Using Predictive Analytics to Improve Healthcare Outcomes
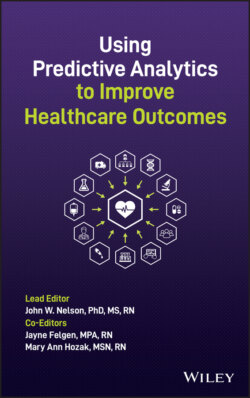
Реклама. ООО «ЛитРес», ИНН: 7719571260.
Оглавление
Группа авторов. Using Predictive Analytics to Improve Healthcare Outcomes
Table of Contents
List of Tables
List of Illustrations
Guide
Pages
Using Predictive Analytics to Improve Healthcare Outcomes
Contributors
Foreword
Preface: Bringing the Science of Winning to Healthcare
List of Acronyms
Acknowledgments
1 Using Predictive Analytics to Move from Reactive to Proactive Management of Outcomes
The Art and Science of Making Data Accessible
Step 1: Identify the Variable of Interest
Step 2: Identify the Things That Relate to the Variable of Interest: AKA, Predictor Variables
Step 3: Organize the Predictor Variables by Similarity to Form a Structural Model
Step 4: Rank Predictor Variables Based on How Directly They Appear to Relate to the Variable of Interest
Step 5: Structure the Predictor Variables into a Model in Order to Visually Communicate Their Relationship to the Variable of Interest
Step 6: Evaluate if and/or Where Data on the Predictor Variables Is Already Being Collected (AKA, Data Discovery)
Step 7: Find Ways to Measure Predictor Variables Not Currently Being Measured
Step 8: Select an Analytic Method
Step 9: Collect Retrospective Data Right Away and Take Action
Step 10: Examine Data Before Analysis
Step 11: Analyze the Data
Step 12: Present Data to the People Who Work Directly With the Variable of Interest, and Get Their Interpretation of the Data
Step 13: Respecify (Correct, Refine, and/or Expand) the Measurement Model
Step 14: Repeat Steps 2–13 if Explained Variance Declines
Step 15: Interface and Automate
Step 16: Write Predictive Mathematical Formulas to Proactively Manage the Variable of Interest
Summary 1: The “Why”
Summary 2: The Even Bigger “Why”
Implications for the Future
2 Advancing a New Paradigm of Caring Theory
Maturation of a Discipline
Theory
Caring Theory
Sociotechnical Systems Theory
Frameworks of Care
RBC's Four Decades of Wisdom
The Three Key Relationships in Relationship‐Based Care
Relationship with Self/Care of Self
Relationship with Colleagues/Care of Colleagues
Relationship with Patients and Families/Care of Patients and Families
Responsibility + Authority + Accountability (R+A+A)
Responsibility
Authority
Accountability
Clarity of Self, Role, and System
Clarity of Self
Clarity of Role
Clarity of System
Primary Nursing
Summary
Note
3 Cultivating a Better Data Process for More Relevant Operational Insight
Taking on the Challenge
“PSI RNs”: A Significant Structural Change to Support Performance and Safety Improvement Initiatives and Gain More Operational Insight
The Importance of Interdisciplinary Collaboration in Data Analysis
Key Success Factors
Education Levels of PSI RNs
Recognition of Excellence
Summary
4 Leadership for Improved Healthcare Outcomes
Data as a Tool to Make the Invisible Visible
Identifying a Bully
Managers Who Are “Buddies”
Using Data to Bring Objectivity to Sensitive Topics
Leaders Using Data for Inspiration: Story 1
Leaders Using Data for Inspiration: Story 2
How Leaders Can Advance the Use of Predictive Analytics and Machine Learning
Understanding an Organization's “Personality” Through Data Analysis
Note
5 Using Predictive Analytics to Reduce Patient Falls
Predictors of Falls, Specified in Model 1
Lessons Learned from This Study
Lesson 1: Age Was a Factor, But in an Unexpected Way
Lesson 2: Patients Resist Assistance When They Underestimate Their Own Fall Risks
Lesson 3: A Blame‐Free Environment Accelerates Fall Reduction Efforts
Lesson 4: Clarity Around R+A+A Is Essential
Lesson 5: Informal Conversation Was the Biggest Factor in Spreading the Word About the Study
Lesson 6: Complete and Accurate Data Are Essential to Any Study
Respecifying the Model
Summary
6 Using the Profile of Caring® to Improve Safety Outcomes
The Profile of Caring
Machine Learning
Supervised Problems
Unsupervised Problems
Semi‐supervised Problems
Reinforcement Problems
Exploration of Two Variables of Interest: Early Readmission for Heart Failure and Falls
Readmission for Heart Failure
Review of Predictive Analytics Studies Related to Heart Failure
