Advances in Electric Power and Energy
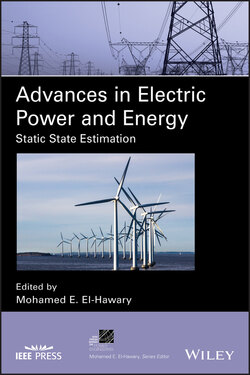
Реклама. ООО «ЛитРес», ИНН: 7719571260.
Оглавление
Группа авторов. Advances in Electric Power and Energy
Table of Contents
List of Tables
List of Illustrations
Guide
Pages
ADVANCES IN ELECTRIC POWER AND ENERGY. Static State Estimation
TO FRED C. SCHWEPPE, 1933–1988
ABOUT THE EDITOR
ABOUT THE CONTRIBUTORS
CHAPTER 1GENERAL CONSIDERATIONS
1.1 PRELUDE
1.2 DEFINING SSE
1.3 THE NEED FOR STATE ESTIMATION
1.4 STATIC STATE ESTIMATION IN PRACTICE
1.4.1 SE Performance Issues
1.4.2 Weights Assigned to Measurements
1.4.3 SE Availability Considerations
1.4.4 SE Solution Quality (Accuracy)
1.4.4.1 Metrics to Evaluate SE Solution Quality
1.4.4.2 Methods for Evaluating SE Solution Quality (Accuracy)
1.4.5 Using SE to Monitor External Facilities
1.4.6 SE Maintenance/Troubleshooting and Support Practices
1.5 APPLICATIONS THAT USE SE SOLUTION
1.5.1 Contingency Analysis
1.5.2 Power Flow (Online/Operator)
1.5.3 Locational Marginal Pricing
1.5.4 Security‐Constrained Economic Dispatch
1.5.5 Voltage Stability Assessment
1.5.6 Dynamic Stability Assessment
1.6 OVERVIEW OF CHAPTERS
REFERENCES
CHAPTER 2STATE ESTIMATION IN POWER SYSTEMS BASED ON A MATHEMATICAL PROGRAMMING APPROACH
2.1 INTRODUCTION
2.2 FORMULATION
Example 2.1 Traditional Formulation
2.3 CLASSICAL STATE ESTIMATION PROCEDURE
Example 2.2 Classical Solution Example
2.3.1 Bad Measurement Detection
Example 2.3 Bad Measurement Detection Example
2.3.2 Identification of Erroneous Measurements
Example 2.4 Bad Measurement Identification Example
2.4 MATHEMATICAL PROGRAMMING SOLUTION
Example 2.5 Mathematical Programming Problem
2.5 ALTERNATIVE STATE ESTIMATORS
2.5.1 Weighted Least of Squares
2.5.1.1 WLS General Formulation
2.5.2 Weighted Least Absolute Value
2.5.2.1 LAV General Formulation
2.5.2.2 LAV Mathematical Programming Formulation
2.5.3 Quadratic‐Constant Criterion
2.5.3.1 QC General Formulation
2.5.3.2 QC Mathematical Programming Formulation
2.5.4 Quadratic‐Linear Criterion
2.5.4.1 QL General Formulation
2.5.4.2 QL Mathematical Programming Formulation
2.5.5 Least Median of Squares
2.5.5.1 LMS General Formulation
2.5.5.2 LMS Mathematical Programming Formulation
2.5.6 Least Trimmed of Squares
2.5.6.1 LTS General Formulation
2.5.6.2 LTS Mathematical Programming Formulation
2.5.7 Least Measurements Rejected
2.5.7.1 LMR General Formulation
2.5.7.2 LMR Mathematical Programming Formulation
2.5.8 Formulation Overview
2.5.9 Illustrative Example
2.5.10 Case Study
2.5.10.1 Estimation Assessment
2.5.11 Results
2.5.11.1 Performance Analysis: Bad Data
2.5.12 Conclusions
REFERENCES
CHAPTER 3SYSTEM STRESS AND CASCADING BLACKOUTS
3.1 INTRODUCTION
3.2 CASCADING BLACKOUTS AND PREVIOUS WORK. 3.2.1 Cascading Blackouts
3.2.2 Typical Events
