Intelligent Data Analytics for Terror Threat Prediction
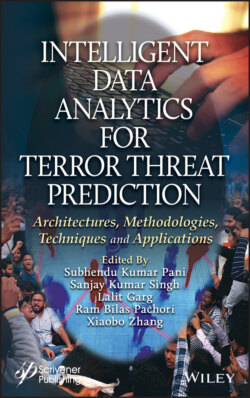
Реклама. ООО «ЛитРес», ИНН: 7719571260.
Оглавление
Группа авторов. Intelligent Data Analytics for Terror Threat Prediction
Table of Contents
List of Tables
List of Illustrations
Guide
Pages
Intelligent Data Analytics for Terror Threat Prediction. Architectures, Methodologies, Techniques and Applications
Preface
1. Rumor Detection and Tracing its Source to Prevent Cyber-Crimes on Social Media
1.1 Introduction
1.2 Social Networks
1.2.1 Types of Social Networks
1.3 What Is Cyber-Crime? 1.3.1 Definition
1.3.2 Types of Cyber-Crimes
1.3.2.1 Hacking
1.3.2.2 Cyber Bullying
1.3.2.3 Buying Illegal Things
1.3.2.4 Posting Videos of Criminal Activity
1.3.3 Cyber-Crimes on Social Networks
1.4 Rumor Detection
1.4.1 Models
1.4.1.1 Naïve Bayes Classifier
1.4.1.2 Support Vector Machine
1.4.1.2.1 Cost Function and Gradient Features
1.4.2 Combating Misinformation on Instagram
1.5 Factors to Detect Rumor Source
1.5.1 Network Structure
1.5.1.1 Network Topology
1.5.1.2 Network Observation
1.5.1.2.1 Complete Observation
1.5.1.2.2 Snapshot Observation
1.5.1.2.3 Monitor Observation
1.5.2 Diffusion Models
1.5.2.1 SI Model
1.5.2.2 SIS Model
1.5.2.3 SIR Model
1.5.2.4 SIRS Model
1.5.3 Centrality Measures
1.5.3.1 Degree Centrality
1.5.3.2 Closeness Centrality
1.5.3.3 Betweenness Centrality
1.6 Source Detection in Network
1.6.1 Single Source Detection
1.6.1.1 Network Observation
1.6.1.1.1 Complete Observation
1.6.1.1.2 Snapshot Observation
1.6.1.1.3 Monitor Observation
1.6.1.2 Query-Based Approach
1.6.1.3 Anti-Rumor-Based Approach
1.6.2 Multiple Source Detection
1.7 Conclusion
References
2. Internet of Things (IoT) and Machine to Machine (M2M) Communication Techniques for Cyber Crime Prediction
2.1 Introduction
2.2 Advancement of Internet
2.3 Internet of Things (IoT) and Machine to Machine (M2M) Communication
2.4 A Definition of Security Frameworks
2.5 M2M Devices and Smartphone Technology
2.6 Explicit Hazards to M2M Devices Declared by Smartphone Challenges
2.7 Security and Privacy Issues in IoT
2.7.1 Dynamicity and Heterogeneity
2.7.2 Security for Integrated Operational World with Digital World
2.7.3 Information Safety with Equipment Security
2.7.4 Data Source Information
2.7.5 Information Confidentiality
2.7.6 Trust Arrangement
2.8 Protection in Machine to Machine Communication
2.9 Use Cases for M2M Portability
2.10 Conclusion
References
3. Crime Predictive Model Using Big Data Analytics
3.1 Introduction
3.1.1 Geographic Information System (GIS)
3.2 Crime Data Mining
3.2.1 Different Methods for Crime Data Analysis
3.3 Visual Data Analysis
3.4 Technological Analysis. 3.4.1 Hadoop and MapReduce
3.4.1.1 Hadoop Distributed File System (HDFS)
3.4.1.2 MapReduce
3.4.1.2.1 MapReduce-Based Data Analytic
3.4.1.2.2 Work Style of MapReduce
3.4.2 Hive
3.4.2.1 Analysis of Crime Data using Hive
3.4.2.2 Data Analytic Module With Hive
3.4.3 Sqoop
3.4.3.1 Pre-Processing and Sqoop
3.4.3.2 Data Migration Module With Sqoop
