quantification improves decision-making, processes and policies, including HR. Lawler et al. (2010) thus believe that the use of figures and the EBM approach have become central to making the HR function a strategic function of the company. For example, they identify three types of metrics of interest in an EBM approach: the efficiency and effectiveness of the HR function, and the impact of HR policies and practices on variables such as organizational performance. More generally, according to the work resulting from this approach, quantification makes it possible to meet several HR challenges. The first challenge is to make the right human resources management decisions: recruitment, promotion and salary increases, for example. The psychotechnical approach already mentioned seems to provide an answer to this first challenge: by measuring individuals’ skills, motivations and abilities in an objective way, it seems to guarantee greater objectivity and rigor in HR decision-making.
The second challenge is to define the right HR policies. Rasmussen and Ulrich (2015) thus give an example where an offshore drilling company uses quantification to define a policy linking management quality, operational performance and customer satisfaction (Box I.2). This example therefore illustrates how quantification can help identify problems and links between different factors in order to define more appropriate and effective HR policies.
.....