Statistical Methods and Modeling of Seismogenesis
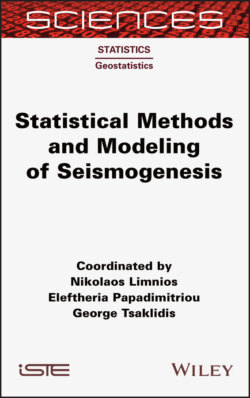
Реклама. ООО «ЛитРес», ИНН: 7719571260.
Оглавление
Eleftheria Papadimitriou. Statistical Methods and Modeling of Seismogenesis
Table of Contents
List of Illustrations
List of Table
Guide
Pages
Statistical Methods and Modeling of Seismogenesis
Preface
1. Kernel Density Estimation in Seismology
1.1. Introduction
1.2. Complexity of magnitude distribution
1.3. Kernel estimation of magnitude distribution
1.4. Implications for hazard assessments
1.5. Interval estimation of magnitude CDF and related hazard parameters
1.6. Transformation to equivalent dimensions
1.7. References
2. Earthquake Simulators Development and Application
2.1. Introduction
2.2. Development of earthquake simulators in the seismological literature
2.2.1. ALLCAL
2.2.2. Virtual quake
2.2.3. RSQSim
2.2.4. ViscoSim
2.2.5. Other simulation codes
2.2.6. Comparisons among simulators
2.3. Conceptual evolution of a physics-based earthquake simulator
2.3.1. A physics-based earthquake simulator (2015)
2.3.2. Frequency-magnitude distribution of the simulated catalog (2015)
2.3.3. Temporal features of the synthetic catalog (2015)
2.3.4. Improvements in the physics-based earthquake simulator (2017–2018)
2.3.5. Application to the seismicity of Central Italy
2.3.6. Further improvements of the simulator code (2019)
2.4. Application of the last version of the simulator to the Nankai mega-thrust fault system
2.5. Appendix 1: Relations among source parameters adopted in the simulation model
2.6. Appendix 2: Outline of the simulation program
2.7. References
3. Statistical Laws of Post-seismic Activity
3.1. Introduction
3.2. Earthquake productivity
3.2.1. The proposed method to study productivity
3.2.2. Earthquake productivity at the global level
3.2.3. Independence of the proximity function
3.2.4. Earthquake productivity at the regional level
3.2.5. Productivity in relation to the threshold of the proximity function
3.2.6. Discussion
3.3. Time-dependent distribution of the largest aftershock magnitude
3.3.1. The distribution of the magnitude of the largest aftershock in relation to time
3.3.2. The agreement between the dynamic Båth law and observations
3.3.3. Discussion
3.4. The distribution of the hazardous period
3.4.1. A model for the duration of the hazardous period
3.4.2. Determining the model parameters
3.4.3. Using the early aftershocks
3.5. Conclusion
3.6. References
4. Explaining Foreshock and the Båth Law Using a Generic Earthquake Clustering Model
4.1. Introduction
4.1.1. Issues related to foreshocks
4.1.2. Issues related to the Båth law
4.1.3. Study objectives
4.2. Theories related to foreshock probability and the Båth law under the assumptions of the ETAS model. 4.2.1. Space–time ETAS model, stochastic declustering and classification of earthquakes
4.2.2. Master equation
4.2.3. Asymptotic property ofF (m′)
4.2.4. Foreshock probabilities and their magnitude distribution in the ETAS model
4.2.5. Explanation of the Båth law by the ETAS model
4.3. Foreshock simulations based on the ETAS model. 4.3.1. Works by Helmstetter and others
4.3.2. Works by Zhuang and others
4.3.3. Evidence of statistics between mainshocks and foreshocks
4.3.4. Different simulation results
4.4. Simulation of the Båth law based on the ETAS model. 4.4.1. On the simulation study by Helmstetter
4.4.2. Observation on Båth’s law for volcanic earthquake swarms
4.5. Conclusion. 4.5.1. Back to the starting point
4.5.2. On the comparison between foreshock probability in the ETAS model and real catalogs
4.5.3. Impracticality of the foreshock concept
4.5.4. What should we do?
4.6. Acknowledgments
4.7. References
5. The Genesis of Aftershocks in Spring Slider Models
5.1. Introduction
5.2. The rate-and-state equation
5.3. The Dieterich model
5.3.1. Time to instability
5.3.2. Initial conditions during stationary seismicity
5.3.3. Effect of a constant stress increase Δτ
5.4. The mechanics of afterslip
5.5. The two-block model
5.5.1. Synthetic catalogs
5.5.1.1. Gutenberg–Richter law
5.6. Conclusion
5.7. References
6. Markov Regression Models for Time Series of Earthquake Counts
6.1. Introduction
6.2. Markov regression HMMs: definition and notation
6.3. Application. 6.3.1. Data
6.3.2. Results
6.4. Conclusion
6.5. Acknowledgments
6.6. References
7. Scaling Properties, Multifractality and Range of Correlations in Earthquake Time Series: Are Earthquakes Random?
7.1. Introduction
7.2. The range of correlations in earthquake time series
7.2.1. Short-range correlations
7.2.2. Long-range correlations
7.3. Scaling properties of earthquake time series
7.3.1. The probability distribution function
7.3.2. A stochastic dynamic mechanism with memory effects
7.3.3. The cumulative distribution function
7.4. Fractal and multifractal structures
7.5. Discussion and conclusions
7.6. References
8. Self-correcting Models in Seismology: Possible Coupling Among Seismic Areas
8.1. Introduction
8.2. Review of applications
8.3. Formulation of the models. 8.3.1. Simple Stress Release Model
8.3.2. Independent Stress Release Model
8.3.3. Linked Stress Release Model
8.4. Applications
8.4.1. Greece and the surrounding area
8.4.2. Gulf of Corinth
8.5. Conclusion
8.6. References
9. Markovian Arrival Processes for Earthquake Clustering Analysis
9.1. Introduction
9.2. State of the art. 9.2.1. Earthquake clustering methods and applications
9.2.2. Hidden Markov models and applications in seismology
9.3. Markovian Arrival Process
9.3.1. Definition and basic results
9.3.2. Parameter fitting
9.3.3. Inference of the latent states
9.4. Methodology and results. 9.4.1. Motivation
9.4.2. Clustering detection procedure
9.5. Conclusion
9.6. References
10. Change Point Detection Techniques on Seismicity Models
10.1. Introduction
10.2. The change point framework
10.3. Changes in a Poisson process
10.4. Changes in the Epidemic Type Aftershock Sequence model
10.5. Changes in the Gutenberg–Richter law
10.6. ZMAP
10.7. Other statistical tests
10.8. Detection of changes without hypothesis testing
10.9. Discussion and conclusion
10.10. References
11. Semi-Markov Processes for Earthquake Forecast
11.1. Introduction
11.2. Semi-Markov processes – preliminaries
11.2.1. Special class of distributions
11.3. Transition probabilities and earthquake occurrence
11.3.1. Likelihood and estimation
11.4. Semi-Markov transition matrix
11.5. Illustrative example
11.6. References
List of Authors
Index
WILEY END USER LICENSE AGREEMENT
Отрывок из книги
Statistics, Field Director – Nikolaos Limnios and Kerrie Mengersen Geostatistics
.....
When the existence of a strict, single value upper bound to the magnitude range, Mmax, is assumed, the estimators of magnitude distribution functions are:
.....