Reservoir Characterization
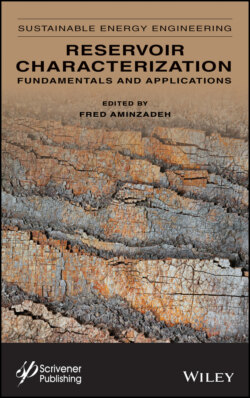
Реклама. ООО «ЛитРес», ИНН: 7719571260.
Оглавление
Группа авторов. Reservoir Characterization
Table of Contents
List of Illustrations
Tables
Guide
Pages
Reservoir Characterization. Fundamentals and Applications
Foreword
Preface
1. Reservoir Characterization: Fundamental and Applications - An Overview
1.1 Introduction to Reservoir Characterization?
1.2 Data Requirements for Reservoir Characterization
1.3 SURE Challenge
1.4 Reservoir Characterization in the Exploration, Development and Production Phases
1.4.1 Exploration Stage/Development Stage
1.4.2 Primary Production Stage
1.4.3 Secondary/Tertiary Production Stage
1.5 Dynamic Reservoir Characterization (DRC)
1.5.1 4D Seismic for DRC
1.5.2 Microseismic Data for DRC
1.6 More on Reservoir Characterization and Reservoir Modeling for Reservoir Simulation
1.6.1 Rock Physics
1.6.2 Reservoir Modeling
1.7 Conclusion
References
2. A Comparison Between Estimated Shear Wave Velocity and Elastic Modulus by Empirical Equations and that of Laboratory Measurements at Reservoir Pressure Condition
2.1 Introduction
2.2 Methodology. 2.1.2 Estimating the Shear Wave Velocity
2.2.2 Estimating Geomechanical Parameters
2.3 Laboratory Set Up and Measurements
2.3.1 Laboratory Data Collection
2.4 Results and Discussion
2.5 Conclusions
2.6 Acknowledgment
References
3. Anomaly Detection within Homogenous Geologic Area
3.1 Introduction
3.2 Anomaly Detection Methodology
3.3 Basic Anomaly Detection Classifiers
3.4 Prior and Posterior Characteristics of Anomaly Detection Performance
3.5 ROC Curve Analysis
3.6 Optimization of Aggregated AD Classifier Using Part of the Anomaly Identified by Universal Classifiers
3.7 Bootstrap Based Tests of Anomaly Type Hypothesis
3.8 Conclusion
References
4. Characterization of Carbonate Source-Derived Hydrocarbons Using Advanced Geochemical Technologies
4.1 Introduction
4.2 Samples and Analyses Performed
4.3 Results and Discussions
4.4 Summary and Conclusions
References
5. Strategies in High-Data-Rate MWD Mud Pulse Telemetry
5.1 Summary
5.1.1 High Data Rates and Energy Sustainability
5.1.2 Introduction
5.1.3 MWD Telemetry Basics
5.1.4 New Telemetry Approach
5.2 New Technology Elements
5.2.1 Downhole Source and Signal Optimization
5.2.2 Surface Signal Processing and Noise Removal
5.2.3 Pressure, Torque and Erosion Computer Modeling
5.2.4 Wind Tunnel Analysis: Studying New Approaches
5.2.5 Example Test Results
5.3 Directional Wave Filtering
5.3.1 Background Remarks
5.3.2 Theory
5.3.3 Calculations
5.4 Conclusions
Acknowledgments
References
6. Detection of Geologic Anomalies with Monte Carlo Clustering Assemblies
6.1 Introduction
6.2 Analysis of Inhomogeneity of the Training and Test Sets and Instability of Clustering
6.3 Formation of Multiple Randomized Test Sets and Construction of the Clustering Assemblies
6.4 Irregularity Index of Individual Clusters in the Cluster Set
6.5 Anomaly Indexes of Individual Records and Clustering Assemblies
6.6 Prior and Posterior True and False Discovery Rates for Anomalous and Regular Records
6.7 Estimates of Prior False Discovery Rates for Anomalous Cluster Sets, Clusters, and Individual Records. Permeability Dataset
6.8 Posterior Analysis of Efficiency of Anomaly Identification. High Permeability Anomaly
6.9 Identification of Records in the Gas Sand Dataset as Anomalous, using Brine Sand Dataset as Data with Regular Records
6.10 Notations
