Industry 4.1
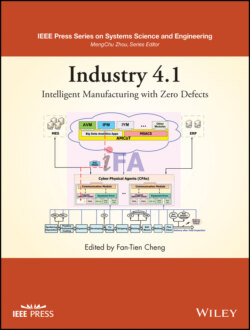
Реклама. ООО «ЛитРес», ИНН: 7719571260.
Оглавление
Группа авторов. Industry 4.1
Table of Contents
List of Tables
List of Illustrations
Guide
Pages
Industry 4.1. Intelligent Manufacturing with Zero Defects
Editor Biography
List of Contributors
Preface
Overview and Goals
Organization and Features
Acknowledgments
Foreword
1 Evolution of Automation and Development Strategy of Intelligent Manufacturing with Zero Defects
1.1 Introduction
1.2 Evolution of Automation
1.2.1 e‐Manufacturing
1.2.1.1 Manufacturing Execution System (MES)
1.2.1.2 Supply Chain (SC)
1.2.1.3 Equipment Engineering System (EES)
1.2.1.4 Engineering Chain (EC)
1.2.2 Industry 4.0
1.2.2.1 Definition and Core Technologies of Industry 4.0
1.2.2.2 Migration from e‐Manufacturing to Industry 4.0
1.2.2.3 Mass Customization
1.2.3 Zero Defects – Vision of Industry 4.1
1.2.3.1 Two Stages of Achieving Zero Defects
1.3 Development Strategy of Intelligent Manufacturing with Zero Defects
1.3.1 Five‐Stage Strategy of Yield Enhancement and Zero‐Defects Assurance
1.4 Conclusion
Appendix 1.A ‐ Abbreviation List
References
2 Data Acquisition and Preprocessing
2.1 Introduction
2.2 Data Acquisition
2.2.1 Process Data Acquisition
2.2.1.1 Sensing Signals Acquisition
Sensing Techniques
Sensor Selection and Installation
Force: Strain Gauge
Loading: Current Transducer
Vibration: Accelerometer
Temperature: Thermal Couple
AE Waves: AE Transducer
Sensor Fusion
2.2.1.2 Manufacturing Parameters Acquisition
2.2.2 Metrology Data Acquisition
2.3 Data Preprocessing
2.3.1 Segmentation
2.3.2 Cleaning
2.3.2.1 Trend Removal
2.3.2.2 Wavelet Thresholding
Decomposition
Thresholding
Reconstruction
2.3.3 Feature Extraction
2.3.3.1 Time Domain
Statistical SFs
EK‐based Selection Procedure
Cross‐Correlation SFs
Autocorrelation SFs
2.3.3.2 Frequency Domain
2.3.3.3 Time–Frequency Domain
2.3.3.4 Autoencoder
2.4 Case Studies
2.4.1 Detrending of the Thermal Effect in Strain Gauge Data
2.4.2 Automated Segmentation of Signal Data
2.4.3 Tool State Diagnosis
2.4.4 Tool Diagnosis using Loading Data
2.5 Conclusion
Appendix 2.A ‐ Abbreviation List
Appendix 2.B ‐ List of Symbols in Equations
References
3 Communication Standards
3.1 Introduction
3.2 Communication Standards of the Semiconductor Equipment
3.2.1 Manufacturing Portion
3.2.1.1 SEMI Equipment Communication Standard I (SECS‐I) (SEMI E4)
Block Protocol
Header
Timeouts
Protocol Parameters Summary
3.2.1.2 SEMI Equipment Communication Standard II (SECS‐II) (SEMI E5)
Message Transfer Protocol
Streams and Functions
Transaction and Conversation Protocols
Data Structure Rules
Example Messages
Notations of SECS‐II Messages
3.2.1.3 Generic Model for Communications and Control of Manufacturing Equipment (GEM) (SEMI E30)
GEM Requirements and Capabilities
GEM Compliant
3.2.1.4 High‐Speed SECS Message Services (HSMS) (SEMI E37)
HSMS‐Generic Services
HSMS‐SS and HSMS‐GS
HSMS Adoption
HSMS Design Principles
HSMS Message Format
HSMS‐SS Procedures
HSMS Timeouts
Configuring HSMS‐SS Parameters
Sharing Network with Other TCP/IP Protocols
Comparison and Migration of SECS‐I and HSMS
3.2.2 Engineering Portion (Interface A)
3.2.2.1 Authentication & Authorization (A&A) (SEMI E132)
