Administrative Records for Survey Methodology
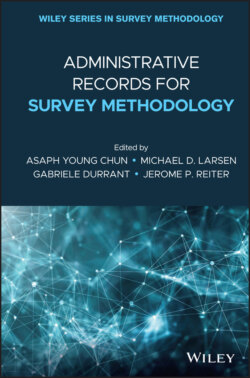
Реклама. ООО «ЛитРес», ИНН: 7719571260.
Оглавление
Группа авторов. Administrative Records for Survey Methodology
Table of Contents
List of Figures
List of Tables
Guide
Pages
WILEY SERIES IN SURVEY METHODOLOGY
Administrative Records for Survey Methodology
Preface
References
Acknowledgments
References
List of Contributors
1 On the Use of Proxy Variables in Combining Register and Survey Data
1.1 Introduction
1.1.1 A Multisource Data Perspective
1.1.2 Concept of Proxy Variable
1.2 Instances of Proxy Variable
1.2.1 Representation
1.2.2 Measurement
1.3 Estimation Using Multiple Proxy Variables
1.3.1 Asymmetric Setting
1.3.2 Uncertainty Evaluation: A Case of Two-Way Data
1.3.3 Symmetric Setting
1.4 Summary
References
2 Disclosure Limitation and Confidentiality Protection in Linked Data
2.1 Introduction
2.2 Paradigms of Protection
2.2.1 Input Noise Infusion
2.2.2 Formal Privacy Models
2.3 Confidentiality Protection in Linked Data: Examples
2.3.1 HRS–SSA. 2.3.1.1 Data Description
2.3.1.2 Linkages to Other Data
2.3.1.3 Disclosure Avoidance Methods
2.3.2 SIPP–SSA–IRS (SSB) 2.3.2.1 Data Description
2.3.2.2 Disclosure Avoidance Methods
2.3.2.3 Disclosure Avoidance Assessment
2.3.2.4 Analytical Validity Assessment
Box 2.1 Sidebox: Practical Synthetic Data Use
2.3.3 LEHD: Linked Establishment and Employee Records. 2.3.3.1 Data Description
2.3.3.2 Disclosure Avoidance Methods
2.3.3.3 Disclosure Avoidance Assessment for QWI
2.3.3.4 Analytical Validity Assessment for QWI
Time-Series Properties of Distorted Data
Cross-sectional Unbiasedness of the Distorted Data
Box 2.2 Sidebox: Do-It-Yourself Noise Infusion
2.4 Physical and Legal Protections
2.4.1 Statistical Data Enclaves
2.4.2 Remote Processing
2.4.3 Licensing
2.4.4 Disclosure Avoidance Methods
2.4.5 Data Silos
2.5 Conclusions
2.A Appendix: Technical Terms and Acronyms
2.A.1 Other Abbreviations
2.A.2 Concepts
Acknowledgments
References
Notes
3 Evaluation of the Quality of Administrative Data Used in the Dutch Virtual Census
3.1 Introduction
3.2 Data Sources and Variables
3.3 Quality Framework
3.3.1 Source and Metadata Hyper Dimensions
3.3.2 Data Hyper Dimension
3.4 Quality Evaluation Results for the Dutch 2011 Census
3.4.1 Source and Metadata: Application of Checklist
3.4.2 Data Hyper Dimension: Completeness and Accuracy Results
3.4.2.1 Completeness Dimension
3.4.2.2 Accuracy Dimension
3.4.2.3 Visualizing with a Tableplot
3.4.3 Discussion of the Quality Findings
3.5 Summary
3.6 Practical Implications for Implementation with Surveys and Censuses
3.7 Exercises
References
4 Improving Input Data Quality in Register-Based Statistics: The Norwegian Experience
4.1 Introduction
4.2 The Use of Administrative Sources in Statistics Norway
4.3 Managing Statistical Populations
4.4 Experiences from the First Norwegian Purely Register-Based Population and Housing Census of 2011
4.5 The Contact with the Owners of Administrative Registers Was Put into System
4.5.1 Agreements on Data Processing
4.5.2 Agreements of Cooperation on Data Quality in Administrative Data Systems
4.5.3 The Forums for Cooperation
4.6 Measuring and Documenting Input Data Quality. 4.6.1 Quality Indicators
4.6.2 Operationalizing the Quality Checks
4.6.3 Quality Reports
4.6.4 The Approach Is Being Adopted by the Owners of Administrative Data
4.7 Summary
4.8 Exercises
4.A Example of a Quality Report for Registered Persons in the Central Population Register
References
Notes
5 Cleaning and Using Administrative Lists: Enhanced Practices and Computational Algorithms for Record Linkage and Modeling/Editing/Imputation
5.1 Introductory Comments
5.1.1 Example 1
5.1.2 Example 2
5.1.3 Example 3
5.2 Edit/Imputation
5.2.1 Background
5.2.2 Fellegi–Holt Model
5.2.3 Imputation Generalizing Little–Rubin
5.2.4 Connecting Edit with Imputation
5.2.5 Achieving Extreme Computational Speed
5.3 Record Linkage
5.3.1 Fellegi–Sunter Model
5.3.2 Estimating Parameters
5.3.3 Estimating False Match Rates
5.3.3.1 The Data Files
5.3.4 Achieving Extreme Computational Speed