6.11 Conclusions
References
7. Dissimilarity Analysis of Petrophysical Parameters as Gas-Sand Predictors
7.1 Introduction
7.2 Petrophysical Parameters for Gas-Sand Identification
7.3 Lithologic and Fluid Content Dissimilarities of Values of Petrophysical Parameters
7.4 Parameter Ranking and Efficiency of Identification of Gas-Sands
7.5 ROC Curve Analysis with Cross Validation
7.6 Ranking Parameters According to AUC Values
7.7 Classification with Multidimensional Parameters as Gas Predictors
7.8 Conclusions
Definitions and Notations
References
8. Use of Type Curve for Analyzing Non-Newtonian Fluid Flow Tests Distorted by Wellbore Storage Effects
8.1 Introduction
8.2 Objective
8.3 Problem Analysis
8.3.1 Model Assumptions
8.3.2 Solution Without the Wellbore Storage Distortion
8.3.3 Wellbore Storage and Skin Effects
8.3.4 Solution by Mathematical Inspection
8.3.5 Solution Verification
8.4 Use of Finite Element
8.5 Analysis Methodology
8.5.1 Finding the n Value
8.5.2 Dimensionless Wellbore Storage
8.5.3 Use of Type Curves
8.5.4 Match Point
8.5.5 Uncertainty in Analysis
8.6 Test Data Examples
8.6.1 Match Point
8.6.2 Match Point
8.6.3 Analysis Recommendations
8.6.4 Match Point
8.6.5 Analysis Recommendations
8.6.6 Match Point
8.7 Conclusion
Nomenclature
References
Appendix A: Non-Linear Boundary Condition and Laplace Transform
Appendix B: Type Curve Charts for Various Power Law Indices
9. Permeability Prediction Using Machine Learning, Exponential, Multiplicative, and Hybrid Models
9.1 Introduction
9.2 Additive, Multiplicative, Exponential, and Hybrid Permeability Models
9.3 Combination of Basis Function Expansion and Exhaustive Search for Optimum Subset of Predictors
9.4 Outliers in the Forecasts Produced with Four Permeability Models
9.5 Additive, Multiplicative, and Exponential Committee Machines
9.6 Permeability Forecast with First Level Committee Machines. Sandstone Dataset
9.7 Permeability Prediction with First Level Committee Machines. Carbonate Reservoirs
9.8 Analysis of Accuracy of Outlier Replacement by The First and Second Level Committee Machines. Sandstone Dataset
9.9 Conclusion
Notations and Definitions
References
10. Geological and Geophysical Criteria for Identifying Zones of High Gas Permeability of Coals (Using the Example of Kuzbass CBM Deposits)
10.1 Introduction
10.2 Physical Properties and External Load Conditions on a Coal Reservoir
10.3 Basis for Evaluating Physical and Mechanical Coalbed Properties in the Borehole Environment
10.4 Conclusions
Acknowledgement
References
11. Rock Permeability Forecasts Using Machine Learning and Monte Carlo Committee Machines
11.1 Introduction
11.2 Monte Carlo Cross Validation and Monte Carlo Committee Machines
11.3 Performance of Extended MC Cross Validation and Construction MC Committee Machines
11.4 Parameters of Distribution of the Number of Individual Forecasts in Monte Carlo Cross Validation
11.5 Linear Regression Permeability Forecast with Empirical Permeability Models
11.6 Accuracy of the Forecasts with Machine Learning Methods
11.7 Analysis of Instability of the Forecast
11.8 Enhancement of Stability of the MC Committee Machines Forecast Via Increase of the Number of Individual Forecasts
11.9 Conclusions
Nomenclature
Appendix 1 - Description of Permeability Models from Different Fields
Appendix 2-A Brief Overview of Modular Networks or Committee Machines⋆
References
12. The Gulf of Mexico Petroleum System – Foundation for Science-Based Decision Making