3.2.3 Prior Work on Blackouts
3.3 PROBLEM STATEMENT AND APPROACH. 3.3.1 Diagnosis of the Cascading Blackout Problem [19]
3.3.2 An Approach to Blackouts: Focus on Stress
3.3.3 Cascading Failure Networks
3.3.4 Stress Metrics
3.4 DFAXes, VULNERABILITY, AND CRITICALITY METRICS
3.4.1 DFAX Matrix
3.4.2 Vulnerability and Criticality
3.4.3 Rank and Degree
3.5 VALIDITY OF METRICS. 3.5.1 Proof of Validity of Metrics
3.5.2 Examples of Metrics
3.5.3 What Makes Metrics Valid and Useful
3.5.4 Why the Vulnerability and Criticality Metrics Are Valid and Useful
3.6 STUDIES WITH METRICS
3.6.1 Line Outage Distribution Factor Properties
3.6.2 Eastern Interconnection Study [19]
3.6.3 National System of Peru [19]
3.6.4 Western Interconnection of North America [26]
3.6.5 Tipping Points
3.6.6 Pre‐Blackout Stress
3.7 SUMMARY
3.8 APPLICATION OF STRESS METRICS
3.9 CONCLUSIONS
ACKNOWLEDGMENTS
REFERENCES
NOTES
CHAPTER 4MODEL‐BASED ANOMALY DETECTION FOR POWER SYSTEM STATE ESTIMATION
4.1 INTRODUCTION
4.2 CYBERATTACKS ON STATE ESTIMATION
4.2.1 State of the Art: Literature Survey
4.2.1.1 Cyberattacks on Analog Measurements
4.2.1.2 Cyberattacks on Topology Measurements
4.3 ATTACK‐RESILIENT STATE ESTIMATION
4.3.1 Attack Prevention
4.3.1.1 Measurement Design
4.3.1.1.1 Protecting Critical Measurements
4.3.1.1.2 MTD‐Based State Estimation
4.3.2 Attack Detection/Mitigation
4.3.2.1 Bad Data Detection
4.3.2.2 Model‐Based Anomaly Detection
4.3.2.3 Dynamic State Estimation
4.4 MODEL‐BASED ANOMALY DETECTION
4.4.1 Overall Methodology
4.4.2 Detailed Workflow
4.4.3 Case Study and Performance Evaluation on IEEE 14‐Bus System
4.4.3.1 System Model
4.4.3.2 Experimental Setup and Parameters
4.4.3.3 Illustrative Example for an Execution Interval
4.4.3.4 Detecting Stealthy False Data Injection Attacks
4.4.3.5 False Positive Rate (FPR) and True Positive Rate (TPR) Analysis
4.5 CONCLUSIONS
REFERENCES
CHAPTER 5PROTECTION, CONTROL, AND OPERATION OF MICROGRIDS
Acronyms
5.1 PRELUDE
5.2 INTRODUCTION
5.3 STATE OF THE ART IN MICROGRID PROTECTION AND CONTROL. 5.3.1 Present Protection Methods and Limitations
5.3.2 Performance of Legacy Protection Functions Applied to mGrids
Method 1: Legacy Distance Protection
Method 2: Legacy Line Differential Protection
Bolted Internal Fault Case
High Impedance Internal Fault Case
Method 3: Adaptive Protection Scheme with Microgrid Central Protection Unit (MCPU)
Step 1: Calculate the Settings of Any Relay
Step 2: Coordination Consideration of Relays
Method 4: Differential Energy‐Based Protection
Step 1: Calculate the Spectral Energy of the Interested Current
Step 2: Calculate the Differential Spectral Energy of Currents Between Two Terminals
Step 3: Compare the Differential Energy to the User‐Defined Threshold
Case 1: Grid‐Connected Mode
Case 2: Islanded Mode