3.2.2.2 Common Equipment Model (CEM) (SEMI E120)
3.2.2.3 Equipment Self‐Description (EqSD) (SEMI E125)
3.2.2.4 Equipment Data Acquisition (EDA) Common Metadata (ECM) (SEMI E164)
3.2.2.5 Data Collection Management (DCM) (SEMI E134)
DCP Definition
DCP Operation
DCR Definition
Event Report Definition and Example
Exception Report Definition and Example
Trace Report Definition and Example
Example of DCR Buffering
3.3 Communication Standards of the Industrial Devices and Systems
3.3.1 Historical Roadmaps of Classic Open Platform Communications (OPC) and OPC Unified Architecture (OPC‐UA) Protocols
3.3.1.1 Classic OPC
3.3.1.2 OPC‐UA
3.3.2 Fundamentals of OPC‐UA
3.3.2.1 Requirements
Data Modeling
Communication
3.3.2.2 Foundations
3.3.2.3 Specifications
3.3.2.4 System Architecture
OPC‐UA Services
Architecture Overview
OPC‐UA Client
OPC‐UA Server
Security Model
Sequence Diagram of OPC‐UA
3.3.3 Example of Intelligent Manufacturing Hierarchy Applying OPC‐UA Protocol
3.3.3.1 Equipment Application Program (EAP) Server
3.3.3.2 Use Cases of Data Manipulation
3.3.3.3 Sequence Diagrams of Data Manipulation
Sequence Diagram of Data Collection
Sequence Diagram of Real‐Time Monitoring
Sequence Diagram of Recipe Download
3.4 Conclusion
Appendix 3.A ‐ Abbreviation List
References
4 Cloud Computing, Internet of Things (IoT), Edge Computing, and Big Data Infrastructure
4.1 Introduction
4.2 Cloud Computing
4.2.1 Essentials of Cloud Computing
4.2.2 Cloud Service Models
4.2.3 Cloud Deployment Models
4.2.4 Cloud Computing Applications in Manufacturing
4.2.5 Summary
4.3 IoT and Edge Computing
4.3.1 Essentials of IoT
4.3.2 Essentials of Edge Computing
4.3.3 Applications of IoT and Edge Computing in Manufacturing
4.3.4 Summary
4.4 Big Data Infrastructure
4.4.1Application Demands
4.4.2 Core Software Stack Components
4.4.3 Bridging the Gap between Core Software Stack Components and Applications
4.4.3.1 Hadoop Data Service (HDS)
4.4.3.2 Distributed R Language Computing Service (DRS)
4.4.4 Summary
4.5 Conclusion
Appendix 4.A ‐ Abbreviation List
Appendix 4.B ‐ Abbreviation List
References
5 Docker and Kubernetes
5.1 Introduction
5.2 Fundamentals of Docker
5.2.1 Docker Architecture
5.2.1.1 Docker Engine
5.2.1.2 High‐Level Docker Architecture
5.2.1.3 Architecture of Linux Docker Host
5.2.1.4 Architecture of Windows Docker Host
5.2.1.5 Architecture of Windows Server Containers
5.2.1.6 Architecture of Hyper‐V Containers
5.2.2 Docker Operational Principles
5.2.2.1 Docker Image
5.2.2.2 Dockerfile
5.2.2.3 Docker Container
5.2.2.4 Container Network Model
5.2.3 Illustrative Applications of Docker
5.2.3.1 Workflow of Building, Shipping, and Deploying a Containerized Application
5.2.3.2 Deployment of a Docker Container Running a Linux Application
5.2.3.3 Deployment of a Docker Container Running a Windows Application
5.2.4 Summary
5.3 Fundamentals of Kubernetes
5.3.1 Kubernetes Architecture
5.3.1.1 Kubernetes Control Plane Node
5.3.1.2 Kubernetes Worker Nodes
5.3.1.3 Kubernetes Objects
5.3.2 Kubernetes Operational Principles
5.3.2.1 Deployment
5.3.2.2 High Availability and Self‐Healing
5.3.2.3 Ingress
5.3.2.4 Replication
5.3.2.5 Scheduler
5.3.2.6 Autoscaling
5.3.3 Illustrative Applications of Kubernetes
5.3.4 Summary
5.4 Conclusion
Appendix 5.A ‐ Abbreviation List
