Agricultural Informatics
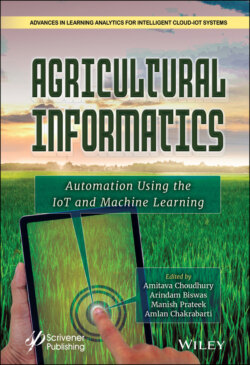
Реклама. ООО «ЛитРес», ИНН: 7719571260.
Оглавление
Группа авторов. Agricultural Informatics
Table of Contents
Guide
List of Illustrations
List of Tables
Pages
Agricultural Informatics. Automation Using the IoT and Machine Learning
Preface
1. A Study on Various Machine Learning Algorithms and Their Role in Agriculture
1.1 Introduction
1.1.1 Machine Learning Model. 1.1.1.1 Artificial Neural Networks
1.1.1.2 Information Fuzzy Network
1.1.1.3 Decision Trees
1.1.1.4 Regression Analysis
1.1.1.5 Principal Component Analysis
1.1.1.6 Bayesian Networks
1.1.1.7 Time Series Analysis
1.1.1.8 Markov Chain Model
1.2 Conclusions
References
2. Smart Farming Using Machine Learning and IoT
2.1 Introduction
2.1.1 Smart Farming
2.1.2 Technology Involvement in Smart Agriculture
2.2 Related Work
2.2.1 Monitoring Soil, Climate and Crop
2.2.2 Pesticide Control
2.2.3 Proper Fertilizer Uses
2.2.4 Intrusion Detection
2.2.5 Weed Control
2.2.6 Water Supply Management
2.3 Problem Identification
2.4 Objective Behind the Integrated Agro-IoT System
2.5 Proposed Prototype of the Integrated Agro-IoT System
2.5.1 Pest or Weed Detection Process
2.5.2 Fire Detection Process
2.6 Hardware Component Requirement for the Integrated Agro-IoT System. 2.6.1 Sensors
2.6.2 Camera
2.6.3 Water Pump
2.6.4 Relay
2.6.5 Water Reservoir
2.6.6 Solar Panel
2.6.7 GSM Module
2.6.8 Iron Railing
2.6.9 Beaglebone Black
2.7 Comparative Study Between Raspberry Pi vs Beaglebone Black. 2.7.1 Raw Comparison
2.7.2 Ease of Setup
2.7.3 Connections
2.7.4 Processor Showdown
2.7.5 Right Choice for Projects
2.8 Conclusions
2.9 Future Work
References
3. Agricultural Informatics vis-à-vis Internet of Things (IoT): The Scenario, Applications and Academic Aspects — International Trend & Indian Possibilities
3.1 Introduction
3.2 Objectives
3.3 Methods
3.4 Agricultural Informatics: An Account
3.4.1 Agricultural Informatics and Environmental Informatics
3.4.2 Stakeholders of Agricultural Informatics
3.4.2.1 Technology
3.4.2.2 Digital Content
3.4.2.3 Agricultural Components
3.4.2.4 Peoples
3.5 Agricultural Informatics & Technological Components: Basics & Emergence
3.6 IoT: Basics and Characteristics
3.7 IoT: The Applications & Agriculture Areas
3.8 Agricultural Informatics & IoT: The Scenario
3.8.1 Weather, Climate & Agro IoT
3.8.2 Precision Cultivation With Agro IoT
3.8.3 IoT in Making Green House Perfect
3.8.4 Data Analytics and Management by IoT in Agro Space
3.8.5 Drone, IoT and Agriculture
3.8.6 Livestock Management Using AIoT
3.8.7 Environmental Monitoring & IoT, Environmental Informatics
3.9 IoT in Agriculture: Requirement, Issues & Challenges
3.10 Development, Economy and Growth: Agricultural Informatics Context
3.11 Academic Availability and Potentiality of IoT in Agricultural Informatics: International Scenario & Indian Possibilities
3.12 Suggestions
3.13 Conclusion
References
4. Application of Agricultural Drones and IoT to Understand Food Supply Chain During Post COVID-19
4.1 Introduction
4.2 Related Work
4.3 Smart Production With the Introduction of Drones and IoT
4.3.1 Real-Time Surveyed Data Collection and Storage Utilizing an IoT System. 4.3.1.1 Efficient Control of Distributed Networks of Services (Using the Integrated Networks Sensors Provided)
4.3.1.2 Human Participation in Surveillance and Monitoring can be Identified to Take the Following Roles
4.4 Agricultural Drones
4.5 IoT Acts as a Backbone in Addressing COVID-19 Problems in Agriculture
4.5.1 Implementation in Agriculture—Drones
4.5.2 Communication and Networking Mechanisms
4.5.3 Managing Agricultural Data Safety and Security of Individual Farmers
4.6 Conclusion
References
5. IoT and Machine Learning-Based Approaches for Real Time Environment Parameters Monitoring in Agriculture: An Empirical Review
5.1 Introduction
5.2 Machine Learning (ML)-Based IoT Solution
5.3 Motivation of the Work
5.4 Literature Review of IoT-Based Weather and Irrigation Monitoring for Precision Agriculture
5.5 Literature Review of Machine Learning-Based Weather and Irrigation Monitoring for Precision Agriculture
5.6 Challenges
5.7 Conclusion and Future Work
References
6. Deep Neural Network-Based Multi-Class Image Classification for Plant Diseases
6.1 Introduction
6.2 Related Work
6.3 Proposed Work
6.3.1 Dataset Description
6.3.2 Data Pre-Processing and Augmentation
6.3.3 CNN Architecture
6.4 Results and Evaluation
6.5 Conclusion
References
