Mechanical Engineering in Uncertainties From Classical Approaches to Some Recent Developments
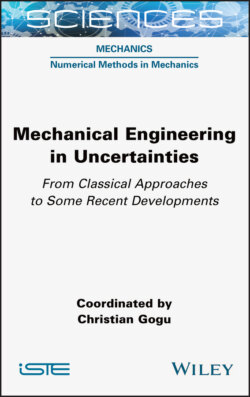
Реклама. ООО «ЛитРес», ИНН: 7719571260.
Оглавление
Группа авторов. Mechanical Engineering in Uncertainties From Classical Approaches to Some Recent Developments
Table of Contents
List of Illustrations
List of Tables
Guide
Pages
Mechanical Engineering under Uncertainties. From Classical Approaches to Some Recent Developments
Foreword
Preface
1. Uncertainty Modeling
1.1. Introduction
1.2. The usefulness of separating epistemic uncertainty from aleatory uncertainty
1.3. Probability theory
1.3.1. Theoretical context
1.3.2. Probabilistic approach for modeling aleatory uncertainties
1.3.3. Probabilistic approach for modeling epistemic uncertainties
1.4. Probability box theory (p-boxes)
1.5. Interval analysis
1.6. Fuzzy set theory
1.7. Possibility theory. 1.7.1. Theoretical context
1.7.2. Comparison between probability theory and possibility theory
1.7.3. Rules for combining possibility distributions
1.8. Evidence theory
1.8.1. Theoretical context
1.8.2. Rules for combining belief mass functions
1.9. Evaluation of epistemic uncertainty modeling
1.10. References
2. Microstructure Modeling and Characterization
2.1. Introduction
2.2. Probabilistic characterization of microstructures. 2.2.1. Random sets
2.2.2. Covariance
2.2.3. Granulometry
2.2.4. Minkowski functionals
2.2.5. Stereology
2.2.6. Linear erosion
2.2.7. Representative volume element
2.3. Point processes
2.3.1. Homogeneous Poisson point processes
2.3.2. Inhomogeneous Poisson point processes
2.4. Boolean models. 2.4.1. Definition and Choquet capacity
2.4.2. Properties
2.4.3. Covariance
2.4.4. Other characteristics. 2.4.4.1. Three-point function
2.4.4.2. Contact distribution
2.4.4.3. Specific surface area
2.4.4.4. Linear erosion curves for convex primary grains
2.5. RSA models
2.6. Random tessellations
2.6.1. Voronoi tessellation
2.6.2. Johnson–Mehl tessellation
2.6.3. Laguerre tessellation
2.6.4. Random Poisson tessellation
2.6.5. The dead-leaves model
2.6.6. Generalized random partition models
2.7. Gaussian fields
2.8. Conclusion
2.9. Acknowledgments
2.10. References
3. Uncertainty Propagation at the Scale of Aging Civil Engineering Structures
3.1. Introduction
3.2. Problem positioning. 3.2.1. Probabilistic formulation
3.2.2. Thermo-hydro-mechanical-leakage transfer function
3.2.3. Resulting probabilistic THM-F problem
3.3. Random field-based modeling of material properties
3.3.1. Random fields
3.3.2. Generation methods for discretized random fields
3.3.2.1. Discrete approximations
3.3.2.2. Functional approximations
3.3.3. Random fields and autocorrelations
3.3.4. Application: contribution to modeling the cracking of reinforced concrete works by self-correlated r.f. 3.3.4.1. Context presentation
3.3.4.2. Effect of autocorrelated r.f
3.4. Modeling uncertainty propagation using response surface methods
3.4.1. Probabilistic coupling strategies
3.4.1.1. Monte Carlo or quasi-Monte Carlo-based approaches
3.4.1.2. Spectral approaches to metamodeling
3.4.2. Polynomial chaos method
3.4.2.1. Application: contribution to the modeling of cracking of reinforced concrete works at an early age using metamodeling
3.4.2.2. Application: contribution to the modeling of the THM+L uncertainty propagation in a reinforced concrete structure by polynomial chaos methods
3.5. Conclusion
3.6. References
4. Reduction of Uncertainties in Multidisciplinary Analysis Based on a Polynomial Chaos Sensitivity Study
4.1. Introduction
4.2. MDA with model uncertainty. 4.2.1. Formalism
4.2.2. Solving the random MDA
4.2.3. Approximation of the quantity of interest using sparse polynomial chaos