References
6 Intelligent Factory Automation (iFA) System Platform
6.1 Introduction
6.2 Architecture Design of the Advanced Manufacturing Cloud of Things (AMCoT) Framework
6.3 Brief Description of the Automatic Virtual Metrology (AVM) Server
6.4 Brief Description of the Baseline Predictive Maintenance (BPM) Scheme in the Intelligent Prediction Maintenance (IPM) Server
6.5 Brief Description of the Key‐variable Search Algorithm (KSA) Scheme in the Intelligent Yield Management (IYM) Server
6.6 The iFA System Platform
6.6.1 Cloud‐based iFA System Platform
6.6.2 Server‐based iFA System Platform
6.7 Conclusion
Appendix 6.A ‐ Abbreviation List
Appendix 6.B ‐ List of Symbols
References
7 Advanced Manufacturing Cloud of Things (AMCoT) Framework
7.1 Introduction
7.2 Key Components of AMCoT Framework
7.2.1 Key Components of Cloud Part
7.2.2 Key Components of Factory Part
7.2.3 An Example Intelligent Manufacturing Platform Based on AMCoT Framework
7.2.4 Summary
7.3 Framework Design of Cyber‐Physical Agent (CPA)
7.3.1 Framework of CPA
7.3.2 Framework of Containerized CPA (CPAC)
7.3.3 Summary
7.4 Rapid Construction Scheme of CPAs (RCSCPA) Based on Docker and Kubernetes
7.4.1 Background and Motivation
7.4.2 System Architecture of RCSCPA
7.4.3 Core Functional Mechanisms of RCSCPA
7.4.3.1 Horizontal Auto‐Scaling Mechanism
7.4.3.2 Load Balance Mechanism
7.4.3.3 Failover Mechanism
7.4.4 Industrial Case Study of RCSCPA. 7.4.4.1 Experimental Setup
7.4.4.2 Testing Results
7.4.5 Summary
7.5 Big Data Analytics Application Platform
7.5.1 Architecture of Big Data Analytics Application Platform
7.5.2 Performance Evaluation of Processing Big Data
7.5.3 Big Data Analytics Application in Manufacturing – Electrical Discharge Machining
7.5.4 Summary
7.6 Manufacturing Services Automated Construction Scheme (MSACS)
7.6.1 Background and Motivation
7.6.2 Design of Three‐Phase Workflow of MSACS
7.6.3 Architecture Design of MSACS
7.6.4 Designs of Core Components
7.6.4.1 Design of Key Information (KI) Extractor
7.6.4.2 Design of Library Information (Lib. Info.) Template
7.6.4.3 Design of Service Interface Information (SI Info.) Template
7.6.4.4 Design of Web Service Package (WSP) Generator
7.6.4.5 Design of Service Constructor
7.6.5 Industrial Case Studies
7.6.5.1 Web Graphical User Interface (GUI) of MSACS
7.6.5.2 Case Study 1: Automated Construction of the AVM Cloud‐based Manufacturing (CMfg) Service for Validating the Efficacy of MSACS
7.6.5.3 Case Study 2: Performance Evaluation of MSACS
7.6.6 Summary
7.7 Containerized MSACS (MSACSC)
7.8 Conclusion
Appendix 7.A ‐ Abbreviation List
Appendix 7.B ‐ Patents (AMCoT + CPA)
References
8 Automatic Virtual Metrology (AVM)
8.1 Introduction
8.1.1 Survey of Virtual Metrology (VM)‐Related Literature
8.1.2 Necessity of Applying VM
8.1.3 Benefits of VM
8.2 Evolution of VM and Invention of AVM
8.2.1 Invention of AVM
8.3 Integrating AVM Functions into the Manufacturing Execution System (MES)
8.3.1 Operating Scenarios among AVM, MES Components, and Run‐to‐Run (R2R) Controllers
8.4 Applying AVM for Workpiece‐to‐Workpiece (W2W) Control
8.4.1 Background Materials
8.4.2 Fundamentals of Applying AVM for W2W Control
8.4.3 R2R Control Utilizing VM with Reliance Index (RI) and Global Similarity Index (GSI)
