Global Drought and Flood
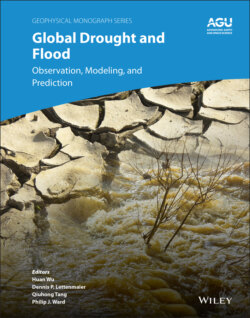
Реклама. ООО «ЛитРес», ИНН: 7719571260.
Оглавление
Группа авторов. Global Drought and Flood
Table of Contents
List of Tables
List of Illustrations
Guide
Pages
Geophysical Monograph Series
Global Drought and Flood. Observation, Modeling, and Prediction
LIST OF CONTRIBUTORS
PREFACE
1 Progress, Challenges, and Opportunities in Remote Sensing of Drought
ABSTRACT
1.1. INTRODUCTION
1.2. PROGRESS IN REMOTE SENSING OF DRIVERS OF DROUGHT
1.2.1. Precipitation
1.2.2. Soil Moisture
1.2.3. Relative Humidity
1.2.4. Evapotranspiration
1.2.5. Snow
1.2.6. Groundwater
1.3. MULTI‐INDICATOR DROUGHT MODELING
1.4. DROUGHT AND HEATWAVES FEEDBACKS
1.5. REMAINING CHALLENGES AND OPPORTUNITIES
1.6. CONCLUSION
REFERENCES
2 Remote Sensing of Evapotranspiration for Global Drought Monitoring
ABSTRACT
2.1. INTRODUCTION
2.2. HISTORICAL SKETCH OF ET REMOTE SENSING STUDIES AND ET DATA PRODUCTS
2.3. ESTIMATING ET AND MONITORING DROUGHT WITH GEOSTATIONARY SATELLITE THERMAL OBSERVATIONS. 2.3.1. The ALEXI Model
2.3.2. Extrapolation from Instantaneous to Hourly and Daily Fluxes
2.3.3. Potential Evapotranspiration
2.3.4. Evaporative Stress Index
2.4. DROUGHT MONITORING PRODUCT SYSTEM BASED ON ET REMOTE SENSING. 2.4.1. Theoretical Description of ET and Drought Monitoring Product System
2.4.2. GOES ET and Drought Product System Design
2.4.3. GET‐D System Outputs
2.5. COMBINING ET REMOTE SENSING WITH MICROWAVE SOIL MOISTURE DATA FOR DROUGHT MONITORING
2.6. DISCUSSION
ACKNOWLEDGMENTS
REFERENCES
3 Drought Monitoring Using Reservoir Data Collected via Satellite Remote Sensing
ABSTRACT
3.1. INTRODUCTION
3.2. DROUGHT MONITORING USING REMOTELY SENSED RESERVOIR DATA. 3.2.1. Reservoir Elevation
3.2.2. Reservoir Storage
3.2.3. Reservoir Area
3.3. ADOPTING REMOTELY SENSED RESERVOIR DATA TO SUPPORT DROUGHT MODELING APPLICATIONS
3.4. FUTURE DIRECTIONS
3.5. DISCUSSION AND CONCLUSIONS
ACKNOWLEDGMENTS
REFERENCES
4 Automatic Near‐Real‐Time Flood Mapping from Geostationary Low Earth Orbiting Satellite Observations
ABSTRACT
4.1. INTRODUCTION
4.2. DATA USED
4.3. METHODS. 4.3.1. Physical Basis
4.3.2. Challenges
4.3.3. Algorithm Development
Water Detection
Cloud Shadow Removal
Terrain Shadow Removal
Minor Flood Detection
Water Fraction Retrieval
Flood Determination
4.3.4. Algorithm Process
4.4. APPLICATIONS
4.4.1. Application in Dynamic Flood‐Extent Monitoring
4.4.2. Application to Snowmelt and Ice‐Jam Flood Prediction and Monitoring
4.5. VALIDATION
4.5.1. Visual Inspection
4.5.2. Comparison with the MODIS Automatic Flood Products
4.5.3. Comparison with Radar Flood Products
4.5.4. Validation with Landsat‐8 OLI Imagery
4.6. DISCUSSION
4.6.1. Problems with VIIRS/ABI Flood Products
4.6.2. Potential Improvements on Future Flood Products
4.7. SUMMARY
ACKNOWLEDGMENTS
REFERENCES
5 Global Flood Observation with Multiple Satellites: Applications in Rio Salado (Argentina) and the Eastern Nile Basin
ABSTRACT
5.1. INTRODUCTION: THE STATE OF THE SCIENCE AND NEED FOR GLOBAL SATELLITE FLOOD MAPPING
5.1.1. The Need for Global Satellite‐Based Flood Maps