3.4.3.3 Partitioning
3.4.3.4 Bucketing
3.4.3.5 R-Tool Analyse Crime Data
3.4.3.6 Correlation Matrix
3.5 Big Data Framework
3.6 Architecture for Crime Technical Model
3.7 Challenges
3.8 Conclusions
References
4. The Role of Remote Sensing and GIS in Military Strategy to Prevent Terror Attacks
4.1 Introduction
4.2 Database and Methods
4.3 Discussion and Analysis
4.4 Role of Remote Sensing and GIS
4.5 Cartographic Model
4.5.1 Spatial Data Management
4.5.2 Battlefield Management
4.5.3 Terrain Analysis
4.6 Mapping Techniques Used for Defense Purposes
4.7 Naval Operations
4.7.1 Air Operations
4.7.2 GIS Potential in Military
4.8 Future Sphere of GIS in Military Science
4.8.1 Defense Site Management
4.8.2 Spatial Data Management
4.8.3 Intelligence Capability Approach
4.8.4 Data Converts Into Information
4.8.5 Defense Estate Management
4.9 Terrain Evolution
4.9.1 Problems Regarding the Uses of Remote Sensing and GIS
4.9.2 Recommendations
4.10 Conclusion
References
5. Text Mining for Secure Cyber Space
5.1 Introduction
5.2 Literature Review
5.2.1 Text Mining With Latent Semantic Analysis
5.3 Latent Semantic Analysis
5.4 Proposed Work
5.5 Detailed Work Flow of Proposed Approach
5.5.1 Defining the Stop Words
5.5.2 Stemming
5.5.3 Proposed Algorithm: A Hybrid Approach
5.6 Results and Discussion
5.6.1 Analysis Using Hybrid Approach
5.7 Conclusion
References
6. Analyses on Artificial Intelligence Framework to Detect Crime Pattern
6.1 Introduction
6.2 Related Works
6.3 Proposed Clustering for Detecting Crimes
6.3.1 Data Pre-Processing
6.3.2 Object-Oriented Model
6.3.3 MCML Classification
6.3.4 GAA
6.3.5 Consensus Clustering
6.4 Performance Evaluation
6.4.1 Precision
6.4.2 Sensitivity
6.4.3 Specificity
6.4.4 Accuracy
6.5 Conclusions
References
7. A Biometric Technology-Based Framework for Tackling and Preventing Crimes
7.1 Introduction
7.2 Biometrics
7.2.1 Biometric Systems Technologies
7.2.2 Biometric Recognition Framework
7.2.3 Biometric Applications/Usages
7.3 Surveillance Systems (CCTV)
7.3.1 CCTV Goals
7.3.2 CCTV Processes
7.3.3 Fusion of Data From Multiple Cameras
7.3.4 Expanding the Use of CCTV
7.3.5 CCTV Effectiveness
7.3.6 CCTV Limitations
7.3.7 Privacy and CCTV
7.4 Legality to Surveillance and Biometrics vs. Privacy and Human Rights
7.5 Proposed Work (Biometric-Based CCTV System)
7.5.1 Biometric Surveillance System. 7.5.1.1 System Component and Flow Diagram
7.5.2 Framework
7.6 Conclusion
References
8. Rule-Based Approach for Botnet Behavior Analysis
8.1 Introduction
8.2 State-of-the-Art
8.3 Bots and Botnets
8.3.1 Botnet Life Cycle
8.3.2 Botnet Detection Techniques
8.3.3 Communication Architecture
8.4 Methodology
8.5 Results and Analysis
8.6 Conclusion and Future Scope
References
9. Securing Biometric Framework with Cryptanalysis
9.1 Introduction
9.2 Basics of Biometric Systems
9.2.1 Face
9.2.2 Hand Geometry
9.2.3 Fingerprint
9.2.4 Voice Detection
9.2.5 Iris
9.2.6 Signature
9.2.7 Keystrokes
9.3 Biometric Variance
9.3.1 Inconsistent Presentation
9.3.2 Unreproducible Presentation