8.4.4 Illustrative Examples
8.4.5 Summary
8.5 AVM System Deployment
8.5.1 Automation Levels of VM Systems
8.5.2 Deployment of the AVM System
8.6 Conclusion
Appendix 8.A – Abbreviation List
Appendix 8.B – List of Symbols in Equations
Appendix 8.C – Patents (AVM)
References
9 Intelligent Predictive Maintenance (IPM)
9.1 Introduction
9.1.1 Necessity of Baseline Predictive Maintenance (BPM)
9.1.2 Prediction Algorithms of Remaining Useful Life (RUL)
9.1.3 Introducing the Factory‐wide IPM System
9.2 BPM
9.2.1 Important Samples Needed for Creating Target‐Device Baseline Model
9.2.2 Samples Needed for Creating Baseline Individual Similarity Index (ISIB) Model
9.2.3 Device‐Health‐Index (DHI) Module
9.2.4 Baseline‐Error‐Index (BEI) Module
9.2.5 Illustration of Fault‐Detection‐and‐Classification (FDC) Logic
9.2.6 Flow Chart of Baseline FDC Execution Procedure
9.2.7 Exponential‐Curve‐Fitting (ECF) RUL Prediction Module
9.3 Time‐Series‐Prediction (TSP) Algorithm for Calculating RUL
9.3.1 ABPM Scheme
9.3.2 Problems Encountered with the ECF Model
Case A: TD’s Aging Feature Goes Too Smooth
Case B: TD’s Aging Feature Rises or Drops Drastically
9.3.3Details of the TSP Algorithm
9.3.3.1 AR Model
9.3.3.2 MA Model
9.3.3.3 ARMA and ARIMA Models
9.3.3.4 TSP Algorithm
9.3.3.5 Pre‐Alarm Module
9.3.3.6 Death Correlation Index
9.4 Factory‐Wide IPM Management Framework
9.4.1 Management View and Equipment View of a Factory
Management View
Equipment View
9.4.2 Health Index Hierarchy (HIH)
9.4.3 Factory‐wide IPM System Architecture
(i) Concise‐and‐healthy Creation Server (CCS)
(ii) IPM Server
(iii) IPM Manager
(iv) IPM Client
(v) Central Database
9.5 IPM System Implementation Architecture
9.5.1 Implementation Architecture of IPMC based on Docker and Kubernetes
9.5.2 Construction and Implementation of the IPMC
9.6 IPM System Deployment
Step 1: TD Selection and Operation Analysis
Step 2: IPM System Setup
Step 3: Data Collection
Step 4: IPM Modeling
Step 5: IPM Function and Integration Tests
Step 6: System Release
9.7 Conclusion
Appendix 9.A ‐ Abbreviation List
Appendix 9.B – List of Symbols in Equations
Appendix 9.C – Patents (IPM) USA Patents
Taiwan, ROC Patents
Japan Patent
European Patent
China Patents
Korea Patent
References
10 Intelligent Yield Management (IYM)
10.1 Introduction
10.1.1 Traditional Root‐Cause Search Procedure of a Yield Loss
10.1.2 IYM System
10.1.3 Procedure for Finding the Root Causes of a Yield Loss by Applying the Key‐variable Search Algorithm (KSA) Scheme
10.2 KSA Scheme
10.2.1 Data Preprocessing Module
10.2.2 KSA Module
10.2.2.1 Triple Phase Orthogonal Greedy Algorithm (TPOGA)
10.2.2.2 Automated Least Absolute Shrinkage and Selection Operator (ALASSO)
10.2.2.3 Reliance Index of KSA (RIK) Module
10.2.3 Blind‐stage Search Algorithm (BSA) Module
10.2.3.1 Blind Cases
10.2.3.2 Blind‐stage Search Algorithm
10.2.4 Interaction‐Effect Search Algorithm (IESA) Module
10.2.4.1 Interaction‐Effect
10.2.4.2 Interaction‐Effect Search Algorithm
10.3 IYM System Deployment
10.4 Conclusion
Appendix 10.A ‐ Abbreviation List
Appendix 10.B ‐ List of Symbols in Equations
Appendix 10.C ‐ Patents (IYM) USA Patents
Taiwan, ROC Patents
China Patents
Korea Patent
References
11 Application Cases of Intelligent Manufacturing