5.3.3 Present Control Methods and Limitations
Case 1: HIGH‐VOLTAGE NETWORK
Method 1
Method 2
Case 2: Low‐Voltage Network
Limitations
5.4 EMERGING TECHNOLOGIES
Method 5: Dynamic State Estimation‐Based Protection (EBP)
Step 1: Build High‐Fidelity Dynamic Model of the Circuit Under Protection
Step 2: Calculate Confidence Level
Case 1
Case 2
5.4.1 Adaptive Setting‐Less Protection
5.4.2 Real‐Time Operation by the DERMS
5.5 TEST CASE FOR DDSE
5.5.1 Formulation of Measurement Models
5.5.2 Static State Estimation and Performance Evaluation
5.5.3 Test Scenarios
5.6 TEST RESULTS. 5.6.1 Test Case 1
5.6.2 Test Case 2
5.7 TEST CASE FOR ADAPTIVE SETTING‐LESS PROTECTION
5.7.1 Induction Machine Component Model
5.7.2 State Estimation Formulation
5.7.3 Test Scenarios
5.7.4 Test Results and Observation
5.8 CONCLUSIONS
REFERENCES
CHAPTER 6PSSE REDUX: CONVEX RELAXATION, DECENTRALIZED, ROBUST, AND DYNAMIC SOLVERS
6.1 INTRODUCTION
6.2 POWER GRID MODELING
6.3 PROBLEM STATEMENT
6.3.1 Weighted Least Squares Formulation
6.3.2 Cramér–Rao Lower Bound Analysis
Proposition 6.1
6.3.3 Gauss–Newton Iterations
6.3.4 Semidefinite Relaxation
Proposition 6.2
6.3.5 Penalized Semidefinite Relaxation
6.3.6 Feasible Point Pursuit
6.3.7 Synchrophasors
6.4 DISTRIBUTED SOLVERS
6.4.1 Distributed Linear Estimator
6.4.2 Distributed SDR‐Based Estimator
Proposition 6.3
6.5 ROBUST ESTIMATORS AND CYBERATTACKS
6.5.1 Bad Data Detection and Identification
6.5.2 Observability and Cyberattacks
Definition 6.1
Definition 6.2
6.6 POWER SYSTEM STATE TRACKING
6.6.1 Model‐Free State Tracking via Online Learning
6.6.2 Model‐Based State Tracking
6.7 DISCUSSION
ACKNOWLEDGMENTS
6.A APPENDIX
REFERENCES
CHAPTER 7ROBUST WIDE‐AREA FAULT VISIBILITY AND STRUCTURAL OBSERVABILITY IN POWER SYSTEMS WITH SYNCHRONIZED MEASUREMENT UNITS
7.1 INTRODUCTION
7.2 ROBUST FAULT VISIBILITY USING STRATEGICALLY DEPLOYED SYNCHRONIZED MEASUREMENTS
7.2.1 Wide‐Area Synchronized Measurement‐Based Fault Location Exploiting Traveling Waves
7.2.2 Optimal Deployment of Synchronized Sensors for Wide‐Area Fault Visibility
7.2.3 Application of Robust Estimation for Fault Location
7.2.4 Simulation Results on the Modified IEEE 118‐Bus System
7.3 OPTIMAL PMU DEPLOYMENT FOR SYSTEM‐WIDE STRUCTURAL OBSERVABILITY
7.3.1 Optimal PMU Deployment Considering Channel Limits
7.3.2 Modeling Zero‐Injection Buses
7.3.3 Optimal Deployment Accounting for Single PMU Loss
7.3.4 Consolidated PMU Deployment Results
7.4 CONCLUSIONS
ACKNOWLEDGMENTS
REFERENCES
CHAPTER 8A ROBUST HYBRID POWER SYSTEM STATE ESTIMATOR WITH UNKNOWN MEASUREMENT NOISE
8.1 INTRODUCTION
8.2 PROBLEM STATEMENT
8.3 PROPOSED FRAMEWORK FOR ROBUST HYBRID STATE ESTIMATION
8.3.1 Statistical Model of Noises
8.3.2 Determining Optimal Buffered PMU Data and Its Robust Covariance Matrix
