Seismic Reservoir Modeling
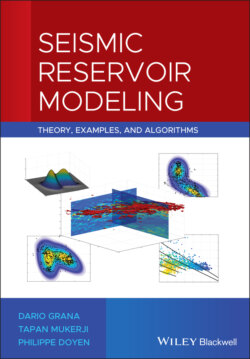
Реклама. ООО «ЛитРес», ИНН: 7719571260.
Оглавление
Dario Grana. Seismic Reservoir Modeling
Table of Contents
List of Tables
List of Illustrations
Guide
Pages
Seismic Reservoir Modeling. Theory, Examples, and Algorithms
Preface
Acknowledgments
1 Review of Probability and Statistics
1.1 Introduction to Probability and Statistics
1.2 Probability
Example 1.1
1.3 Statistics
1.3.1 Univariate Distributions
Example 1.2
1.3.2 Multivariate Distributions
1.4 Probability Distributions
1.4.1 Bernoulli Distribution
1.4.2 Uniform Distribution
1.4.3 Gaussian Distribution
1.4.4 Log‐Gaussian Distribution
1.4.5 Gaussian Mixture Distribution
1.4.6 Beta Distribution
1.5 Functions of Random Variable
1.6 Inverse Theory
1.7 Bayesian Inversion
Example 1.3
2 Rock Physics Models
2.1 Rock Physics Relations
2.1.1 Porosity – Velocity Relations
Example 2.1
2.1.2 Porosity – Clay Volume – Velocity Relations
2.1.3 P‐Wave and S‐Wave Velocity Relations
2.1.4 Velocity and Density
Example 2.2
2.2 Effective Media
2.2.1 Solid Phase
Example 2.3
Example 2.4
2.2.2 Fluid Phase
Example 2.5
2.3 Critical Porosity Concept
Example 2.6
2.4 Granular Media Models
Example 2.7
2.5 Inclusion Models
Example 2.8
2.6 Gassmann's Equations and Fluid Substitution
Example 2.9
2.7 Other Rock Physics Relations
2.8 Application
3 Geostatistics for Continuous Properties
3.1 Introduction to Spatial Correlation
3.2 Spatial Correlation Functions
Example 3.1
3.3 Spatial Interpolation
3.4 Kriging
3.4.1 Simple Kriging
Example 3.2
Example 3.3
3.4.2 Data Configuration
3.4.3 Ordinary Kriging and Universal Kriging
3.4.4 Cokriging
Example 3.4
3.5 Sequential Simulations
3.5.1 Sequential Gaussian Simulation
Example 3.5
3.5.2 Sequential Gaussian Co‐Simulation
Example 3.6
3.6 Other Simulation Methods
3.7 Application
4 Geostatistics for Discrete Properties
4.1 Indicator Kriging
Example 4.1
Example 4.2
4.2 Sequential Indicator Simulation
Example 4.3
Example 4.4
4.3 Truncated Gaussian Simulation
Example 4.5
4.4 Markov Chain Models
Example 4.6
4.5 Multiple‐Point Statistics
Example 4.7
4.6 Application
5 Seismic and Petrophysical Inversion
5.1 Seismic Modeling
Example 5.1
5.2 Bayesian Inversion
5.3 Bayesian Linearized AVO Inversion
5.3.1 Forward Model
5.3.2 Inverse Problem
Example 5.2
5.4 Bayesian Rock Physics Inversion
5.4.1 Linear – Gaussian Case
5.4.2 Linear – Gaussian Mixture Case
Example 5.3
5.4.3 Non‐linear – Gaussian Mixture Case
5.4.4 Non‐linear – Non‐parametric Case
Example 5.4
5.5 Uncertainty Propagation
Example 5.5
5.6 Geostatistical Inversion
5.6.1 Markov Chain Monte Carlo Methods
5.6.2 Ensemble Smoother Method
Example 5.6
5.6.3 Gradual Deformation Method
5.7 Other Stochastic Methods
6 Seismic Facies Inversion
6.1 Bayesian Classification
Example 6.1
Example 6.2
Example 6.3
Example 6.4
6.2 Bayesian Markov Chain Gaussian Mixture Inversion
Example 6.5
6.3 Multimodal Markov Chain Monte Carlo Inversion
Example 6.6
6.4 Probability Perturbation Method
6.5 Other Stochastic Methods
7 Integrated Methods
7.1 Sources of Uncertainty
7.2 Time‐Lapse Seismic Inversion
7.3 Electromagnetic Inversion
7.4 History Matching
7.5 Value of Information
8 Case Studies
8.1 Hydrocarbon Reservoir Studies
8.1.1 Bayesian Linearized Inversion
8.1.2 Ensemble Smoother Inversion
8.1.3 Multimodal Markov Chain Monte Carlo Inversion
8.2 CO2 Sequestration Study
Appendix: MATLAB Codes
A.1 Rock Physics Modeling
A.2 Geostatistical Modeling
A.3 Inverse Modeling
A.3.1 Seismic Inversion
A.3.2 Petrophysical Inversion
A.3.3 Ensemble Smoother Inversion
A.4 Facies Modeling
References
Index. a
b
c
d
e
f
g
h
i
j
k
l
m
n
o
p
q
r
s
t
u
v
w
z
WILEY END USER LICENSE AGREEMENT
Отрывок из книги
Dario Grana
.....
Figure 1.6 Examples of different correlations of the joint distribution of two random variables X and Y. The correlation coefficient ρX,Y is 0.9 and −0.6 in the top plots and approximately 0 in the bottom plots.
Different probability mass and density functions can be used for discrete and continuous random variables, respectively. For parametric distributions, the function is completely defined by a limited number of parameters (e.g. mean and variance). In this section, we review the most common probability mass and density functions. Probability mass functions are commonly used in geoscience problems for discrete random variables such as facies or rock types, whereas PDFs are used for continuous properties such as porosity, fluid saturations, density, P‐wave and S‐wave velocity. Some applications in earth sciences include mixed discrete–continuous problems with both discrete and continuous random variables.
.....