What Successful Interventions for Reduction in Readmissions for Heart Failure Teach Us About What to Do Next
Limitations of Past Work
Patient Falls
Limitations
Proposal for a Machine Learning Problem
The Profile of Caring
Constructing the Study for Our Machine Learning Problem
Machine Learning Provides Data for the Real‐Time Monitoring of Safety
Using the Profile of Caring to Proactively Hire and Train Staff for Safety
Notes
7 Forecasting Patient Experience: Enhanced Insight Beyond HCAHPS Scores
Methods to Measure the Patient Experience
Results of the First Factor Analysis
Implications of This Factor Analysis
Predictors of Patient Experience
Discussion
Transforming Data into Action Plans
Summary
Notes
8 Analyzing a Hospital‐Based Palliative Care Program to Reduce Length of Stay
Building a Program for Palliative Care
The Context for Implementing a Program of Palliative Care
Building a Model to Study Length of Stay in Palliative Care
Demographics of the Patient Population for Model 1
Results from Model 1
Respecifying the Model
Results from Model 2
Discussion
9 Determining Profiles of Risk to Reduce Early Readmissions Due to Heart Failure
Step 1: Seek Established Guidelines in the Literature
Step 2: Crosswalk Literature with Organization's Tool
Step 3: Develop a Structural Model of the 184 Identified Variables
Step 4: Collect Data
Details of the Study
Variables for Which Data Was Collected
Zeroing in on Frequency of Readmission
Limitations of the Study
Results: Predictors of Readmission in Fewer Than 30 Days
Summary of Variables That Proved Insignificant After Analysis
Summary of Inconclusive Findings
Description of Inconclusive Findings Warranting Further Study
Discharge Disposition
Cardiologist
Variables That Proved Significant Predictors of Readmission for Heart Failure in Fewer Than 30 Days
Concurrent Diagnoses/Comorbidities
Left Ventricular Systolic Dysfunction (LVSD)
Ejection Fraction
Total Number of Readmissions
Follow‐Up Phone Call Scheduled
Next Steps
Notes
10 Measuring What Matters in a Multi‐Institutional Healthcare System
Testing a Model of Caring
Methods
Results from the Study of Model 1
Respecifying the Model
Testing Model 2
Results from the Study of Model 2
The Self‐Care Factor
Further Discussion
Summary
11 Pause and Flow: Using Physics to Improve the Efficiency of Workflow
Types of Pause
Types of Flow
Methods
Sample Size and Response Rates
What We Learned About Pause
What We Learned About Flow
Recall or Reflect?
Application of Results to Operations
Reflections from the Medical Unit—R6S
Lessons from Engaging in the Study Itself
A Lesson from the Results of the Study
What the Manager Learned About Her Own Practice
Changes Made on the Medical Unit Because of the Study
Analyzing Pause and Flow of Work as a Method of Quality Improvement
Summary and Next Steps
Note
12 Lessons Learned While Pursuing CLABSI Reduction
Development of a Specified Model of Measurement for Prevention of CLABSI
First Lesson Learned: Quality Data Collection Requires Well‐Trained Data Collectors
Other Lessons Learned
Lesson 1: Auditors Can and Should Mentor Staff Members
Lesson 2: Risks Identified in the Literature May Not Match Your Reality
Lesson 3: Practice Errors, Beyond What You Are Looking for, May Be Discovered
Lesson 4: Competence in Counting Numerator and Denominator Data Is Essential
Lesson 5: Leverage the Process to Advance Clinical Practice of Nurses
Summary and Next Steps
13 Theory and Model Development to Address Pain Relief by Improving Comfort
A New Theory
Developing a New Model Based on a New Theory
The Shift: The Physiology of Pain Includes the “Physiology of Comfort”
Clinicians' Beliefs Drive Their Practice
Dimensions of Comfort
Dimensions of Comfort That Clinicians Seek to Decrease. Fear
Pain
Suffering
Grief/Loss of Function
Dimensions of Comfort That Clinicians Seek to Increase. Trust
Well‐being
Perceived Inclusion
Predictors of Comfort
External Predictors of Comfort
Internal Predictors of Comfort
The Model
Summary
NOTES
14 Theory and Model Development to Improve Recovery from Opioid Use Disorder
The Current Costs of Opioid Use Disorder (OUD)
Interventions for OUD
Introduction of a “Trusted Other”
Pain Management, OUD, and Therapeutic Relationships
Interventions Which Include Potential Trusted Others
