A Framework of Human Systems Engineering
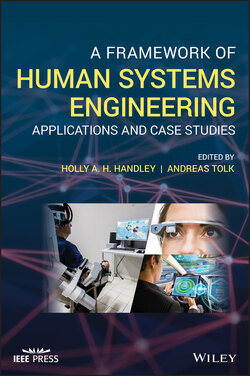
Реклама. ООО «ЛитРес», ИНН: 7719571260.
Оглавление
Группа авторов. A Framework of Human Systems Engineering
Table of Contents
List of Tables
List of Illustrations
Guide
Pages
A Framework of Human Systems Engineering. Applications and Case Studies
Editor Biographies
Contributors List
Foreword
Preface
1 Introduction to the Human Systems Engineering Framework
1.1 Introduction
1.2 Human‐Centered Disciplines
1.3 Human Systems Engineering
1.4 Development of the HSE Framework
1.5 HSE Applications
1.6 Conclusion
References
2 Human Interface Considerations for Situational Awareness
2.1 Introduction
2.2 Situational Awareness: A Global Challenge
2.3 Putting Situational Awareness in Context: First Responders
2.4 Deep Dive on Human Interface Considerations
2.5 Putting Human Interface Considerations in Context: Safe Cities
2.6 Human Interface Considerations for Privacy‐Aware SA
Reference
Notes
3 Utilizing Artificial Intelligence to Make Systems Engineering More Human*
3.1 Introduction
3.2 Changing Business Needs Drive Changes in Systems Engineering
3.3 Epoch 4: Delivering Capabilities in the Sociotechnical Ecosystem
3.3.1 A Conceptual Architecture for Epoch 4
3.3.2 Temporal Sociotechnical Measures
3.3.3 Systems Engineering Frameworks
3.3.3.1 Sociotechnical Network Models
3.3.3.2 Digital Twins
3.4 The Artificial Intelligence Opportunity for Building Sociotechnical Systems
3.5 Using AI to Track and Interpret Temporal Sociotechnical Measures
3.6 AI in Systems Engineering Frameworks
3.7 AI in Sociotechnical Network Models
3.8 AI‐Based Digital Twins
3.9 Discussion
3.10 Case Study
3.11 Systems Engineering Sociotechnical Modeling Approach
3.11.1 Modeling the Project
3.12 Results
3.13 Summary
References
Note
4 Life Learning of Smart Autonomous Systems for Meaningful Human‐Autonomy Teaming
4.1 Introduction
4.2 Trust in Successful Teaming
4.3 Meaningful Human‐Autonomy Teaming
4.4 Systematic Taxonomy for Iterative Through‐Life Learning of SAS
4.5 Ensuring Successful SAS
4.6 Developing Case Study: Airborne Shepherding SAS
4.7 Conclusion
Acknowledgment
References
Notes
5 Modeling the Evolution of Organizational Systems for the Digital Transformation of Heavy Rail
5.1 Introduction
5.2 Organizational System Evolution. 5.2.1 Characteristics of Organizational Systems
5.2.2 The Organization in Flux
5.2.3 Introducing New Technologies
5.3 Model‐Based Systems Engineering
5.4 Modeling Approach for the Development of OCMM
5.4.1 Technology Specification
5.4.2 Capture System Change
5.4.3 Capture Organizational Changes
5.4.4 Manage Organization Change
5.4.5 Analyze Emergent System
5.5 Implementation
5.5.1 User Portals
5.5.2 OCMM Metamodel
5.6 Case Study: Digital Transformation in the Rail Industry
5.6.1 Technology Specification
5.6.2 Capture System Change
5.6.3 Capture Organization Changes
5.6.4 Organization Change Management
5.6.5 Analyze Emergent System
5.6.5.1 Situation Awareness
5.6.5.2 Workload Analysis
5.6.5.2.1 In2Rail Workload Predesign
5.6.5.2.2 NASA TLX Workload Survey
5.7 OCMM Reception
5.8 Summary and Conclusions
References
6 Human Systems Integration in the Space Exploration Systems Engineering Life Cycle
6.1 Introduction
6.2 Spacecraft History
6.2.1 Mercury/Gemini/Apollo
6.2.2 Space Shuttle
6.2.3 International Space Station
6.2.4 Orion Spacecraft
6.3 in the NASA Systems Engineering Process
6.3.1 NASA Systems Engineering Process and HSI
6.4 Mission Challenges. 6.4.1 Innovation and Future Vehicle Designs Challenge
6.4.2 Operations Challenges
6.4.3 Maintainability and Supportability Challenges
6.4.4 Habitability and Environment Challenges
6.4.5 Safety Challenges
6.4.6 Training Challenges
6.5 Conclusions
References