9.3.3 Fault Signal/Representational Accession
9.4 Performance of Biometric System
9.5 Justification of Biometric System
9.5.1 Authentication (“Is this individual really the authenticate user or not?”)
9.5.2 Recognition (“Is this individual in the database?”)
9.5.3 Concealing (“Is this a needed person?”)
9.6 Assaults on a Biometric System
9.6.1 Zero Effort Attacks
9.6.2 Adversary Attacks
9.6.2.1 Circumvention
9.6.2.2 Coercion
9.6.2.3 Repudiation
9.6.2.4 DoB (Denial of Benefit)
9.6.2.5 Collusion
9.7 Biometric Cryptanalysis: The Fuzzy Vault Scheme
9.8 Conclusion & Future Work
References
10. The Role of Big Data Analysis in Increasing the Crime Prediction and Prevention Rates
10.1 Introduction: An Overview of Big Data and Cyber Crime
10.2 Techniques for the Analysis of BigData
10.3 Important Big Data Security Techniques
10.4 Conclusion
References
11. Crime Pattern Detection Using Data Mining
11.1 Introduction
11.2 Related Work
11.3 Methods and Procedures
11.4 System Analysis
11.5 Analysis Model and Architectural Design
11.6 Several Criminal Analysis Methods in Use
11.7 Conclusion and Future Work
References
12. Attacks and Security Measures in Wireless Sensor Network
12.1 Introduction
12.2 Layered Architecture of WSN
12.2.1 Physical Layer
12.2.2 Data Link Layer
12.2.3 Network Layer
12.2.4 Transport Layer
12.2.5 Application Layer
12.3 Security Threats on Different Layers in WSN
12.3.1 Threats on Physical Layer
12.3.1.1 Eavesdropping Attack
12.3.1.2 Jamming Attack
12.3.1.3 Imperil or Compromised Node Attack
12.3.1.4 Replication Node Attack
12.3.2 Threats on Data Link Layer
12.3.2.1 Collision Attack
12.3.2.2 Denial of Service (DoS) Attack
12.3.2.3 Intelligent Jamming Attack
12.3.3 Threats on Network Layer
12.3.3.1 Sybil Attack
12.3.3.2 Gray Hole Attack
12.3.3.3 Sink Hole Attack
12.3.3.4 Hello Flooding Attack
12.3.3.5 Spoofing Attack
12.3.3.6 Replay Attack
12.3.3.7 Black Hole Attack
12.3.3.8 Worm Hole Attack
12.3.4 Threats on Transport Layer
12.3.4.1 De-Synchronization Attack
12.3.4.2 Flooding Attack
12.3.5 Threats on Application Layer
12.3.5.1 Malicious Code Attack
12.3.5.2 Attack on Reliability
12.3.6 Threats on Multiple Layer
12.3.6.1 Man-in-the-Middle Attack
12.3.6.2 Jamming Attack
12.3.6.3 Dos Attack
12.4 Threats Detection at Various Layers in WSN
12.4.1 Threat Detection on Physical Layer
12.4.1.1 Compromised Node Attack
12.4.1.2 Replication Node Attack
12.4.2 Threat Detection on Data Link Layer
12.4.2.1 Denial of Service Attack
12.4.3 Threat Detection on Network Layer
12.4.3.1 Black Hole Attack
12.4.3.2 Worm Hole Attack
12.4.3.3 Hello Flooding Attack
12.4.3.4 Sybil Attack
12.4.3.5 Gray Hole Attack
12.4.3.6 Sink Hole Attack
12.4.4 Threat Detection on the Transport Layer
12.4.4.1 Flooding Attack
12.4.5 Threat Detection on Multiple Layers
12.4.5.1 Jamming Attack
12.5 Various Parameters for Security Data Collection in WSN
12.5.1 Parameters for Security of Information Collection
12.5.1.1 Information Grade
12.5.1.2 Efficacy and Proficiency
12.5.1.3 Reliability Properties
12.5.1.4 Information Fidelity