8.3.2.1 Detect System Abnormality by Proposed Robust Mahalanobis Distances
Remark:
8.3.3 Proposed Robust Nonlinear State Estimation using SCADA Measurements
8.3.3.1 Asymptomatic Normality of Estimation Error Covariance Matrix
Theorem 8.1
8.3.3.2 Total Influence Function of the GM‐estimator
Remark:
8.3.4 State Fusion: Robust Linear State Estimation Incorporating Buffered PMU Measurements
8.3.5 Implementation Issues
8.4 NUMERICAL RESULTS
8.4.1 Gaussian Measurement Noise
8.4.2 Non‐Gaussian Measurement Noise
8.4.3 Robustness to Various Types of Bad Data
8.5 CONCLUSIONS
REFERENCES
CHAPTER 9LEAST‐TRIMMED‐ABSOLUTE‐VALUE STATE ESTIMATOR
9.1 BAD DATA DETECTION AND ROBUST ESTIMATORS. 9.1.1 Bad Data Detection
9.1.1.1 χ2 Distribution
9.1.1.2 χ2 Test for Bad‐Data Detection in WLS Estimator
9.1.2 Robust Estimators
9.1.2.1 M‐Estimator
9.1.2.2 L‐Estimator
9.1.2.3 R‐Estimator
9.1.3 Existing Robust Estimators
9.1.3.1 Least‐Absolute‐Value (LAV)
9.1.3.2 Least‐Median‐of‐Squares (LMedS)
9.1.3.3 Least‐Measurement‐Rejected (LMR)
9.1.3.4 Least‐Trimmed‐Square (LTS)
9.1.4 Proposed LTAV Robust Estimators
9.1.4.1 General Steps of LTAV Estimator
9.1.4.2 Mixed Integer Linear Programming Implementation
9.2 RESULTS AND DISCUSSION
9.2.1 6‐Bus System
9.2.1.1 6‐Bus DC State Estimation
9.2.1.1.1 Case 0: Basic Case with Only Random Noise
9.2.1.1.2 Case 1: Single Bad Data
9.2.1.1.3 Case 2: Multiple Noninteracting Bad Data
9.2.1.1.4 Case 3: Multiple Interacting Bad Data
9.2.1.1.5 Summary
9.2.1.2 5.1.2 6‐Bus AC State Estimation
9.2.1.2.1 Case 0: Basic Case with Only Random Noise
9.2.1.2.2 Case 1: Single Bad Data
9.2.1.2.3 Case 2: Multiple Noninteracting Bad Data
9.2.1.2.4 Case 3: Multiple Interacting Bad Data
9.2.1.2.5 Summary
9.2.2 14‐Bus System
9.2.3 30‐Bus System
9.2.4 Section Summary
9.3 CONCLUSIONS
9.A.1 6‐Bus DC System
9.A.2 6‐Bus AC System
9.B 14‐Bus AC System
9.C 30‐Bus AC System
REFERENCES
CHAPTER 10PROBABILISTIC STATE ESTIMATION IN DISTRIBUTION NETWORKS
10.1 INTRODUCTION
10.2 STATE ESTIMATION IN DISTRIBUTION NETWORKS
10.2.1 A Confidence‐Based Approach to State Estimation in Distribution Networks
10.2.2 State Estimation Accuracy
10.2.3 Computation Time and System Integration
10.2.4 Case Studies
10.3 IMPROVING OBSERVABILITY IN DISTRIBUTION NETWORKS
10.3.1 Probabilistic Approach to Observability
10.3.2 Probabilistic Observability Assessment Algorithm
10.3.3 Scalability
10.3.4 Case Studies
10.4 CONCLUSION
REFERENCES
CHAPTER 11ADVANCED DISTRIBUTION SYSTEM STATE ESTIMATION IN MULTI‐AREA ARCHITECTURES
11.1 ISSUES AND CHALLENGES OF DISTRIBUTION SYSTEM STATE ESTIMATION. 11.1.1 Distribution Grid Peculiarities
11.1.2 Future Scenarios
11.1.3 Distribution System State Estimation, DSSE
11.1.4 Open Issues of DSSE
11.1.5 Sensors and Smart Metering
11.1.6 Automation and Communication Requirements
