Change Detection and Image Time-Series Analysis 1
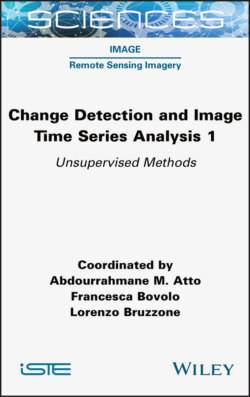
Реклама. ООО «ЛитРес», ИНН: 7719571260.
Оглавление
Группа авторов. Change Detection and Image Time-Series Analysis 1
Table of Contents
List of Illustrations
List of Tables
Guide
Pages
Change Detection and Image Time Series Analysis 1. Unsupervised Methods
Preface
Volume 1: Unsupervised methods
Volume 2: Supervised methods
List of Notations
1. Unsupervised Change Detection in Multitemporal Remote Sensing Images
1.1. Introduction
1.2. Unsupervised change detection in multispectral images. 1.2.1. Related concepts
1.2.2. Open issues and challenges
1.2.3. Spectral–spatial unsupervised CD techniques
1.3. Unsupervised multiclass change detection approaches based on modeling spectral–spatial information. 1.3.1. Sequential spectral change vector analysis (S2CVA)
1.3.2. Multiscale morphological compressed change vector analysis
1.3.2.1. SCV reconstruction based on multiscale morphological processing
1.3.2.2. Multiscale change information ensemble
1.3.2.3. Multiclass change representation and discrimination
1.3.3. Superpixel-level compressed change vector analysis
1.3.3.1. Superpixel-level spectral change representation
1.3.3.2. Determination of the optimal segmentation scale
1.3.3.3. Decision fusion-based CD
1.4. Dataset description and experimental setup. 1.4.1. Dataset description
1.4.2. Experimental setup
1.5. Results and discussion. 1.5.1. Results on the Xuzhou dataset
1.5.2. Results on the Indonesia tsunami dataset
1.6. Conclusion
1.7. Acknowledgements
1.8. References
2. Change Detection in Time Series of Polarimetric SAR Images
2.1. Introduction
2.1.1. The problem
2.1.2. Important concepts illustrated by means of the gamma distribution
2.2. Test theory and matrix ordering
2.2.1. Test for equality of two complex Wishart distributions
2.2.2. Test for equality of k-complex Wishart distributions
2.2.3. The block diagonal case
2.2.4. The Loewner order
2.3. The basic change detection algorithm
2.4. Applications
2.4.1. Visualizing changes
2.4.2. Fieldwise change detection
2.4.3. Directional changes using the Loewner ordering
2.4.4. Software availability
2.5. References
3. An Overview of Covariance-based Change Detection Methodologies in Multivariate SAR Image Time Series
3.1. Introduction
3.2. Dataset description
3.3. Statistical modeling of SAR images. 3.3.1. The data
3.3.2. Gaussian model
3.3.3. Non-Gaussian modeling
3.4. Dissimilarity measures. 3.4.1. Problem formulation
3.4.2. Hypothesis testing statistics
3.4.2.1. Gaussian assumption
3.4.2.2. Non-Gaussian assumption
3.4.3. Information-theoretic measures
3.4.4. Riemannian geometry distances
3.4.5. Optimal transport
3.4.6. Summary
3.4.7. Results of change detectors on the UAVSAR dataset
3.5. Change detection based on structured covariances
3.5.1. Low-rank Gaussian change detector
3.5.2. Low-rank compound Gaussian change detector
3.5.2.1. Maximum likelihood under H1
Algorithm 3.1.– MLE under H1 and fixed t
3.5.2.2. Maximum likelihood under H0
Algorithm 3.2.– MLE under H0
3.5.3. Results of low-rank change detectors on the UAVSAR dataset
3.6. Conclusion
3.7. References
4. Unsupervised Functional Information Clustering in Extreme Environments from Filter Banks and Relative Entropy
4.1. Introduction
4.2. Parametric modeling of convnet features
4.3. Anomaly detection in image time series
4.4. Functional image time series clustering
4.5. Conclusion
4.6. References
5. Thresholds and Distances to Better Detect Wet Snow over Mountains with Sentinel-1 Image Time Series
5.1. Introduction
5.2. Test area and data
5.3. Wet snow detection using Sentinel-1
5.4. Metrics to detect wet snow
5.5. Discussion
5.6. Conclusion
5.7. Acknowledgements
5.8. References
6. Fractional Field Image Time Series Modeling and Application to Cyclone Tracking
6.1. Introduction
6.2. Random field model of a cyclone texture
6.2.1. Cyclone texture feature
6.2.2. Wavelet-based power spectral densities and cyclone fields
6.2.3. Fractional spectral power decay model
6.3. Cyclone field eye detection and tracking. 6.3.1. Cyclone eye detection
6.3.2. Dynamic fractal field eye tracking
6.4. Cyclone field intensity evolution prediction
6.5. Discussion
6.6. Acknowledgements
6.7. References