7. Deep Residual Neural Network for Plant Seedling Image Classification
7.1 Introduction
7.1.1 Architecture of CNN
7.1.1.1 Principles of ConvNet
7.1.2 Residual Network (ResNet)
7.2 Related Work
7.3 Proposed Work
7.3.1 Data Collection
7.3.2 Data Pre-Processing
7.3.3 Data Annotation and Augmentation
7.3.4 Training and Fine-Tuning
7.4 Result and Evaluation
7.4.1 Metrics
7.4.2 Result Analysis. 7.4.2.1 Experiment I
7.4.2.2 Experiment II
7.5 Conclusion
References
8. Development of IoT-Based Smart Security and Monitoring Devices for Agriculture
8.1 Introduction
8.2 Background & Related Works
8.3 Proposed Model
8.3.1 Raspberry Pi 4 Model B
8.3.2 Passive Infrared Sensor (PIR Sensor)
8.3.3 pH Sensor
8.3.4 Dielectric Soil Moisture Sensor
8.3.5 RGB-D Sensor
8.3.6 GSM Module
8.3.7 Unmanned Aerial Vehicle (UAV)
8.4 Methodology
8.5 Performance Analysis
8.6 Future Research Direction
8.7 Conclusion
References
9. An Integrated Application of IoT-Based WSN in the Field of Indian Agriculture System Using Hybrid Optimization Technique and Machine Learning
9.1 Introduction
9.1.1 Contribution in Detail
9.2 Literature Review
9.3 Proposed Hybrid Algorithms (GA-MWPSO)
9.4 Reliability Optimization and Coverage Optimization Model
9.5 Problem Description
9.6 Numerical Examples, Results and Discussion. 9.6.1 Case Example
9.6.2 Theoretical Approach to Make Machine Learning Model
9.7 Conclusion
References
10. Decryption and Design of a Multicopter Unmanned Aerial Vehicle (UAV) for Heavy Lift Agricultural Operations
10.1 Introduction
10.1.1 Classification of Small UAVs
10.2 History of Multicopter UAVs
10.3 Basic Components of Multicopter UAV
10.3.1 Airframe
10.3.1.1 Fuselage
10.3.1.2 Landing Gear
10.3.1.3 Arms
10.3.1.4 Selection of Material for UAV Airframe
10.3.2 Propulsion System
10.3.2.1 Lithium Polymer (LiPo) Battery
10.3.2.2 Propeller
10.3.2.3 Brushless DC (BLDC) Motor
10.3.2.4 Electronic Speed Controller (ESC)
10.3.3 Command and Control System. 10.3.3.1 Remote Controlled (RC) Transmitter and Receiver
10.3.3.2 Flight Controller Unit (FCU)
10.3.3.3 Ground Control Station (GCS)
10.3.3.4 Radio Telemetry
10.3.3.5 Global Positioning System (GPS)
10.4 Working and Control Mechanism of Multicopter UAV
10.4.1 Upward and Downward Movement
10.4.2 Forward and Backward Movement
10.4.3 Leftward-and-Rightward Movement
10.4.4 Yaw Movement
10.5 Design Calculations and Selection of Components
10.5.1 Fuselage Configuration
10.5.2 Propeller Selection
10.5.3 Motor Selection
10.5.4 Maximum Power and Current Requirement
10.5.5 Thrust Requirement by Motor [32]
10.5.6 Thrust Requirement by the Propeller
10.5.7 Endurance or Flight Time
10.5.8 Maximum Airframe Size
10.6 Conclusion
References
11. IoT-Enabled Agricultural System Application, Challenges and Security Issues
11.1 Introduction
11.2 Background & Related Works
11.3 Challenges to Implement IoT-Enabled Systems
11.3.1 Secured Data Generation and Transmission and Privacy
11.3.2 Lack of Supporting Infrastructure
11.3.3 Technical Skill Requirement
11.3.4 Complexity in Software and Hardware
11.3.5 Bulk Data
11.3.6 Disrupted Connectivity to the Cloud
11.3.7 Better Connectivity
11.3.8 Interoperability Issue
11.3.9 Crop Management Issues
11.3.10 Power Consumption
11.3.11 Environmental Challenges
11.3.12 High Cost
11.4 Security Issues and Measures
11.5 Future Research Direction
11.6 Conclusion
References
12. Plane Region Step Farming, Animal and Pest Attack Control Using Internet of Things
12.1 Introduction
12.1.1 Possible Various Applications in Agriculture
12.1.2 Cayenne IoT Builder
12.2 Proposed Work. 12.2.1 Design of Agro Farm Structure
12.3 Irrigation Methodology
12.3.1 Irrigation Scheduling
12.3.2 Two Critical Circumstances Farmers Often Face
12.3.3 Irrigation Indices
12.4 Sensor Connection Using Internet of Things
12.4.1 Animal Attack Control
12.4.2 Pest Attack Control
12.4.3 DHT 11 Humidity & Temperature Sensor
12.4.4 Rain Sensor Module
12.4.5 Soil Moisture Sensor
12.5 Placement of Sensor in the Field
12.6 Conclusion
References
Index
WILEY END USER LICENSE AGREEMENT
Отрывок из книги
Scrivener Publishing 100 Cummings Center, Suite 541J Beverly, MA 01915-6106
.....
19. Basso, B., Cammarano, D., Carfagna, E., Review of Crop Yield Forecasting Methods and Early Warning Systems. First Meet. Sci. Advis. Comm. Glob. Strateg. to Improv. Agric. Rural Stat, 2013.
20. De La Rosa, D., Cardona, F., Almorza, J., Crop yield predictions based on properties of soils in Sevilla, Spain. Geoderma, 25, 3–4, 267–274, May, 1981.
.....