11.1 Introduction
11.2 Application Case I: Thin Film Transistor Liquid Crystal Display (TFT‐LCD) Industry
11.2.1 Automatic Virtual Metrology (AVM) Deployment Examples in the TFT‐LCD Industry
11.2.1.1 Introducing the TFT‐LCD Production Tools and Manufacturing Processes for AVM Deployment
TFT Process
CF Process
LCD Process
11.2.1.2 AVM Deployment Types for TFT‐LCD Manufacturing
Single‐Stage AVM Deployment [Solid Squares in Figures 11.2b, 11.5b, and 11.7b]
Dual‐Stage VM Deployment [Dotted Square in Figure 11.2b]
Cooperative‐Tools AVM Deployment [Segmented Squares in Figures 11.2b, 11.5b, and 11.7b]
Concept of a Virtual Cassette
11.2.1.3 Illustrative Examples
Single‐Stage Example
Dual‐Stage Example
Cooperative‐Tools Example [Combination Case]
Cooperative‐Tools Example [Inline Case]
11.2.1.4 Summary
11.2.2 Intelligent Yield Management (IYM) Deployment Examples in the TFT‐LCD Industry
11.2.2.1 Introducing the TFT‐LCD Production Tools and Manufacturing Processes for IYM Deployment
11.2.2.2 KSA Deployment Example
11.2.2.3 Summary
11.3 Application Case II: Solar Cell Industry
11.3.1 Introducing the Solar Cell Manufacturing Process and Requirement Analysis of Intelligent Manufacturing
11.3.2 T2T Control with AVM Deployment Examples
11.3.2.1 T2T+VM Control Scheme with RI&GSI
11.3.2.2 Illustrative Examples of T2T Control with AVM
Case 0: VM Accuracy Verification
Case 1: T2T with AM
Case 2: T2T with VM
Case 3: Add Two Bad‐Quality VM Samples & Apply T2T+VM w/o RI&GSI
Case 4: Add Two Bad‐Quality VM Samples & Apply T2T+VM with RI&GSI
11.3.3 Factory‐Wide Intelligent Predictive Maintenance (IPM) Deployment Examples
11.3.3.1 Illustrative Examples of BPM and RUL Prediction
Necessity of Adopting the C&H Samples in the Baseline Model
Illustration of the FDC Portion of the BPM Scheme
Illustration of the RUL Portion of the BPM Scheme
Exponential‐Curve‐Fitting (ECF) Model
Time‐Series‐Prediction (TSP) Algorithm
11.3.3.2 Illustrative Example of Factory‐Wide IPM System
11.3.4 Summary
11.4 Application Case III: Semiconductor Industry
11.4.1 AVM Deployment Example in the Semiconductor Industry
11.4.1.1 AVM Deployment Example of the Etching Process
11.4.1.2 Summary
11.4.2 IPM Deployment Examples in the Semiconductor Industry
11.4.2.1 Introducing the Bumping Production Tools for IPM Deployment
11.4.2.2 Illustrative Example
11.4.2.3 Summary
11.4.3 IYM Deployment Examples in the Semiconductor Industry
11.4.3.1 Introducing the Bumping Process of Semiconductor Manufacturing for IYM Deployment
11.4.3.2 Illustrative Example
Phase I
Phase II
11.4.3.3 Summary
11.5 Application Case IV: Automotive Industry
11.5.1 AMCoT and AVM Deployment Examples in Wheel Machining Automation (WMA)
11.5.1.1 Integrating GED‐plus‐AVM (GAVM) into WMA for Total Inspection
Enhancing GED to Become CPA
11.5.1.2 Applying AMCoT to WMA
11.5.1.3 Applying AVM in AMCoT to WMA
11.5.1.4 Summary
11.5.2 Mass Customization (MC) Example for WMA
11.5.2.1 Requirements of MC Production for WMA
11.5.2.2 Considerations for Applying AVM in MC‐Production of WMA
11.5.2.3 The AVM‐plus‐Target‐Value‐Adjustment (TVA) Scheme for MC
11.5.2.4 AVM‐plus‐TVA Deployment Example for WMA
11.5.2.5 Summary
11.6 Application Case V: Aerospace Industry