7. Graph of Characteristic Points for Texture Tracking: Application to Change Detection and Glacier Flow Measurement from SAR Images
7.1. Introduction
7.2. Texture representation and characterization using local extrema. 7.2.1. Motivation and approach
7.2.2. Local extrema keypoints within SAR images
7.3. Unsupervised change detection. 7.3.1. Proposed framework
7.3.2. Weighted graph construction from keypoints
7.3.3. Change measure (CM) generation
7.4. Experimental study
7.4.1. Data description and evaluation criteria. 7.4.1.1. Data description
7.4.1.2. Evaluation criteria
7.4.2. Change detection results
7.4.2.1. Results for Dataset 1
7.4.2.2. Results for Dataset 2
7.4.3. Sensitivity to parameters
7.4.3.1. Sensitivity to the keypoint density
7.4.3.2. Sensitivity to K and wp
7.4.4. Comparison with the NLM model
7.4.5. Analysis of the algorithm complexity
7.5. Application to glacier flow measurement
7.5.1. Proposed method
7.5.2. Results
7.6. Conclusion
7.7. References
8. Multitemporal Analysis of Sentinel-1/2 Images for Land Use Monitoring at Regional Scale
8.1. Introduction
8.2. Proposed method
8.2.1. Test site and data
8.3. SAR processing
8.4. Optical processing
8.5. Combination layer
8.6. Results
8.7. Conclusion
8.8. References
9. Statistical Difference Models for Change Detection in Multispectral Images
9.1. Introduction
9.2. Overview of the change detection problem
9.2.1. Change detection methods for multispectral images
9.2.1.1. Mid-/high-spatial-resolution images
9.2.1.2. Very high-spatial-resolution images
9.2.2. Challenges addressed in this chapter
9.3. The Rayleigh–Rice mixture model for the magnitude of the difference image
9.3.1. Magnitude image statistical mixture model
9.3.2. Bayesian decision
9.3.3. Numerical approach to parameter estimation
9.3.3.1. EM algorithm
9.3.3.2. Iterative equations
9.3.3.3. Initialization
9.4. A compound multiclass statistical model of the difference image
9.4.1. Difference image statistical mixture model
9.4.1.1. Mathematical formulation
9.4.1.2. Physical interpretation
9.4.2. Magnitude image statistical mixture model
9.4.3. Bayesian decision
9.4.4. Numerical approach to parameter estimation
9.4.4.1. Model assumptions
9.4.4.2. EM algorithm
9.4.4.3. Iterative equations
9.4.4.4. Initialization
9.5. Experimental results
9.5.1. Dataset description
Dataset A
Dataset B
Dataset C
Dataset D
9.5.2. Experimental setup
9.5.3. Test 1: Two-class Rayleigh–Rice mixture model. 9.5.3.1. Test description
Rayleigh–Rice mixture (rR)
Gaussian mixture (GG)
Rician mixture with common scale parameter (MF)
9.5.3.2. EM algorithm and fitting performance
9.5.3.3. Change detection results
9.5.4. Test 2: Multiclass Rician mixture model. 9.5.4.1. Test description
Rayleigh-Rice mixture (rR)
Multiclass Rician mixture (rrR)
9.5.4.2. EM algorithm and fitting performance
9.5.4.3. Change detection results
9.6. Conclusion
9.7. References
List of Authors
Index
Summary of Volume 2
WILEY END USER LICENSE AGREEMENT
Отрывок из книги
Image, Field Director – Laure Blanc-Feraud
.....
From the detailed quantitative and qualitative analyses based on the obtained CD accuracy and error indices (see Table 1.2) and the obtained CD maps (see Figure 1.10), it can be observed that the proposed M2C2VA and SPC2VA approaches resulted in higher performance than the reference methods, with respect to the higher OA and Kappa values and smaller detection errors (see Table 1.2). In particular, SPC2VA achieved the highest accuracy (i.e. OA = 92.74% and Kappa = 0.7464), outperforming the other methods. Improvement can also be observed from the obtained change maps by comparing them with the reference map; the identified change targets are more accurate in the two proposed approaches (i.e. Figure 1.10(c) and (d)) than those in the two reference methods (i.e. Figure 1.10(a) and (b)). In two reference methods, S2CVA showed better performance than IR-MAD. As for the computational cost, the proposed SPC2VA approach exhibited efficient performance, which consumed less time than the proposed M2C2VA and IR-MAD, and similar or slightly more time than S2CVA (i.e. 8.91 s vs. 7.12 s), but yielding a significantly higher OA value (i.e. 92.74% vs. 87.74%).
Table 1.2. Multiclass CD results obtained by the proposed and reference methods (Xuzhou dataset)
.....