11.1.7 Multi‐Area State Estimation
11.1.8 Multi‐Area Approaches
11.1.8.1 Level of Area Overlapping
11.1.8.2 Execution of the Estimation Processes
11.1.8.2.1 In‐Series Implementation
11.1.8.2.2 In‐Parallel Implementation
11.1.8.3 Architecture
11.1.8.3.1 Centralized Solution
11.1.8.3.2 Decentralized Architecture
11.1.8.4 Solution Methodology
11.2 DISTRIBUTION SYSTEM MULTI‐AREA STATE ESTIMATION (DS‐MASE) APPROACH
11.2.1 Multi‐Area Partition and Architecture
11.2.2 DS‐MASE Procedure
11.2.2.1 First Step
11.2.2.2 Second Step
11.2.3 Harmonization/Integration Method
11.2.3.1 Simplified WLS Procedure
11.2.3.2 Impact of Shared Measurements
11.2.3.3 Modified WLS Procedure
11.2.3.4 Summary of the Second‐Step Process
11.3 APPLICATION OF THE DS‐MASE APPROACH
11.3.1 Description of the Scenario and Assumptions
11.3.2 Example of MASE Applications. 11.3.2.1 Scenario 1: No Measurements in the Overlapping Nodes
11.3.2.2 Scenario 2: Coordinated Network Partition and Measurement System
11.3.2.3 Scenario 3: Meter Placement on a Pre‐existing Measurement System
11.3.2.4 Scenario 4: PMU‐Based Measurement System
11.4 VALIDITY AND APPLICABILITY OF DS‐MASE APPROACH
REFERENCES
CHAPTER 12HIERARCHICAL MULTI‐AREA STATE ESTIMATION
12.1 INTRODUCTION. 12.1.1 Problem Description
12.1.2 Classification of Existing Methods
12.1.2.1 Architecture: Hierarchical Versus Fully Decentralized
12.1.2.2 Optimality: Optimal Versus Suboptimal
12.1.2.3 Communication Frequency: Inter‐area and Intra‐area Gauss–Newton Iterations
12.1.3 Organization of this Chapter
12.2 PRELIMINARIES. 12.2.1 Measurement Model
12.2.2 Weighted Least Square (WLS) Estimator
12.2.3 Add‐On Functions
12.3 MODELING AND PROBLEM FORMULATION. 12.3.1 Multi‐area Power System Modeling
12.3.2 Centralized State Estimation
12.4 A BRIEF SURVEY OF SOLUTION TECHNIQUES. 12.4.1 Overview
12.4.2 Two‐Level Single‐Iteration State Estimators
12.4.3 Inter‐area Gauss–Newton State Estimators
12.4.4 Intra‐area Gauss–Newton State Estimators
12.5 HIERARCHICAL STATE ESTIMATOR VIA SENSITIVITY FUNCTION EXCHANGES. 12.5.1 Outline
12.5.2 Initialization
12.5.3 Local State Estimator
12.5.4 Coordinator's Problem
12.5.5 Complete Scheme
12.6 ADD‐ON FUNCTIONS IN MULTI‐AREA STATE ESTIMATION. 12.6.1 Observability Analysis
12.6.2 Bad Data Identification
12.7 PROPERTIES. 12.7.1 Assumptions
Assumption 12.1
Assumption 12.2
Assumption 12.3
12.7.2 Optimality
Theorem 12.1:
12.7.3 Convergence
Theorem 12.2:
12.7.4 Computation and Communication Costs
12.8 SIMULATIONS. 12.8.1 Tests Setup
12.8.2 Tests on the IEEE 14‐Bus System with Two Areas
12.8.3 Tests on the IEEE 118‐Bus System with Three Areas
12.8.4 Tests on Real Power System with Four Areas
12.9 CONCLUSIONS
ACKNOWLEDGMENTS
REFERENCES
NOTE
CHAPTER 13PARALLEL DOMAIN‐DECOMPOSITION‐BASED DISTRIBUTED STATE ESTIMATION FOR LARGE‐SCALE POWER SYSTEMS