5.1.2. The Need for Satellite Flood Observation Locally: Rio Salado and Nile Basin
5.1.3. Current Satellites Available for Flood Mapping
5.2. METHODS FOR GLOBAL FLOOD OBSERVATION. 5.2.1. Generating Historic Flood‐Event Data Sets
The Dartmouth Flood Observatory
DesInventar
Media and Local Knowledge
5.2.2. Google Earth Engine
5.2.3. Remote Sensing Methods for Flood Event Mapping
MODIS Flood Mapping
Accuracy Assessment with the MODIS Flood Extent Data Sets
Landsat Flood Mapping
5.2.4. Recurrence Mapping, Frequency, and Historic Flooding. Flood Recurrence
Frequency
Historic Flooding
5.2.5. Precipitation Hyetographs
5.2.6. Population and Area Exposure
5.2.7. User‐Friendly Dashboards
5.3. WATERSHED CASE STUDIES: ARGENTINA AND THE EASTERN NILE REGION. 5.3.1. Rio Salado Basin, Argentina
5.3.2. Eastern Nile Basin
5.3.3. Flood Events for Argentina and Eastern Nile
5.4. RESULTS FROM FLOOD MAPPING IN CASE STUDIES. 5.4.1. Rio Salado
5.4.2. Eastern Nile Basin
5.5. LIMITATIONS AND FUTURE DIRECTIONS FOR THE UTILITY OF SATELLITE FLOOD‐EVENT DATA. 5.5.1. Limitations
5.5.2. Future Directions and Potential for Global Flood‐Event Mapping
5.6. CONCLUSION
ACKNOWLEDGMENTS
REFERENCES
6 Integrating Earth Observation Data of Floods with Large‐Scale Hydrodynamic Models
ABSTRACT
6.1. INTRODUCTION
6.2. EARTH OBSERVATION FLOOD DATA
6.2.1. Optical Range
6.2.2. Microwave Range
6.2.3. Altimeters
6.3. INTEGRATION OF EO DATA AND FLOOD MODELS
6.3.1. Model Calibration and Validation Methods
6.3.2. Assimilation Methods
6.4. OUTLOOK
6.5. CONCLUSION
REFERENCES
7 Global Integrated Drought Monitoring with a Multivariate Framework
ABSTRACT
7.1. INTRODUCTION
7.2. METHOD
7.3. DATA
7.4. RESULTS. 7.4.1. Illustration of the Indicator Property
7.4.2. Integrated Drought Monitoring
7.4.3. Drought Monitoring with Categories
7.4.4. Drought Monitoring Based on Rescaled MSDI
7.5. CONCLUSION
REFERENCES
8 A Probabilistic Framework for Agricultural Drought Forecasting Using the Ensemble Data Assimilation and Bayesian Multivariate Modeling
ABSTRACT
8.1. INTRODUCTION
8.2. REVIEW OF CURRENT DROUGHT FORECASTING SYSTEMS
8.2.1. Drought Forecasting Using Statistical Methods
8.2.2. Drought Forecasting Using Dynamical Methods
8.2.3. Drought Forecasting Using Coupled Methods
8.2.4. Current Operational Drought Forecasting Systems
8.3. THE PROPOSED COUPLED DYNAMICAL–STATISTICAL DROUGHT FORECASTING SYSTEM
8.3.1. The Overall Framework
8.3.2. Component I: Hydrologic Modeling
8.3.3. Component II: Land Surface Observations
8.3.4. Component III: Ensemble Data Assimilation
8.3.5. Component IV: Bayesian Network Model Using Copula Functions
8.3.6. Component V: High‐Performance Computing Implementation
8.4. CASE STUDIES
8.4.1. The Mid United States Drought of 2012
8.4.2. The 2017 Montana Drought
8.5. CONCLUSIONS AND DISCUSSION
REFERENCES
9 Integrating Soil Moisture Active/Passive Observations with Rainfall Data Using an Analytic Model for Drought Monitoring at the Continental Scale
ABSTRACT
9.1. INTRODUCTION
9.2. DATA AND METHOD. 9.2.1. Data
9.2.2. Analytic Model for Estimating Daily Surface Soil Moisture
9.3. RESULTS. 9.3.1. Model Training and Verification
9.3.2. A Case Study for Monitoring Drought