5.4 Models for Adjusting Statistical Analyses for Linkage Error. 5.4.1 Scheuren–Winkler
5.4.2 Lahiri–Larsen
5.4.3 Chambers and Kim
5.4.4 Chipperfield, Bishop, and Campbell
5.4.4.1 Empirical Data
5.4.5 Goldstein, Harron, and Wade
5.4.6 Hof and Zwinderman
5.4.7 Tancredi and Liseo
5.5 Concluding Remarks
5.6 Issues and Some Related Questions
References
6 Assessing Uncertainty When Using Linked Administrative Records
6.1 Introduction
6.2 General Sources of Uncertainty
6.2.1 Imperfect Matching
6.2.2 Incomplete Matching
6.3 Approaches to Accounting for Uncertainty
6.3.1 Modeling Matching Matrix as Parameter
6.3.2 Direct Modeling
6.3.3 Imputation of Entire Concatenated File
6.4 Concluding Remarks. 6.4.1 Problems to Be Solved
6.4.2 Practical Implications
6.5 Exercises
Acknowledgment
References
7 Measuring and Controlling for Non-Consent Bias in Linked Survey and Administrative Data
7.1 Introduction. 7.1.1 What Is Linkage Consent? Why Is Linkage Consent Needed?
7.1.2 Linkage Consent Rates in Large-Scale Surveys
7.1.3 The Impact of Linkage Non-Consent Bias on Survey Inference
7.1.4 The Challenge of Measuring and Controlling for Linkage Non-Consent Bias
7.2 Strategies for Measuring Linkage Non-Consent Bias. 7.2.1 Formulation of Linkage Non-Consent Bias
7.2.2 Modeling Non-Consent Using Survey Information
7.2.3 Analyzing Non-Consent Bias for Administrative Variables
7.3 Methods for Minimizing Non-Consent Bias at the Survey Design Stage
7.3.1 Optimizing Linkage Consent Rates
7.3.2 Placement of the Consent Request
7.3.3 Wording of the Consent Request
7.3.4 Active and Passive Consent Procedures
7.3.5 Linkage Consent in Panel Studies
7.4 Methods for Minimizing Non-Consent Bias at the Survey Analysis Stage
7.4.1 Controlling for Linkage Non-Consent Bias via Statistical Adjustment
7.4.2 Weighting Adjustments
7.4.3 Imputation
7.5 Summary
7.5.1 Key Points for Measuring Linkage Non-Consent Bias
7.5.2 Key Points for Controlling for Linkage Non-Consent Bias
7.6 Practical Implications for Implementation with Surveys and Censuses
7.7 Exercises
References
8 A Register-Based Census: The Swedish Experience
8.1 Introduction
8.2 Background
8.3 Census 2011
8.4 A Register-Based Census
8.4.1 Registers at Statistics Sweden
8.4.2 Facilitating a System of Registers
8.4.3 Introducing a Dwelling Identification Key
8.4.4 The Census Household and Dwelling Populations
8.5 Evaluation of the Census. 8.5.1 Introduction
8.5.2 Evaluating Household Size and Type
8.5.2.1 Sampling Design
8.5.2.2 Data Collection
8.5.2.3 Reconciliation
8.5.2.4 Results
8.5.3 Evaluating Ownership
8.5.4 Lessons Learned
8.6 Impact on Population and Housing Statistics
8.7 Summary and Final Remarks
References
Notes
9 Administrative Records Applications for the 2020 Census
9.1 Introduction
9.2 Administrative Record Usage in the U.S. Census
9.3 Administrative Record Integration in 2020 Census Research
9.3.1 Administrative Record Usage Determinations
9.3.2 NRFU Design Incorporating Administrative Records
9.3.3 Administrative Records Sources and Data Preparation
9.3.4 Approach to Determine Administrative Record Vacant Addresses
9.3.5 Extension of Vacant Methodology to Nonexistent Cases
9.3.6 Approach to Determine Occupied Addresses
9.3.7 Other Aspects and Alternatives of Administrative Record Enumeration
9.4 Quality Assessment
9.4.1 Microlevel Evaluations of Quality
9.4.2 Macrolevel Evaluations of Quality
9.5 Other Applications of Administrative Record Usage
9.5.1 Register-Based Census
9.5.2 Supplement Traditional Enumeration with Adjustments for Estimated Error for Official Census Counts
9.5.3 Coverage Evaluation
9.6 Summary
9.7 Exercises
References
Note
10 Use of Administrative Records in Small Area Estimation
10.1 Introduction
10.2 Data Preparation
10.3 Small Area Estimation Models for Combining Information
10.3.1 Area-level Models
10.3.2 Unit-level Models
10.4 An Application
10.5 Concluding Remarks
10.6 Exercises
Acknowledgments
References
11 Enhancement of Health Surveys with Data Linkage
11.1 Introduction. 11.1.1 The National Center for Health Statistics (NCHS)
11.1.2 The NCHS Data Linkage Program