12.5.1.5 Information Isolation
12.5.2 Attack Detection Standards in WSN
12.5.2.1 Precision
12.5.2.2 Germane
12.5.2.3 Extensibility
12.5.2.4 Identifiability
12.5.2.5 Fault Forbearance
12.6 Different Security Schemes in WSN
12.6.1 Clustering-Based Scheme
12.6.2 Cryptography-Based Scheme
12.6.3 Cross-Checking-Based Scheme
12.6.4 Overhearing-Based Scheme
12.6.5 Acknowledgement-Based Scheme
12.6.6 Trust-Based Scheme
12.6.7 Sequence Number Threshold-Based Scheme
12.6.8 Intrusion Detection System-Based Scheme
12.6.9 Cross-Layer Collaboration-Based Scheme
12.7 Conclusion
References
13. Large Sensing Data Flows Using Cryptic Techniques
13.1 Introduction
13.2 Data Flow Management. 13.2.1 Data Flow Processing
13.2.2 Stream Security
13.2.3 Data Privacy and Data Reliability
13.2.3.1 Security Protocol
13.3 Design of Big Data Stream. 13.3.1 Data Stream System Architecture
13.3.1.1 Intrusion Detection Systems (IDS)
13.3.2 Malicious Model
13.3.3 Threat Approaches for Attack Models
13.4 Utilization of Security Methods
13.4.1 System Setup
13.4.2 Re-Keying
13.4.3 New Node Authentication
13.4.4 Cryptic Techniques
13.5 Analysis of Security on Attack
13.6 Artificial Intelligence Techniques for Cyber Crimes
13.6.1 Cyber Crime Activities
13.6.2 Artificial Intelligence for Intrusion Detection
13.6.3 Features of an IDPS
13.7 Conclusions
References
14. Cyber-Crime Prevention Methodology
14.1 Introduction
14.1.1 Evolution of Cyber Crime
14.1.2 Cybercrime can be Broadly Defined as Two Types
14.1.3 Potential Vulnerable Sectors of Cybercrime
14.2 Credit Card Frauds and Skimming
14.2.1 Matrimony Fraud
14.2.2 Juice Jacking
14.2.3 Technicality Behind Juice Jacking
14.3 Hacking Over Public WiFi or the MITM Attacks
14.3.1 Phishing
14.3.2 Vishing/Smishing
14.3.3 Session Hijacking
14.3.4 Weak Session Token Generation/Predictable Session Token Generation
14.3.5 IP Spoofing
14.3.6 Cross-Site Scripting (XSS) Attack
14.4 SQLi Injection
14.5 Denial of Service Attack
14.6 Dark Web and Deep Web Technologies
14.6.1 The Deep Web
14.6.2 The Dark Web
14.7 Conclusion
References
Index
WILEY END USER LICENSE AGREEMENT
Отрывок из книги
Scrivener Publishing
.....
In social networks, once rumor is diffused and received by any user he/she becomes infectious if doesn’t know truth about rumor. If they knew truth, he/she recover by ignoring rumor or not passing to neighbors. This is ignored in SI and SIS models. Recovery from rumors is only between SIR and SIS models. Figure 1.10 shows how users are transforming from one state to other.
In SIR model once a person recovered from disease he/she remains in same state in future. In general once a person is cured from any disease there is chance that they may be reinfected with same decease in future, which is ignored in SIR model. SIRS model addresses this problem where once a person is infected and have recovered by having immunity or medical treatment, they couldn’t be in same recovered state in future. After recovery, there is possibility that again infected by same decease [16].
.....