11.6.1 Introducing the Engine‐Case (EC) Manufacturing Process
11.6.1.1 Manufacturing Processes of an EC
11.6.1.2 Inspection Processes of the Flange Holes
11.6.1.3 Literature Reviews
11.6.2 Integrating GAVM into EC Manufacturing for Total Inspection
11.6.2.1 Considerations of Applying AVM in EC Manufacturing
11.6.3 The DF Scheme for Estimating the Flange Deformation of an EC
11.6.3.1 Probing Scenario
11.6.3.2 Ellipse‐like Deformation of an EC
Definition of {a, b, h, k, θ}
Relationship between EC Deformation Amount and Approximate Position on the End‐Face
11.6.3.3 Position Error
Genetic Algorithm
Interpolation Fitting
Deformation Fusion
11.6.3.4 Integrating the On‐Line Probing, the DF Scheme, and the AVM Prediction
11.6.4 Illustrative Examples
11.6.4.1 Diameter Prediction
11.6.4.2 Position Prediction
11.6.5 Summary
11.7 Application Case VI: Chemical Industry
11.7.1 Introducing the Carbon‐Fiber Manufacturing Process
11.7.2 Three Preconditions of Applying AVM
11.7.3 Challenges of Applying AVM to Carbon‐Fiber Manufacturing
11.7.3.1 CPA+AVM (CPAVM) Scheme for Carbon‐Fiber Manufacturing
11.7.3.2 AMCoT for Carbon‐Fiber Manufacturing
11.7.4 Illustrative Example
11.7.4.1 Production Data Traceback (PDT) Mechanism for Work‐in‐Process (WIP) Tracking
11.7.4.2 AVM for Carbon‐Fiber Manufacturing
11.7.5 Summary
11.8 Application Case VII: Bottle Industry
11.8.1 Bottle Industry and Its Intelligent Manufacturing Requirements
11.8.1.1 Introducing the Blow‐Molding Manufacturing Process
11.8.2 Applying AVM to Blow Molding Manufacturing Process
11.8.3 AVM‐Based Run‐to‐Run (R2R) Control for Blow Molding Manufacturing Process
11.8.4 Illustrative Example
11.8.5 Summary
Appendix 11.A ‐ Abbreviation List
Appendix 11.B ‐ List of Symbols in Equations
References
Index. a
b
c
d
e
f
g
h
i
j
k
l
m
n
o
p
q
r
s
t
u
v
w
x
y
z
WILEY END USER LICENSE AGREEMENT
Отрывок из книги
IEEE Press 445 Hoes Lane Piscataway, NJ 08854
.....
Since Germany brought up Industry 4.0 in 2012, the trend of Intelligent Manufacturing has boomed globally. By integrating the innovative information‐and‐communication technologies such as IoT, Cloud, Big Data, AI, etc., various Cyber‐Physical Systems are developed to promote factory process optimization, yield improvement, efficiency enhancement, and cost reduction. Besides, in response to changes in consumers' habits, Zero Defects, High Variety Low Volume, and Rapid Change have become mandatory indicators for Intelligent Manufacturing.
Advanced Semiconductor Engineering Inc. (ASE), is the leading provider of independent semiconductor manufacturing services in assembly and test. ASE develops and offers complete turnkey solutions in IC packaging, design and production of interconnect materials, front‐end engineering test, wafer probing, and final test. In 2011, ASE started to vigorously promote Intelligent Manufacturing and established over 15 lights‐out factories in response to changes in the global industrial environment. Moreover, ASE also collaborated with various top universities in Taiwan, ROC for R&D of IoT, Cloud, Big Data, and AI technologies, which have cultivated more than 400 professionals in the automation field via co‐hosting educational trainings and industry programs to improve the automation capability within ASE.
.....