11.1.3 Initial Linkages with NCHS Surveys
11.2 Examples of NCHS Health Surveys that Were Enhanced Through Linkage
11.2.1 National Health Interview Survey (NHIS)
11.2.2 National Health and Nutrition Examination Survey (NHANES)
11.2.3 National Health Care Surveys
11.3 NCHS Health Surveys Linked with Vital Records and Administrative Data
11.3.1 National Death Index (NDI)
11.3.2 Centers for Medicare and Medicaid Services (CMS)
11.3.3 Social Security Administration (SSA)
11.3.4 Department of Housing and Urban Development (HUD)
11.3.5 United States Renal Data System and the Florida Cancer Data System
11.4 NCHS Data Linkage Program: Linkage Methodology and Processing Issues
11.4.1 Informed Consent in Health Surveys
11.4.2 Informed Consent for Child Survey Participants
11.4.3 Adaptive Approaches to Linking Health Surveys with Administrative Data
11.4.4 Use of Alternate Records
11.4.5 Protecting the Privacy of Health Survey Participants and Maintaining Data Confidentiality
11.4.6 Updates Over Time
11.5 Enhancements to Health Survey Data Through Linkage
11.6 Analytic Considerations and Limitations of Administrative Data
11.6.1 Adjusting Sample Weights for Linkage-Eligibility
11.6.2 Residential Mobility and Linkages to State Programs and Registries
11.7 Future of the NCHS Data Linkage Program
11.8 Exercises
Acknowledgments
Disclaimer
References
Note
12 Combining Administrative and Survey Data to Improve Income Measurement
12.1 Introduction
12.2 Measuring and Decomposing Total Survey Error
12.3 Generalized Coverage Error
12.4 Item Nonresponse and Imputation Error
12.5 Measurement Error
12.6 Illustration: Using Data Linkage to Better Measure Income and Poverty
12.7 Accuracy of Links and the Administrative Data
12.8 Conclusions
12.9 Exercises
Acknowledgments
References
13 Combining Data from Multiple Sources to Define a Respondent: The Case of Education Data
13.1 Introduction
13.1.1 Options for Defining a Unit Respondent When Data Exist from Sources Instead of or in Addition to an Interview
13.1.2 Concerns with Defining a Unit Respondent Without Having an Interview
13.2 Literature Review
13.3 Methodology
13.3.1 Computing Weights for Interview Respondents and for Unit Respondents Who May Not Have Interview Data (Usable Case Respondents)
13.3.1.1 How Many Weights Are Necessary?
13.3.2 Imputing Data When All or Some Interview Data Are Missing
13.3.3 Conducting Nonresponse Bias Analyses to Appropriately Consider Interview and Study Nonresponse
13.4 Example of Defining a Unit Respondent for the National Postsecondary Student Aid Study (NPSAS) 13.4.1 Overview of NPSAS
13.4.2 Usable Case Respondent Approach
13.4.2.1 Results
13.4.3 Interview Respondent Approach
13.4.3.1 Results
13.4.4 Comparison of Estimates, Variances, and Nonresponse Bias Using Two Approaches to Define a Unit Respondent
13.5 Discussion: Advantages and Disadvantages of Two Approaches to Defining a Unit Respondent
13.5.1 Interview Respondents
13.5.2 Usable Case Respondents
13.6 Practical Implications for Implementation with Surveys and Censuses
13.A Appendix. 13.A.1 NPSAS:08 Study Respondent Definition
13.B Appendix
References
Note
Index
WILEY END USER LICENSE AGREEMENT
Отрывок из книги
Established in Part by Walter A. Shewhart and Samuel S. Wilks
Editors: Mick P. Couper, Graham Kalton, J. N. K. Rao, Norbert Schwarz, Christopher Skinner, Lars Lyberg
.....
This is problematic when there are empty and very small sample cells of (a, j). Raking ratio weight can then be given by , where is derived by the IPF of to row and column totals Xa+ and X+j, respectively. Deville, Särndal, and Sautory (1993) provide approximate variance of the raking ratio estimator, say, where
A drawback of the weighting approach above is that no estimate of Yaj will be available in the case of empty sample cell (a, j), and the estimate will have a large sampling variance when the sample cell (a, j) is small in size. This is typically the situation in small area estimation, where, e.g. a is the index of a large number of local areas. Zhang and Chambers (2004) and Luna-Hernández (2016) develop prediction modeling approach.
.....