Smart Buildings, Smart Communities and Demand Response
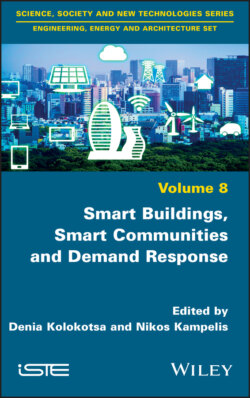
Реклама. ООО «ЛитРес», ИНН: 7719571260.
Оглавление
Группа авторов. Smart Buildings, Smart Communities and Demand Response
Table of Contents
List of Illustrations
List of Tables
Guide
Pages
Smart Buildings, Smart Communities and Demand Response
Preface. Background
Why this book?
Who is this book for?
Structure
Acknowledgments
Nomenclature. Acronyms
Symbols
1. Demand Response in Smart Zero Energy Buildings and Grids. 1.1. Introduction
1.2. Smart and zero energy buildings
1.3. DR and smart grids
1.3.1. DR and congestion management
1.3.2. DR and AS
1.3.3. DR programs
1.3.4. Building level DR
1.3.5. District level DR and microgrids
1.3.6. ANN-based short-term power forecasting
1.4. Scientific focus of the book
1.5. Book outline and objectives
2. DR in Smart and Near-zero Energy Buildings: The Leaf Community
2.1. The Leaf Lab industrial building, AEA Italy
2.2. The Leaf House residential building, AEA Italy
3. Performance of Industrial and Residential Near-zero Energy Buildings
3.1. Materials and methods
3.1.1. Energy simulation model
3.2. Energy performance analysis. 3.2.1. The Leaf Lab
3.2.2. The Leaf House
3.3. Discussion
3.4. Conclusion
4. HVAC Optimization Genetic Algorithm for Industrial Near-Zero Energy Building Demand Response
4.1. Methodology
4.2. GA optimization model
4.3. Model of energy cost
4.4. Results and discussion
4.4.1. Scenario 1: January 25, 2018 (winter)
4.4.2. Scenario 2: March 27, 2018 (spring)
4.4.3. Scenario 3: August 15, 2018 (summer)
4.4.4. Scenario 4: September 10, 2018 (autumn)
4.4.5. Scenario 5: September 21, 2018 (autumn)
4.4.6. Scenario 6: November 20, 2018 (winter)
4.4.7. Scenario 7: November 22, 2018 (winter)
4.4.8. Scenario 8: November 25, 2018 (winter)
4.5. Conclusion and future steps
5. Smart Grid/Community Load Shifting GA Optimization Based on Day-ahead ANN Power Predictions
5.1. Infrastructure and methods
5.2. Day-ahead GA cost of energy/load shifting optimization based on ANN hourly power predictions
5.3. ToU case study. 5.3.1. ANN-based predictions
5.3.2. GA optimization results
5.4. DA real-time case study. 5.4.1. ANN-based predictions
5.4.2. Combined ANN prediction/GA optimization results. 5.4.2.1. DARTP scenario 1: net microgrid level prediction and optimization – 20/3/17
5.4.2.2. DARTP scenario 2: net microgrid level prediction and optimization – 1/8/17
5.4.2.3. DARTP scenario 3a: net microgrid level prediction and optimization – 14/11/17
5.4.2.4. DARTP scenario 3b: net microgrid level prediction and optimization – 14/11/17
5.5. Limitations of the proposed approach
5.6. Conclusion
Conclusions and Recommendations
References
List of Authors
Index. A
B, C
D
E, F
G
H, I
L, M
N
O, P
R
S
T
V
W, Z
WILEY END USER LICENSE AGREEMENT
Отрывок из книги
Engineering, Energy and Architecture Set
.....
Thirdly, the book describes how DR can be applied at the community level by exploiting predictions of day-ahead consumption and/or production and load shifting. The benefits of this approach are evaluated in terms of the economic savings based on a flat versus ToU tariff and an RTP scheme. The reliable prediction of power consumption and/or production 24 hours ahead is performed using artificial neural network modeling, whereas load shifting optimization is conducted using a genetic algorithm dual-objective optimization algorithm.
In Chapter 2, the smart and zero energy building facilities used as case studies for evaluating DR at the building and the community levels are presented.
.....