Common Relationships in OUD Recovery Programs
Case Managers
Recovery/Connection Coach
Recovery Management Checkups
Peer Case Managers, Outreach Workers, and Recovery Specialists
Addiction Counseling Professionals
Existing OUD Measurement Instruments
Updating the Old OUD Measurement Instrument and Model to Include the Trusted Other
Discussion
Broader Recommendations for People Designing Recovery Programs
Advancing a Culture That Supports Clinicians to Become Trusted Others
Conclusion
Note
15 Launching an International Trajectory of Research in Nurse Job Satisfaction, Starting in Jamaica
Background
The Hunch: Where Measurement Begins
The Model
Using Theory to Explain the Model
Measuring the Model
Understanding the Context of Jamaica
Methods to Study Job Satisfaction and Clarity in Jamaica
Stage 1:
Stage 2:
Stage 3:
Managing Disappointment with the Low Response Rate
Results on the Social and Technical Dimensions of Nurse Job Satisfaction in Jamaica
Results on the Relationship of Role Clarity and Demographics to Nurse Job Satisfaction in Jamaica
Application of the Findings
NOTES
16 Testing an International Model of Nurse Job Satisfaction to Support the Quadruple Aim
The Four Goals of Our Study. Goal 1:
Goal 2:
Goal 3:
Goal 4:
Methods
Sample and Setting
Theoretical Framework
Measurement Instruments and a Model of Measurement
Order of Operations of the Study. Step 1:
Step 2:
Step 3:
Step 4:
Step 5:
Step 6:
Simplifying the Model
What We Needed to Understand About Job Satisfaction and Clarity Before We Added Caring
Respecifying the Model to Include Caring
Results from Model 2
How Job Satisfaction Relates to Turnover and Sick Time
Focus on Turnover
Sick Time
Recommendations Based on Findings
Notes
17 Developing a Customized Instrument to Measure Caring and Quality in Western Scotland
Developing an Instrument to Measure Caring as Perceived by the Patient
Watson's Caring Factor Survey (CFS)
Healing Compassion Assessment (the CFS Survey Customized for Use in Western Scotland)
The Healing Compassion Survey—7 Cs NHS Scotland (Patient/Family Version)
Developing an Instrument to Measure Caring as Perceived by the Nursing Staff
The Healing Compassion Survey—7 Cs NHS Scotland (Staff Version)
Building a Structural Model for Assessing How Caring, Clarity, and Job Satisfaction Relate to One Another in Western Scotland
Results
Results: Caring and Quality, as Reported by the Patient
Results: Job Satisfaction as Reported by the Nursing Staff
Comparing Job Satisfaction Over Time
Testing the Final Model to Measure the Experience of Nurses
Discussion
18 Measuring the Effectiveness of a Care Delivery Model in Western Scotland
The Caring Behaviors Assurance System (CBAS)
Raise Quality Standards
Create Employee Accountability for Quality and Safety in the Care Experience
Establish the Self‐Care of Clinicians as Central to Caring
Establish Ward‐Level Employee “Ownership” for Care Delivery
Implementation of CBAS
Measurement of CBAS
The Patient‐centred Care Quality Instrument (PCQI)
The Operations of CBAS Assesssment
The Healthcare Environment Survey (HES)
Findings from the PCQI, the Operations of CBAS Assessment, and the HES
Effectiveness of CBAS Discovered by Using the PCQI
Care and Compassion
Communication
Collaboration
Clean Environment
Continuity of Care
Clinical Excellence
Effectiveness of CBAS Discovered by Using the Operations of CBAS Assessment and the HES
How Knowledge of CBAS Affected Caring as Perceived by Staff
How Knowledge of CBAS Affected Job Satisfaction
Action Planning
Ward 2 East (Post‐Surgical Care): Action Plans and Outcomes
Ward 2 West (Postsurgical Care): Action Plans and Outcomes
Discussion
Epilogue: Imagining What Is Possible
Appendix A Worksheets Showing the Progression from a Full List of Predictor Variables to a Measurement Model
Appendix B The Key to Making Your Relationship-Based Care® Implementation Sustainable Is “I2E2”
Vision
Inspiration (I1)
Infrastructure (I2)
Education (E1)
Evidence (E2)
Appendix D Calculation for Cost of Falls
Appendix D Possible Clinical, Administrative, and Psychosocial Predictors of Readmission for Heart Failure in Fewer Than 30 Days After Discharge
Appendix E Process to Determine Variables for Lee, Jin, Piao, & Lee, 2016 Study
