Statistics
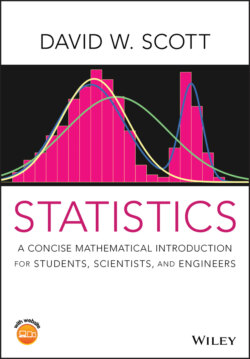
Реклама. ООО «ЛитРес», ИНН: 7719571260.
Оглавление
David W. Scott. Statistics
Table of Contents
List of Tables
List of Illustrations
Guide
Pages
Statistics. A Concise Mathematical Introduction for Students, Scientists, and Engineers
Preface
1 Data Analysis and Understanding
1.1 Exploring the Distribution of Data
1.1.1 Pearson's Father–Son Height Data
1.1.2 Lord Rayleigh's Data
1.1.3 Discussion
1.2 Exploring Prediction Using Data
1.2.1 Body and Brain Weights of Land Mammals
1.2.2 Space Shuttle Flight 25
1.2.3 Pearson's Father–Son Height Data Revisited
1.2.4 Discussion
Problems
2 Classical Probability
2.1 Experiments with Equally Likely Outcomes
2.1.1 Simple Outcomes
2.1.2 Compound Events and Set Operations
2.2 Probability Laws
2.2.1 Union and Intersection of Events and
2.2.1.1 Case (i)
2.2.1.2 Cases (ii) and (iii)
2.2.1.3 Case (iv)
2.2.2 Conditional Probability
2.2.2.1 Definition of Conditional Probability
2.2.2.2 Conditional Probability With More Than Two Events
2.2.3 Independent Events
2.2.4 Bayes Theorem
2.2.5 Partitions and Total Probability
2.3 Counting Methods
2.3.1 With Replacement
2.3.2 Without Replacement (Permutations)
2.3.3 Without Replacement or Order (Combinations)
2.3.4 Examples
2.3.5 Extended Combinations (Multinomial)
2.4 Countable Sets: Implications as
2.4.1 Selecting Even or Odd Integers
2.4.2 Selecting Rational Versus Irrational Numbers
2.5 Kolmogorov's Axioms
2.6 Reliability: Series Versus Parallel Networks
2.6.1 Series Network
2.6.2 Parallel Network
Problems
3 Random Variables and Models Derived From Classical Probability and Postulates
3.1 Random Variables and Probability Distributions: Discrete Uniform Example
3.1.1 Toss of a Single Die
3.1.2 Toss of a Pair of Dice
3.2 The Univariate Probability Density Function: Continuous Uniform Example
3.2.1 Using the PDF to Compute Probabilities
3.2.2 Using the PDF to Compute Relative Odds
3.3 Summary Statistics: Central and Non‐Central Moments
3.3.1 Expectation, Average, and Mean
3.3.2 Expectation as a Linear Operator
3.3.3 The Variance of a Random Variable
3.3.4 Standardized Random Variables
3.3.5 Higher Order Moments
3.3.6 Moment Generating Function
3.3.7 Measurement Scales and Units of Measurement
3.3.7.1 The Four Measurement Scales
3.3.7.2 Units of Measurement
3.4 Binomial Experiments
3.5 Waiting Time for a Success: Geometric PMF
3.6 Waiting Time for Successes: Negative Binomial
3.7 Poisson Process and Distribution
3.7.1 Moments of the Poisson PMF
3.7.2 Examples
3.8 Waiting Time for Poisson Events: Negative Exponential PDF
3.9 The Normal Distribution (Also Known as the Gaussian Distribution)
3.9.1 Standard Normal Distribution
3.9.2 Sums of Independent Normal Random Variables
3.9.3 Normal Approximation to the Poisson Distribution
Problems
4 Bivariate Random Variables, Transformations, and Simulations
4.1 Bivariate Continuous Random Variables
4.1.1 Joint CDF and PDF Functions
4.1.2 Marginal PDF
4.1.3 Conditional Probability Density Function
4.1.4 Independence of Two Random Variables
4.1.5 Expectation, Correlation, and Regression
4.1.5.1 Covariance and Correlation
4.1.5.2 Regression Function
4.1.6 Independence of Random Variables
4.1.7 Bivariate Normal PDF
4.1.8 Correlation, Independence, and Confounding Variables
4.2 Change of Variables
4.2.1 Examples: Two Uniform Transformations
4.2.2 One‐Dimensional Transformations
4.2.2.1 Example 1: Negative exponential PDF
4.2.2.2 Example 2: Cauchy PDF
4.2.2.3 Example 3: Chi‐squared PDF with one degree of freedom
4.2.3 Two‐Dimensional Transformations
4.3 Simulations
4.3.1 Generating Uniform Pseudo‐Random Numbers
4.3.1.1 Reproducibility
4.3.1.2 RANDU
4.3.2 Probability Integral Transformation
4.3.3 Event‐driven Simulation
Problems
5 Approximations and Asymptotics
5.1 Why Do We Like Random Samples?
5.1.1 When Takes a Product Form
5.1.2 When Takes a Summation Form
5.2 Useful Inequalities
5.2.1 Markov's Inequality
5.2.2 Chebyshev's Inequality
5.2.3 Jensen's Inequality1
5.2.4 Cauchy–Schwarz Inequality
5.3 Sequences of Random Variables
5.3.1 Weak Law of Large Numbers
5.3.2 Consistency of the Sample Variance
5.3.3 Relationships Among the Modes of Convergence
5.3.3.1 Proof of Result (5.21)
5.3.3.2 Proof of Result (5.22)2
5.4 Central Limit Theorem
5.4.1 Moment Generating Function for Sums
5.4.2 Standardizing the Sum
5.4.3 Proof of Central Limit Theorem
5.5 Delta Method and Variance‐stabilizing Transformations
Problems
Notes
6 Parameter Estimation
6.1 Desirable Properties of an Estimator
6.2 Moments of the Sample Mean and Variance