9.4. DISCUSSION AND CONCLUSIONS
ACKNOWLEDGEMENTS
REFERENCES
10 Global Flood Models
ABSTRACT
10.1. INTRODUCTION
10.1.1. The Challenges and History of GFM Development
10.2. TYPES OF GFM AND SPECIFIC EXAMPLES
10.2.1. Scale Characteristic
10.2.2. Model Forcing
10.2.3. Probability Estimation Methods
10.2.4. Calibration
10.2.5. Hydraulic Method
10.2.6. Other Relevant Models
10.3. APPLICATIONS OF GLOBAL FLOOD MODELS
10.3.1. Flood Hazard Mapping
10.3.2. Flood Risk Analysis
10.3.3. Flood Forecasting
10.3.4. Insurance Exposure
10.4. INSURANCE CATASTROPHE MODELS
10.4.1. Flood Hazard Mapping
10.4.2. Stochastic Precipitation and Discharge Scenarios
10.4.3. Flood Defenses
10.5. GFM CREDIBILITY. 10.5.1. The Importance of Model Credibility
10.5.2. Existing Model Testing
10.5.3. Collective Testing
10.6. THE FUTURE OF GFMS
10.6.1. Improvements in Data Sets for Model Build and Testing
10.6.2. Improvements in Processes Representation
10.6.3. Improved Model Testing
REFERENCES
11 Calibration of Global Flood Models: Progress, Challenges, and Opportunities
ABSTRACT
11.1. INTRODUCTION
11.2. GLOBAL HYDROLOGICAL MODEL CALIBRATION
11.2.1. Global Flood Awareness System
Hydrological Model
Streamflow and Forcing Data
Calibration Procedure and Evaluation
Calibration Results
11.2.2. Global Flood Monitoring Systems. Global Flood Monitoring System
Floods.Global System
Other Global Rainfall‐Runoff Models
11.3. MAIN CHALLENGES OF CALIBRATING GLOBAL HYDROLOGICAL MODELS
11.3.1. Data Limitation
11.3.2. Uncertainty in Forcing Data and Model Structure
11.3.3. Computational Cost
11.4. EMERGING OPPORTUNITIES
11.4.1. Improved Hydrological Monitoring
11.4.2. Improved Precipitation Forcing
11.4.3. Computational Efficiency
11.5. SUMMARY
REFERENCES
12 Digital Elevation Model and Drainage Network Data Sets for Global Flood and Drought Modeling
ABSTRACT
12.1. INTRODUCTION
12.2. GLOBAL BASELINE DIGITAL ELEVATION DATA FOR HYDROLOGICAL MODELING
12.2.1. Global DEM Data and General Evaluation Remarks
12.2.2. Errors Associated with GDEMs and Methods of Correction
Voids
Speckle Noise
Stripe Noise
Absolute Bias
Tree Height and Urban Area Biases
12.2.3. Challenges in Improving High‐Resolution GDEMs. Globally Consistent Error Removal Procedure
Dynamic Terrain Height
12.3. GLOBAL HYDROGRAPHY DATA SETS
12.3.1. High‐Resolution Hydrographic Data Sets
12.3.2. Moderate to Coarse Hydrographic Data Sets
Moderate to Coarse Hydrographic Data Sets Derived from a DEM
Moderate to Coarse Hydrographic Data Sets Using Upscaling Methods
12.3.3. Advanced Upscaling Method: Hierarchical Dominant River Tracing
12.4. CHALLENGES AND OPPORTUNITIES. 12.4.1. Multiple Flow Directions
12.4.2. Comprehensive Global Hydrography Data Set
12.5. SUMMARY
ACKNOWLEDGMENTS
REFERENCES
13 Fundamental Data Set for Global Drought and Flood Modeling: Land Use and Land Cover
ABSTRACT
13.1. INTRODUCTION
13.2. GLOBAL LAND COVER DATA SETS
13.2.1. Global Land Cover Characterization
13.2.2. University of Maryland Land Cover
13.2.3. Global Land Cover 2000
13.2.4. MODIS Land‐Cover Data Set
13.2.5. GlobCover Land‐Cover Product
13.2.6. Global Land Cover by National Mapping Organizations