4.3. Sensitivity analysis and uncertainty reduction. 4.3.1. Introduction
4.3.2. Sobol’ indices approximated by polynomial chaos
4.4. Application to an aeroelastic test case. 4.4.1. Presentation
4.4.2. Construction of disciplinary metamodels
4.4.3. Sensitivity analysis and uncertainty reduction
4.5. Conclusion
4.6. References
5. Rare-event Probability Estimation
5.1. Introduction
5.1.1. Mapping to the multivariate standard normal space
5.1.2. Copulas and correlation
CDF of random vector (X1,…, Xk)T
Conditional CDF of Xk|X1,…, Xk−1
5.1.3. Isoprobabilistic transformations. 5.1.3.1. Independent random inputs
5.1.3.2. Nataf transformation
5.1.3.3. Rosenblatt transformation
5.2. MPFP-based methods. 5.2.1. First-order reliability method. 5.2.1.1. Problem statement
5.2.1.2. Solving the constrained optimization problem
5.2.1.3. Remarks about the accuracy of the FORM solution
5.2.2. Second-order reliability method. 5.2.2.1. Curvature-fitting SORM
5.2.2.2. Point-fitting SORM
5.3. Simulation methods
5.3.1. Crude MC simulation
5.3.2. Subset simulation
5.3.2.1. Conceptual idea of subset simulation
5.3.2.2. Description of the SS algorithm
5.3.2.3. Approximate sampling from φn (·|Es−1) for s = 2, …,m
5.3.2.4. Statistical properties of the SS failure probability estimator. 5.3.2.4.1. Coefficient of variation of
5.3.2.4.2. Bias of
5.3.2.5. Remark about the computer implementation of SS
5.3.3. IS and CE methods
5.3.3.1. Importance sampling
5.3.3.2. CE method
5.3.3.2.1. CE method for estimation
5.3.3.2.2. Multilevel CE method
5.4. Sensitivity measures. 5.4.1. Introduction
5.4.2. FORM. 5.4.2.1. Importance factors
5.4.2.2. Sensitivities of pf w.r.t. distribution parameters
5.4.2.2.1. Sensitivities w.r.t. correlation
5.4.2.2.2. Sensitivities w.r.t. other distribution parameters
5.4.3. Crude MC simulation and subset simulation. 5.4.3.1. Crude MC sensitivities of pf w.r.t. distribution parameters: the score function
5.4.3.2. SS sensitivities of pfw.r.t. distribution parameters
5.5. References
6. Adaptive Kriging-based Methods for Failure Probability Evaluation: Focus on AK Methods
6.1. Introduction
6.2. Presentation of Kriging. 6.2.1. Principle
6.2.2. Identification of Kriging hyperparameters
6.2.3. Kriging-based prediction
6.2.4. Illustration of Kriging-based prediction
6.3. Employing Kriging to calculate failure probabilities
6.3.1. The EFF function
6.3.2. The U function
6.3.3. The IMSET function
6.3.4. The SUR function
6.3.5. The H function
6.3.6. The OBJ function
6.3.7. The L function
6.3.8. Discussion
6.4. The AK-MCS method: presentation and generic principle. 6.4.1. Presentation of the AK-MCS method
6.4.2. Illustration of the AK-MCS method
6.4.3. Discussion
6.5. The AK-IS method for estimating probabilities of rare events. 6.5.1. Presentation of the AK-IS method
6.5.2. Illustration of the AK-IS method
6.5.3. Discussion
6.6. The AK-SYS method for system reliability problems. 6.6.1. Some generalities about system reliability analysis
6.6.2. Presentation of the AK-SYS method
6.6.3. Illustration of the AK-SYS method
6.6.4. Alternatives to the AK-SYS method
6.6.5. Application to problems indexed by a subset
6.7. The AK-HDMR1 method for high-dimensional problems
6.7.1. HDMR functional decomposition
6.7.2. Presentation of the AK-HDMR1 method
6.8. Conclusion
6.9. References
7. Global Reliability-oriented Sensitivity Analysis under Distribution Parameter Uncertainty
7.1. Introduction
7.2. Theoretical framework and notations
7.3. Global variance-based reliability-oriented sensitivity indices
7.3.1. Introducing the Sobol’ indices on the indicator function
7.3.2. Rewriting Sobol’ indices on the indicator function using Bayes’ theorem