6.2.1 Theoretical Mean and Variance of the Sample Mean
6.2.2 Theoretical Mean of the Sample Variance
6.2.3 Theoretical Variance of the Sample Variance
6.3 Method of Moments (MoM)
6.4 Sufficient Statistics and Data Compression
6.5 Bayesian Parameter Estimation
6.6 Maximum Likelihood Parameter Estimation
6.6.1 Relationship to Bayesian Parameter Estimation
6.6.2 Poisson MLE Example
6.6.3 Normal MLE Example
6.6.4 Uniform MLE Example
6.7 Information Inequalities and the Cramér–Rao Lower Bound
6.7.1 Score Function
6.7.2 Asymptotics of the MLE
6.7.3 Minimum Variance of Unbiased Estimators
6.7.4 Examples
Problems
7 Hypothesis Testing
7.1 Setting up a Hypothesis Test
7.1.1 Example of a Critical Region
7.1.2 Accuracy and Errors in Hypothesis Testing
7.2 Best Critical Region for Simple Hypotheses
7.2.1 Simple Example Continued
7.2.2 Normal Shift Model with Common Variance
7.3 Best Critical Region for a Composite Alternative Hypothesis
7.3.1 Negative Exponential Composite Hypothesis Test
7.3.1.1 Example
7.3.1.2 Alternative Critical Regions
7.3.1.3 Mount St. Helens Example
7.3.2 Normal Shift Model with Common But Unknown Variance: The ‐test
7.3.3 The Random Variable
7.3.3.1 Where We Show and Are Independent
7.3.3.2 Where We Show That Scaled Is
7.3.3.3 Where We Finally Derive the PDF
7.3.4 The One‐Sample ‐test
7.3.5 Example
7.3.6 Other ‐tests
7.3.6.1 Paired ‐test
7.3.6.2 Two‐Sample ‐test
7.3.6.3 Example Two‐Sample ‐test: Lord Rayleigh's Data
7.4 Reporting Results: ‐values and Power
7.4.1 Example When the Null Hypothesis Is Rejected
7.4.2 When the Null Hypothesis is Not Rejected
7.4.3 The Power Function
7.5 Multiple Testing and the Bonferroni Correction
Problems
8 Confidence Intervals and Other Hypothesis Tests
8.1 Confidence Intervals
8.1.1 Confidence Interval for : Normal Data, Known
8.1.2 Confidence Interval for : Unknown
8.1.3 Confidence Intervals and ‐values
8.2 Hypotheses About the Variance and the ‐Distribution
8.2.1 The ‐Distribution
8.2.2 Hypotheses About the Value of the Variance
8.2.3 Confidence Interval for the Variance
8.2.4 Two‐Sided Alternative for Testing
8.3 Pearson's Chi‐Squared Tests
8.3.1 The Multinomial PMF
8.3.2 Goodness‐of‐Fit (GoF) Tests
8.3.3 Two‐Category Binomial Case
8.3.4 ‐Category Multinomial Case
8.3.5 Goodness‐of‐Fit Test for a Parametric Model
8.3.6 Tests for Independence in Contingency Tables
8.4 Correlation Coefficient Tests and CIs
8.4.1 How to Test if the Correlation
8.4.2 Confidence Intervals and Tests for a General Correlation Coefficient
8.5 Linear Regression
8.5.1 Least Squares Regression
8.5.2 Distribution of the Least‐Squares Parameters
8.5.3 A Confidence Interval for the Slope
8.5.4 A Two‐side Hypothesis Test for the Slope
8.5.5 Predictions at a New Value
8.5.6 Population Interval at a New Value
8.6 Analysis of Variance
Problems
9 Topics in Statistics
9.1 MSE and Histogram Bin Width Selection
9.1.1 MSE Criterion for Biased Estimators
9.1.2 Case Study: Optimal Histogram Bin Widths
9.1.3 Examples with Normal Data
9.1.4 Normal Reference Rules for the Histogram Bin Width
9.1.4.1 Scott's Rule
9.1.4.2 Freedman–Diaconis Rule
9.1.4.3 Sturges' Rule
9.1.4.4 Comparison of the Three Rules
9.2 An Optimal Stopping Time Problem
9.3 Compound Random Variables
9.3.1 Computing Expectations with Conditioning
9.3.2 Sum of a Random Number of Random Variables
9.4 Simulation and the Bootstrap
9.5 Multiple Linear Regression
9.6 Experimental Design
9.7 Logistic Regression, Poisson Regression, and the Generalized Linear Model
9.8 Robustness
9.9 Conclusions
Appendices
A Notation Used in This Book
B Common Distributions
C Using R and Mathematica For This Text. C.1 R Language – The Very Basics
C.2 Mathematica – The Basics
Bibliography
Index
WILEY END USER LICENSE AGREEMENT
Отрывок из книги
David W. Scott
.....
The second fundamental task of statistics is prediction. Data for this task are typically ordered pairs, . The goal is to predict the value of the variable using the corresponding value of the variable. For example, we might try to predict a son's height () knowing the father's height (). Or a bank contemplating a mortgage loan may use a person's credit score to predict the probability the person will default on the loan.
The initial step is to plot a scatter diagram of the data points in order to determine if there is a strong relationship between and . The relationship, if it exists, is linear or nonlinear. If knowledge of does not convey any information about the value of , then the scatter diagram will have no slope or trends, with values just scattered around their average.
.....