13.1 INTRODUCTION
13.2 FUNDAMENTAL THEORY AND FORMULATION. 13.2.1 State Estimation Formulation
13.2.1.1 Weighted Least Square Static State Estimation
13.2.1.2 Extended Kalman Filter‐Based Dynamic State Estimation
13.2.1.2.1 Parameter Identification
13.2.1.2.2 State Prediction
13.2.1.2.3 State Filtering
13.2.2 Measurement and Component Modeling
13.2.3 Parallel Processing
13.2.3.1 CPU and GPU Architecture
13.2.3.2 OpenMP
13.2.3.2.1 Simple Example
13.2.3.3 Many‐Core GPU
13.2.3.3.1 Simple GPU Kernel
13.2.4 Numerical Methods for Solving Linear Systems
13.2.4.1 Direct Method
13.2.4.1.1 LU Decomposition
13.2.4.1.2 Cholesky Decomposition
13.2.4.2 Iterative Method
13.2.4.2.1 Preconditioned Conjugate Gradient Method
13.2.4.2.2 Gauss–Jacobi Methods
13.2.5 Additive Schwarz Method (ASM)
13.2.5.1 Domain Decomposition
13.2.6 Coherency Analysis
13.3 EXPERIMENTAL RESULTS
13.3.1 Hardware Setup
13.3.2 Extraction of Parallelism
13.3.3 Parallel ASM‐Based WLS Static State Estimation
13.3.3.1 Accuracy Analysis
13.3.3.2 Efficiency Analysis
13.3.4 Parallel ASM‐Based Dynamic State Estimation on GPU
13.3.4.1 Hierarchy of Parallelism
13.3.4.2 Accuracy Evaluation
13.3.4.3 Efficiency Analysis
13.4 CONCLUSION
REFERENCES
CHAPTER 14DISHONEST GAUSS–NEWTON METHOD‐BASED POWER SYSTEM STATE ESTIMATION ON A GPU
14.1 INTRODUCTION
14.2 BACKGROUND. 14.2.1 Nonlinear Power Flow Equations
14.2.2 Weighted Least Squares Estimation
14.2.3 Dishonest Gauss–Newton Method
14.2.4 Difference Between Honest and Dishonest Method
14.2.5 Convergence of Dishonest Method
14.2.6 Graphics Processing Unit
14.3 PERFORMANCE OF DISHONEST GAUSS–NEWTON METHOD
14.3.1 Accuracy of the Estimation
14.3.2 Fast Decoupled State Estimator
14.3.3 Impact of Noise
14.4 GPU IMPLEMENTATION
14.5 SIMULATION RESULTS
14.6 DISCUSSIONS ON SCALABILITY
14.6.1 Estimation of Time for Very Large Systems
14.6.2 Communication Time
14.7 DISTRIBUTED METHOD OF PARALLELIZATION
14.7.1 Cellular Computational Network
14.7.2 Challenges of Cellular Estimation
14.7.3 Structure of the Cellular Dishonest Estimator
14.7.4 Accuracy of Cellular Dishonest Method
14.7.5 Time of Cellular Dishonest Method
14.8 CONCLUSIONS
REFERENCES
INDEX
IEEE Press Series on Power Engineering
WILEY END USER LICENSE AGREEMENT
Отрывок из книги
IEEE Press 445 Hoes Lane Piscataway, NJ 08854
.....
Although problem (2.35) is a mixed integer nonlinear programming problem, the set of constraints (2.35d) can be relaxed:
leading to a relaxed mixed integer nonlinear programming problem. This relaxed problem can be tackled by any nonlinear programming solver (such as MINOS [11]). Numerical simulations show that the particular structure of problem (2.35) imposes that relaxed binary variables bi have a binary value at the optimum, i.e. .
.....