Introduction
Basin Development and Geologic Overview
Petroleum System
Reservoir Geology
Hydrocarbons
Salt and Structure
Conclusions
Acknowledgments and Disclaimer
References
13. Forecast and Uncertainty Analysis of Production Decline Trends with Bootstrap and Monte Carlo Modeling
13.1 Introduction
13.2 Simulated Decline Curves
13.3 Nonlinear Least Squares for Decline Curve Approximation
13.4 New Method of Grid Search for Approximation and Forecast of Decline Curves
13.5 Iterative Minimization of Least Squares with Multiple Approximating Models
13.6 Grid Search Followed by Iterative Minimization with Levenberg-Marquardt Algorithm
13.7 Two Methods for Aggregated Forecast and Analysis of Forecast Uncertainty
13.8 Uncertainty Quantile Ranges Obtained Using Monte Carlo and Bootstrap Methods
13.9 Monte Carlo Forecast and Analysis of Forecast Uncertainty
13.10 Block Bootstrap Forecast and Analysis of Forecast Uncertainty
13.11 Comparative Analysis of Results of Monte Carlo and Bootstrap Simulations
13.12 Conclusions
References
14. Oil and Gas Company Production, Reserves, and Valuation
14.1 Introduction
14.2 Reserves. 14.2.1 Proved Reserves
14.2.2 Proved Reserves Categories
14.2.3 Reserves Reporting
14.2.4 Probable and Possible Reserves
14.2.5 Contractual Differences
14.3 Production
14.4 Factors that Impact Company Value. 14.4.1 Ownership
14.4.1.1 International Oil Companies
14.4.1.2 National Oil Companies
14.4.1.3 Government Sponsored Entities
14.4.1.4 Independents and Juniors
14.4.2 Degree of Integration
14.4.3 Product Mix
14.4.4 Commodity Price
14.4.5 Production Cost
14.4.6 Finding Cost
14.4.7 Assets
14.4.8 Capital Structure
14.4.9 Geologic Diversification
14.4.10 Geographic Diversification
14.4.11 Unobservable Factors
14.5 Summary Statistics. 14.5.1 Sample
14.5.2 Variables
14.5.3 Data Source
14.5.4 International Oil Companies
14.5.5 Independents
14.6 Market Capitalization. 14.6.1 Functional Specification
14.6.2 Expectations
14.7 International Oil Companies
14.8 U.S. Independents. 14.8.1 Large vs. Small Cap, Oil vs. Gas
14.8.2 Consolidated Small-Caps
14.8.3 Multinational vs. Domestic
14.8.4 Conventional vs. Unconventional
14.8.5 Production and Reserves
14.8.6 Regression Models
14.9 Private Companies
14.10 National Oil Companies of OPEC
14.11 Government Sponsored Enterprises and Other International Companies
14.12 Conclusions
References
15. An Analytical Thermal-Model for Optimization of Gas-Drilling in Unconventional Tight-Sand Reservoirs
15.1 Introduction
15.2 Mathematical Model
15.3 Model Comparison
15.4 Sensitivity Analysis
15.5 Model Applications
15.6 Conclusions
Nomenclature
Acknowledgements
References
Appendix A: Steady Heat Transfer Solution for Fluid Temperature in Counter-Current Flow. Assumptions
Governing Equation
Boundary Conditions
Solution
16. Development of an Analytical Model for Predicting the Fluid Temperature Profile in Drilling Gas Hydrates Reservoirs
16.1 Introduction
16.2 Mathematical Model
16.3 Case Study
16.4 Sensitivity Analysis
16.5 Conclusions
Acknowledgements
Nomenclature
References
17. Distinguishing Between Brine-Saturated and Gas-Saturated Shaly Formations with a Monte-Carlo Simulation of Seismic Velocities
17.1 Introduction
17.2 Random Models for Seismic Velocities
17.3 Variability of Seismic Velocities Predicted by Random Models
17.4 The Separability of (Vp, Vs) Clusters for Gas and Brine-Saturated Formations