13.2.7. Climate Change Initiative and Land Cover
13.2.8. GlobeLand30
13.2.9. Data fusion land cover data sets
SYNLCover
UN FAO Global Land‐Cover Network
13.3. DISCUSSION
REFERENCES
14 Global River Flood Risk Under Climate Change
ABSTRACT
14.1. INTRODUCTION
14.2. MODELING GLOBAL RIVER FLOOD RISK: GENERAL CONCEPTS AND METHODS
14.2.1. Risk Components
14.2.2. Risk Evaluation
14.2.3. Modeling Frameworks
14.3. THE GLOFRIS MODELING FRAMEWORK
14.3.1. Data and Methods
Hydrological and Hydraulic Modeling
Extreme Value Statistics
Inundation Modeling
Impact Modeling
14.3.2. Main Scientific Findings
14.3.3. Applications for Policy Support
14.4. CAMA‐FLOOD AND ISIMIP MODELING FRAMEWORKS
14.4.1. Data and Methods: Overview of the Modeling Chain
Climate Models
Global Hydrological Models
River Routing
Downscaling Inundation Area and Depth
Socioeconomic Impacts
14.4.2. Main Scientific Findings
14.5. THE GAR‐2015 FLOOD RISK FRAMEWORK
14.5.1. Data and Methods: Overview of the Modeling Chain
Hydrological Data and Modeling
River Routing and Inundation Modeling
Socioeconomic Impacts
14.5.2. Main Scientific Findings and Applications
14.6. THE JOINT RESEARCH CENTRE MODEL
14.6.1. Modeling Approach
Estimation of Scenarios of Flood Inundation in the Present Climate
Estimation of Flood Frequency and Magnitude
Assessment of Socioeconomic Flood Impacts
14.6.2. Main Scientific Findings
14.6.3. Applications for Policy and Emergency Support
14.7. OTHER FLOOD RISK MODELS
14.8. CONCLUSIONS
14.8.1. Improvement of Data Sets
14.8.2. Improvement in Modeling Processes
14.8.3. Testing and Integration of Risk Modeling Frameworks
REFERENCES
15 Direct Tangible Damage Classification and Exposure Analysis Using Satellite Images and Media Data
ABSTRACT
15.1. INTRODUCTION
15.2. DATA AND STUDY SITE. 15.2.1. Study Site
15.2.2. Remote Sensing Data
15.2.3. Television Media
15.3. METHOD
15.3.1. Land‐Cover Data
15.3.2. Flood Extent Mapping
Water Indexes Calculation
Histogram Thresholding
Flood Extent Mapping
15.3.3. Direct Tangible‐Damage Classification
15.4. RESULTS. 15.4.1. Flood Extent Mapping
15.4.2. Direct Tangible‐Damage Classification and Exposure Analysis. Building Damage Classification and Exposure Analysis
Road Damage Classification and Exposure Analysis
Farmland Damage Classification and Exposure Analysis
15.5. DISCUSSION. 15.5.1. Data Acquisition
15.5.2. Identification and Validation of Direct Tangible‐Damage Classification
15.6. CONCLUSIONS
ACKNOWLEDGMENTS
REFERENCES
16 Flood Risk and Monitoring Data for Preparedness and Response: From Availability to Use
ABSTRACT
16.1. INTRODUCTION
16.2. CHALLENGES IN UNDERSTANDING AND TRUSTING FLOOD DATA
16.2.1. Identification of Questions, Not Only Users
16.2.2. Clear Communication of the Type of Flood(s) the Data Pertain To
16.2.3. Appropriate Presentation of Uncertainty
16.3. TWO CASE STUDIES FRAMING THE DISCONNECT BETWEEN FLOOD DATA DEVELOPERS AND DECISION MAKERS
16.3.1. Bangladesh
Background
Identification of Flood Related Challenges
Key Questions Asked by Decision Makers. Methods
Results
What is the seasonality of flood risk for different types of floods?