7 Aerospace Human Systems IntegrationEvolution over the Last 40 Years
7.1 Introduction
7.2 Evolution of Aviation: A Human Systems Integration Perspective
7.3 Evolution with Respect to Models, Human Roles, and Disciplines. 7.3.1 From Single‐Agent Interaction to Multi‐agent Integration
7.3.2 Systems Management and Authority Sharing
7.3.3 Human‐Centered Disciplines Involved
7.3.4 From Automation Issues to Tangibility Issues
7.4 From Rigid Automation to Flexible Autonomy
7.5 How Software Took the Lead on Hardware
7.6 Toward a Human‐Centered Systemic Framework. 7.6.1 System of Systems, Physical and Cognitive Structures and Functions
7.6.2 Emergent Behaviors and Properties
7.6.3 System of Systems Properties
7.7 Conclusion and Perspectives
References
Notes
8 Building a Socio‐cognitive Evaluation Framework to Develop Enhanced Aviation Training Concepts for Gen Y and Gen Z Pilot Trainees
8.1 Introduction
8.1.1 Gamification Coupled with Cognitive Neuroscience and Data Analysis
8.1.2 Generational Differences in Learning
8.2 Virtual Technologies in Aviation
8.2.1 Potential Approaches for Incorporating Virtual Technologies
8.3 Human Systems Engineering Challenges
8.4 Potential Applications Beyond Aviation Training
8.5 Looking Forward
Acknowledgement
References
9 Improving Enterprise Resilience by Evaluating Training System ArchitectureMethod Selection for Australian Defense
9.1 Introduction
9.2 Defense Training System. 9.2.1 DTS Conceptualization
9.2.2 DTS as an Extended Enterprise Systems
9.2.3 Example: Navy Training System
9.2.3.1 Navy Training System as a Part of DTS
9.2.3.2 Navy Training System as a Part of DoD
9.3 Concept of Resilience in the Academic Literature
9.3.1 Definition of Resilience: A Multidisciplinary and Historical View
9.3.2 Definition of Resilience: Key Aspects
9.3.2.1 What? (Resilience Is and Is Not)
9.3.2.1.1 Resilience as a Singular System's Attribute
9.3.2.1.2 Resilience as a Collection of System's Attributes
9.3.2.2 Why? (Resilience Triggers)
9.3.2.3 How? (Resilience Mechanisms and Measures)
9.3.2.3.1 Resilience Mechanisms
9.3.2.3.2 Measuring Resilience
9.4 DTS Case Study Methodology
9.4.1 DTS Resilience Measurement Methodology
9.4.2 DTS Architecture
9.4.3 DTS Resilience Survey
9.4.3.1 DTS Resilience Survey Design
9.4.3.2 DTS Resilience Survey Conduct
9.5 Research Findings and Future Directions
References
10 Integrating New Technology into the Complex System of Air Combat Training*
10.1 Introduction
10.2 Method. 10.2.1 Data Collection
10.2.2 Data Analysis
10.3 Results and Discussion
10.3.1 Unseen Aircraft Within Visual Range
10.3.2 Unexpected Virtual and Constructive Aircraft Behavior
10.3.3 Complacency and Increased Risk Taking
10.3.4 Human–Machine Interaction
10.3.5 Exercise Management
10.3.6 Big Picture Awareness
10.3.7 Negative Transfer of Training to the Operational Environment
10.4 Conclusion
Acknowledgments
References
Note
11 Engineering a Trustworthy Private Blockchain for Operational Risk ManagementA Rapid Human Data Engineering Approach Based on Human Systems Engineering
11.1 Introduction
11.2 Human Systems Engineering and Human Data Engineering
11.3 Human‐Centered System Design
11.4 Practical Issues Leading to Large Complex Blockchain System Development. 11.4.1 Human‐Centered Operational Risk Management
11.4.2 Issues Leading to Risk Management Innovation Through Blockchain
11.4.3 Issues in Engineering Trustworthy Private Blockchain
11.5 Framework for Rapid Human Systems–Human Data Engineering
11.6 Human Systems Engineering for Trustworthy Blockchain. 11.6.1 Engineering Trustworthy Blockchain
11.6.2 Issues and Challenges in Trustworthy Private Blockchain
11.6.3 Concepts Used in Trustworthy Private Blockchain
11.6.4 Prototype Scenario for Trusted Blockchain Network