17.5 Reliability Analysis of Identifying Gas-Filled Formations
17.5.1 Classification with K-Nearest Neighbor
17.5.2 Classification with Recursive Partitioning
17.5.3 Classification with Linear Discriminant Analysis
17.5.4 Comparison of the Three Classification Techniques
17.6 Conclusions
References
18. Shale Mechanical Properties Influence Factors Overview and Experimental Investigation on Water Content Effects
18.1 Introduction
18.2 Influence Factors
18.2.1. Effective Pressure
18.2.2 Porosity
18.2.3 Water Content
18.2.4 Salt Solutions
18.2.5 Total Organic Carbon (TOC)
18.2.6 Clay Content
18.2.7 Bedding Plane Orientation
18.2.8 Mineralogy
18.2.9 Anisotropy
18.2.10 Temperature
18.3 Experimental Investigation of Water Saturation Effects on Shale’s Mechanical Properties. 18.3.1 Experiment Description
18.3.2 Results and Discussion
18.3.3 Error Analysis of Experiments
18.4 Conclusions
Acknowledgements
References
19. A Numerical Investigation of Enhanced Oil Recovery Using Hydrophilic Nanofuids
19.1. Introduction
19.2 Simulation Framework. 19.2.1 Background
19.2.2 Two Essential Computational Components. 19.2.2.1 Flow Model
19.2.2.2 Nanoparticle Transport and Retention Model
19.3 Coupling of Mathematical Models
19.4 Verification Cases
19.4.1 Effect of Time Steps on the Performance of the in House Simulator
19.4.2 Comparison with Eclipse
19.4.3 Comparison with Software MNM1D
19.5 Results
19.5.1 Continuous Injection
19.5.1.1 Effect of Injection Time on Oil Recovery and Nanoparticle Adsorption
19.5.1.2 Effect of Injection Rate on Oil Recovery and Nanoparticle Adsorption
19.5.2 Slug Injection
19.5.2.1 Effect of Injection Time on Oil Recovery and Nanoparticle Adsorption
19.5.2.2 Effect of Slug Size on Oil Recovery and Nanoparticle Adsorption
19.5.3 Water Postflush
19.5.3.1 Effect of Injection Time Length
19.5.3.2 Effect of Flow Rate Ratio Between Water and Nanofuids on Oil and Nanoparticle Recovery
19.5.4 3D Model Showcase
19.6 Discussions
19.7 Conclusions and Future Work
References
20. 3D Seismic-Assisted CO2-EOR Flow Simulation for the Tensleep Formation at Teapot Dome, USA
20.1 Presentation Sequence
20.2 Introduction
20.3 Geological Background
20.4 Discrete Fracture Network (DFN)
20.5 Petrophysical Modeling
20.6 PVT Analysis
20.7 Streamline Analysis
20.8 CO2-EOR
20.9 Conclusions
Acknowledgement
References
21. Application of Machine Learning in Reservoir Characterization
21.1 Brief Introduction to Reservoir Characterization
21.2 Artificial Intelligence and Machine (Deep) Learning Review
21.2.1 Support Vector Machines
21.2.2 Clustering (Unsupervised Classification)
21.2.3 Ensemble Methods
21.2.4 Artificial Neural Networks (ANN)-Based Methods
21.3 Artificial Intelligence and Machine (Deep) Learning Applications to Reservoir Characterization
21.3.1 3D Structural Model Development
21.3.2 Sedimentary Modeling
21.3.3. 3D Petrophysical Modeling
21.3.4. Dynamic Modeling and Simulations
21.4 Machine (Deep) Learning and Enhanced Oil Recovery (EOR)
21.4.1 ANNs for EOR Performance and Economics
21.4.2 ANNs for EOR Screening
21.5 Conclusion
Acknowledgement
References
Index
Check out these other related titles from Scrivener Publishing
WILEY END USER LICENSE AGREEMENT
Отрывок из книги
Scrivener Publishing
100 Cummings Center, Suite 541J
.....
Figure 1.9 Reservoir modeling process workflow. The process takes control of the data within its modeling framework and integrates the various types of data attributes. Courtesy: Roxar-Emerson.
.....