What does the indicated flood level of a risk map mean?
If a flood risk map exists, does it illustrate the local areas flooded during a normal rainy season, or resulting from extreme events?
How often are these specific flood vulnerability maps updated?
What is the level of uncertainty associated with a given flood map?
What flood risk products are appropriate for each camp?
16.3.2. Malawi
Background
Identification of Flood Related Challenges
Identifying Key Questions Asked by Decision Makers. Methods
Results
Which map do we trust?
What types of floods have occurred?
If we are not sure about what flood data to trust, should we just prioritize responding for the areas that are usually flooded and are perceived to be the flooded areas?
16.4. IDENTIFICATION OF COMMON THEMES FOUND IN THE QUESTIONS ASKED WITHIN THE CASE STUDIES
16.4.1. What Can be Done to Explore “Appropriateness” or “Best” Use of Flood Data for Flood Risk Assessment and Flood Response?
16.4.2. What Influences a Disaster Manager’s Choice to Integrate One Flood Tool or Product Rather than Another when a Multitude of Tools and/or Products are Available?
16.4.3. What is this Map Actually Showing? Is it Showing Absolute Flood Information? Is it Showing Deviations from Normal Flooding?
16.4.4. Which Flood Data do I Trust for the Questions I am Asking?
16.5. SUGGESTED OPPORTUNITIES TO MOVE TOWARDS NARROWING THE GAP
16.5.1. Exploration of Methods to Identify Specific Flood Related Questions and Map Out Associated Decision‐Making Processes
16.5.2. Codevelopment of Tools that Address the Elements Identified in the DMF
16.5.3. Learning From and Leveraging Global Frameworks
16.6. CONCLUSION
ACKNOWLEDGMENTS
REFERENCES
17 Global Flood Partnership*
ABSTRACT
17.1. INTRODUCTION
17.2. MODELS AND PRODUCTS. 17.2.1. Early Warning Systems
Extreme Weather Forecasts
GFP Products
Flood Early Warning Systems
GFP Products
17.2.2. Scenario Analysis
Inundation Models
GFP Products
Satellite Imagery
GFP Products
17.2.3. Hydrological Modeling
GFP Products
17.2.4. Monitoring
GFP Products. Satellite‐based discharge estimation
Satellite‐Based Inundation Mapping
17.2.5. Users and Emergency Responders
GFP Products
17.3. GFP ACTIVATIONS. 17.3.1. The South Asia floods in August 2017
17.3.2. Hurricane Harvey Flooding in Texas and Louisiana, August–September 2017
27 August (2 days after landfall)
28 August (flooding in Houston Metropolitan area)
29 August
30 August
31 August
1 September
3–5 September
17.4. DISCUSSION AND CONCLUSIONS
REFERENCES
Note
18 Drought and Flood Monitoring and Forecasting: Challenges and Opportunities Ahead
ABSTRACT
18.1. REMOTE SENSING FOR DROUGHT AND FLOOD MODELING
18.2. DROUGHT AND FLOOD MODELING
18.3. RISK ANALYSIS AND COLLABORATION
18.4. PERSPECTIVE
INDEX
WILEY END USER LICENSE AGREEMENT
Отрывок из книги
.....
Brian Llamanzares International Research Institute for Climate and Society The Earth Institute, Columbia University Palisades, New York, USA
Valerio Lorini Disaster Risk Management Unit European Commission Joint Research Centre Ispra, Italy
.....