11.6.5 Systems Engineering of the Chain of Trust
11.6.6 Design Public Key Infrastructure (PKI) for Trust
11.6.6.1 Design of Certificate Authority (CA)
11.6.6.2 Design the Trusted Gateways
11.6.6.3 Involving Trusted Peers and Orderers
11.6.6.4 Facilitate Trust Through Channels
11.7 From Human System Interaction to Human Data Interaction
11.8 Future Work for Trust in Human Systems Engineering
11.8.1 Software Engineering of Trust for Large Engineered Complex Systems
11.8.2 Human‐Centered AI for the Future Engineering of Intelligent Systems
11.8.3 Trust in the Private Blockchain for Big Complex Data Systems in the Future
11.9 Conclusion
Acknowledgment
References
12 Light’s Properties and Power in Facilitating Organizational Change
12.1 Introduction
12.2 Implicit Properties and a Mathematical Model of Light
12.3 Materialization of Light
12.3.1 The Electromagnetic Spectrum
12.3.2 Quantum Particles
12.3.3 The Periodic Table and Atoms
12.3.4 A Living Cell
12.3.5 Fundamental Capacities of Self
12.4 Leveraging Light to Bring About Organizational Change
12.5 Summary and Conclusion
References
13 Observations of Real‐Time Control Room Simulation
13.1 Introduction. 13.1.1 What Is a “Real‐Time Control Room Simulator”?
13.1.2 What Is It Used For?
13.1.3 What Does It Look Like?
13.1.4 How Will They Develop?
13.2 Future General‐Purpose Simulators
13.2.1 Future On‐Site Simulators
13.3 Operators
13.4 Data
13.5 Measurement
13.5.1 Objective Measures
13.5.1.1 Recommended
13.5.1.2 Not Recommended
13.5.2 Subjective Measures
13.5.2.1 Recommended
13.5.2.2 Not Recommended
13.6 Conclusion
Disclaimer
References
Note
14 A Research Agenda for Human Systems Engineering
14.1 The State of Human Systems Engineering
14.2 Recommendations from the Chapter Contributions
14.2.1 Data and Visualization Challenges
14.2.2 Next‐Generation Computing
14.2.3 Advanced Methods and Tools
14.2.4 Increased Integration of Social Components into System Artifacts
14.3 Uniting the Human Systems Engineering Stakeholders
14.3.1 Transdisciplinary Approach
14.3.2 Common Formalisms
14.3.3 Common Metrics
14.4 Summary
Disclaimer
References
Index. a
b
c
d
e
f
g
h
i
k
l
m
n
o
p
r
s
t
u
v
w
WILEY END USER LICENSE AGREEMENT
Отрывок из книги
IEEE Press 445 Hoes Lane Piscataway, NJ 08854
.....
Traditional risks in typical system development projects and their evolution are well documented in literature (Warkentin et al., 2009), but analytical analysis of risk particularly in the sociotemporal space is largely a manual process. An AI‐based risk assessor can monitor trends in the sociotechnical measures to examine if there are emerging risks from a growing misalignment between groups of stakeholders. An AI risk assessor can develop forecasts of emerging risks that can be modeled by deliberate introduction of possible evidence that may occur in the future. Modeling in this fashion provides the capability to proactively assess system risk areas and take preventive steps.
In addition to localized risk identification, a holistic view of systemic risk across the sociotechnical ecosystem becomes more feasible using AI‐based models. While localized gross individual misalignment may not manifest itself, a general trend toward misalignment across the enterprise can be discovered and identified. Whereas the values for sociotemporal measures typically have been discovered through interviews, AI offers the opportunity to make assessments based upon noninvasive approaches such as analyzing e‐mails and text messages. Applications like the hedonometer (Dodds et al., 2011) have been used for many years; it is suggested here that AI can tap into the results of the hedonometer for both localized and global assessments of risk.
.....