7.4. Sobol’ indices on the indicator function adapted to the bi-level input uncertainty. 7.4.1. Reliability analysis under distribution parameter uncertainty
7.4.2. Bi-level input uncertainty: aggregated versus disaggregated types of uncertainty
7.4.3. Disaggregated random variables
7.4.4. Extension to the bi-level input uncertainty and pick-freeze estimators
7.4.4.1. Step #1
7.4.4.2. Step #2
7.4.4.3. Step #3
7.4.4.4. Step #4
7.5. Efficient estimation using subset sampling and KDE. 7.5.1. The problem of estimating the optimal distribution at failure
Box 7.1. Basics of multivariate kernel density estimation
7.5.1.1. Challenge #1: input dimension
7.5.1.2. Challenge #2: complex shape of the optimal density at failure
7.5.1.3. Challenge #3: limited number of failure points in the elite set
7.5.1.4. Challenge #4: accuracy of the KDE
7.5.2. Data-driven tensorized KDE
7.5.2.1. Context and prerequisites
7.5.2.2. Main features of the data-driven tensorized G-KDE
7.5.3. Methodology based on subset sampling and data-driven tensorized G-KDE
7.6. Application examples
7.6.1. Example #1: a polynomial function toy-case. 7.6.1.1. Description
7.6.1.2. Results
7.6.2. Example #2: a truss structure. 7.6.2.1. Description
7.6.2.2. Results
7.6.3. Example #3: application to a launch vehicle stage fallback zone estimation. 7.6.3.1. Description
7.6.3.2. Results
7.6.4. Summary about numerical results and discussion
7.7. Conclusion
7.8. Acknowledgments
7.9. References
8. Stochastic Multiobjective Optimization: A Descent Algorithm
8.1. Introduction
8.2. Mathematical refresher
8.2.1. Stochastic processes
8.2.2. Convex analysis
8.2.2.1. Convex set and convex envelope
8.2.2.2. Subdifferentials and directional derivatives
8.2.2.3. Numerical construction of the Clarke subdifferential
8.3. Multiobjective optimization and common descent vector
8.3.1. Binary relations
8.3.2. Multiobjective optimization, Pareto preorder
8.3.3. Common descent vector
8.4. Descent algorithm for multiobjective optimization and its extension to the stochastic framework. 8.4.1. Multiple gradient descent algorithm
8.4.2. Stochastic multiple gradient descent algorithm
8.4.2.1. Multiobjective stochastic optimization problem
8.4.2.2. Algorithm and theoretical results
8.4.2.2.1. SMGDA algorithm
8.4.2.2.2. H assumptions
8.5. Illustrations. 8.5.1. Performance of the SMGDA algorithm
8.5.2. Multiobjective approach to RBDO problems
8.5.3. Rewriting the probabilistic constraint
8.6. References
List of Authors
Index
A
B, C
D, E
F
G, I
K, L
M, P
R
S
T
U, V
WILEY END USER LICENSE AGREEMENT
Отрывок из книги
Mechanics, Field Director – Gilles Pijaudier-Cabot
.....
As we have seen in previous sections, one of the major problems in modeling epistemic uncertainties resides in the determination of the probability distribution (or its bounding) that a certain physical quantity follows. However, in a large majority of cases, the uncertainty can at least be bounded. A range with very wide bounds can often be obtained by considering constraints of physical nature on the quantities of interest. For example, physical considerations make it possible to limit the Poisson ratio of a homogeneous isotropic material between –1 and 0.5. Even if relatively narrow, this range is not very informative. The opinion of an expert, on the other hand, could allow this range to be further significantly reduced. In our example, an expert could, for example, state that, for the material that we are considering, the Poisson ratio will be between 0.2 and 0.4. Note that, based on this information, we cannot characterize how the uncertainty varies within this range (are the boundary values as plausible as the center values?). Nonetheless, there are situations where a range is the only information available.
.....