Patient Factors Included in the Final Model
Environmental Factors Included in the Model
Medical Interventions Included in the Model
Appendix F Summary of National and International Heart Failure Guidelines. National Institute for Health and Care Excellence (NICE) Guidelines
European Society of Cardiology (ESC), Acute and Chronic Heart Failure Guidelines
American College of Cardiology Foundation (ACCF)/American Heart Association (AHA), 2013 and 2017 Guidelines for Management of Heart Failure
Appendix G Crosswalk Hospital Tool and Guidelines
Appendix H Comprehensive Model of 184 Variables Found in Guidelines and Hospital Tool
Appendix I Summary of Variables That Proved Insignificant After Analysis
Age
Gender
Payment Type
History of Smoking
Vital Signs (Heart Rate and Blood Pressure)
Serum Creatinine
EKG
Follow‐Up Visit Scheduled
ICD Codes 9 and 10
Hydralazine Nitrate Prescribed
Aldosterone Antagonist at Discharge
Beta Blocker Prescribed at Discharge
Anticoagulation Medication Prescribed at Discharge
ARNI Prescribed at Discharge
ARB Prescribed at Discharge
ACE Inhibitor Prescribed at Discharge
Pneumococcal Vaccination
Influenza Vaccination
Appendix J Summary of Inconclusive Findings
Race
Ethnicity
Smoking Cessation
Activity Level
Appendix K Nine Tools for Measuring the Provision of Quality Patient Care and Related Variables
Three Tools for Assessing “Caring for the Patient” As Perceived By the Patient
Tool 1: The Caring Factor Survey (CFS)
Tool 2: The Caring Professional Scale (CPS)
Tool 3: The Caring Assessment Tool (CAT)
Three Tools for Assessing “Caring for the Patient” as Perceived By Staff Members
Tool 1: The Caring Factor Survey—Care Provider Version (CFS‐CPV)
Tool 2: The Caring Professional Scale—Care Provider Version (CPS‐CPV)
Tool 3: The Caring Efficacy Scale (CES)
Tools to Measure Self‐Care; Nurse Job Satisfaction; and Clarity of Self, Role, and System
The Tool for Assessing Caring for Self as Perceived by the Staff
The Tool for Assessing Nurse Job Satisfaction
The Tool for Assessing “Clarity of Self, Role, and System”
Notes
Appendix L Data From Pause and Flow Study Related to Participants’ Ability to Recall Moments of Pause and Flow Easily or with Reflection
Data on Participants’ Ability to Recall Pause
Data on Participants’ Ability to Recall Flow
Appendix M Identified Pauses and Proposed Interventions Resulting from a Pause and Flow Study
Pause
Intervention
Pause
Intervention
Pause
Intervention
Appendix N Factors Related to a Focus on Pain Versus Factors Related to a Focus on Comfort
Appendix O Comfort/Pain Perception Survey (CPPS)—Patient Version
Appendix P Comfort/Pain Perception Survey (CPPS)—Care Provider Version
Appendix Q Predictors of OUD
Appendix R Personal Qualities of Clinicians and Others Suited to Become Trusted Others
Modeling “Not Knowing”
Cultivating Self‐Awareness
Commitment to Perspective‐Seeking
Appendix S Qualities of Systems and Organizations Suited to Serve People Recovering from OUD
Advancing a Culture in Which “Not Knowing” Is Accepted
Advancing a Culture in Which Systemic Awareness Is Actively Sought
Advancing a Culture in Which Perspective Seeking Is Prized
Appendix T Factor Loadings for Satisfaction with Staffing/Scheduling and Resources
Appendix U Detail Regarding Item Reduction of Instruments to Measure Caring
Appendix V Factor Loading for Items in the Healing Compassions Assessment (HCA) for Use in Western Scotland
Appendix W Factor Loadings of the Caring Professional Scale for Use in Western Scotland
Appendix X Factor Loadings for the Healing Compassion Survey—7Cs NHS Scotland (Staff Version)
Appendix Y Factor Analysis and Factor Ranking for Survey Items Related to Caring for Self and Caring of the Senior Charge Nurse
Appendix Z Demographics, Particularly Ward, as Predictors of Job Satisfaction
Appendix AA Demographic as Predictors of clarity
Appendix BB Correlates of Operations of CBAS with Items from the Healing Compassion Survey—7 Cs NHS Scotland (Staff Version)
References
Index. a
b
c
d
e
f
g
h
i
j
k
l
m
n
o
p
q
r
s
t
u
v
w
WILEY END USER LICENSE AGREEMENT
Отрывок из книги
Edited by
.....
Denver, Colorado, US
Dawna Maria Perry Chief Nursing Officer Thunder Bay Regional Health Science Center Thunder Bay, Ontario, Canada
.....