Predicting Heart Failure
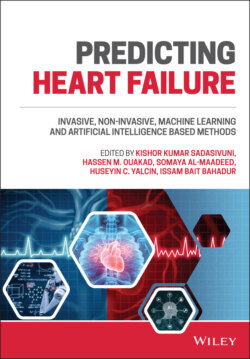
Реклама. ООО «ЛитРес», ИНН: 7719571260.
Оглавление
Группа авторов. Predicting Heart Failure
Predicting Heart Failure. Invasive, Non-Invasive, Machine Learning, and Artificial Intelligence Based Methods
Contents
List of Illustrations
List of Tables
Guide
Pages
Preface
Abbreviations
Acknowledgment
1 Invasive, Non-Invasive, Machine Learning, and Artificial Intelligence Based Methods for Prediction of Heart Failure
1.1 Introduction
1.2 Heart Failure
1.2.1 What is HF?
1.2.2 Causes of HF
1.2.3 What are the Symptoms of HF?
1.2.4 HF Treatment
1.3 Invasive and Non-Invasive Procedures
1.3.1 What are Invasive and Non-Invasive Procedures?
1.3.2 Diagnosis by Invasive Procedures
1.3.3 Diagnosis by Non-Invasive Procedures
1.4 Computer-Aided Diagnosis
1.4.1 Clinical Decision Support Systems
1.5 Diagnosis with Artificial Intelligence Methods
1.5.1 Introduction to Artificial Intelligence
1.5.1.1 Expert Systems
1.5.1.2 Machine Learning
1.5.1.3 Deep Learning
1.5.1.4 Image Processing
1.5.2 Artificial Intelligence Supported HF Diagnostic Studies
1.6 Machine Learning Supported Diagnosis
1.6.1 Introduction to Machine Learning
1.6.2 Machine Learning Algorithms
1.6.2.1 Supervised Learning
1.6.2.1.1 Decision Trees
1.6.2.1.2 Naive Bayes
1.6.2.1.3 Support Vector Machines
1.6.2.1.4 K-Nearest Neighbor
1.6.2.1.5 Neural Nets
1.6.2.2 Unsupervised Learning
1.6.2.2.1 K-Means
1.6.2.2.2 Apriori Algorithm
1.6.3 Machine Learning Supported HF Studies
1.7 Conclusion and Future Directions
References
2 Conventional Clinical Methods for Predicting Heart Disease
2.1 Introduction
2.2 Physical Assessments
2.2.1 Inspection Examination
2.2.2 Palpation Examination
2.2.3 Auscultation Examination
2.2.4 Electrocardiogram (ECG)
2.2.5 Treadmill Test
2.2.6 Cardiac Biomarker Examination
2.2.7 Chest Roentgenography
2.3 Physiological Values and Characteristics Considered for Clinical Examination
2.3.1 Exercise Capacity
2.3.2 Chest Pain or Discomfort
2.3.3 Palpitations
2.3.4 Dyspnea, Orthopnea, and Paroxysmal Nocturnal Dyspnea
2.3.5 Claudication
2.3.6 Recording History of Heart Disease
2.3.7 Family History
2.3.8 Blood Pressure and Pulse
2.3.9 Total Cholesterol, Triglycerides, and Associated Lipid Profiles
2.3.10 Bruits and Hums of the Head and Neck
2.3.11 Jugular Venous Pressure
2.3.12 Carotid Pulse and Precordial Impulse
2.3.13 Heart Sounds and Murmurs
2.4 Evolution of the Devices for Conventional Clinical Cardiac Examination
2.4.1 Stethoscope
2.4.2 Electrocardiogram
2.4.3 Echocardiogram (Ultrasound Devices)
2.5 Artificial Intelligence for Assisting Clinical Cardiac Examination
2.5.1 AI in Echocardiography
2.5.2 AI in Electrocardiography
2.5.3 AI in CT
2.6 Conclusion
References
3 Types of Biosensors and their Importance in Cardiovascular Applications
3.1 Introduction
3.2 Specific Biomarkers for Cardiovascular Research
3.3 Types of Biosensors for Cardiovascular Biomarkers Detection. 3.3.1 Electrochemical Biosensors
3.3.2 Piezoelectric Biosensors
3.3.3 Thermal/Thermometric Biosensors
3.3.4 Optical Biosensors
3.3.5 Wearable/Implantable Biosensors
3.4 Applications of Biosensors for Cardiovascular Research
3.5 Future Outlook
3.6 Conclusion
References
4 Overview and Challenges of Wireless Communication and Power Transfer for Implanted Sensors
4.1 Introduction
4.2 Microchip Insertion Procedure
4.3 Wireless Power Transmission
4.3.1 Categories of Wireless Electricity Transmission
4.3.2 Microwave Wireless Power Transfer
4.3.3 Electromagnetic Induction Energy Transfer
4.3.4 Laser Wireless Power Transmission
4.3.5 Ultrasonic Wireless Power Transfer
4.4 The High-Efficiency Technique to Wirelessly Power Up Implanted Sensors
4.4.1 Inductive Wireless Electricity Transfer and Data Transmission through a Common Inductive Coupling
4.4.2 External Reader Unit
4.4.2.1 High-Frequency Demodulation
4.4.3 Microchip Implant
4.4.4 Data Transmission through a Common Inductive Connection
4.4.5 Discussion and Results
4.5 Study and Realization of Ambient RF Energy Harvesting for Invasive Charging
4.5.1 Antenna Design and Simulation
4.5.2 Design a Full-Wave Bridge Rectenna
4.6 Conclusion
References
5 Minimally Invasive and Non-Invasive Sensor Technologies for Predicting Heart Failure An Overview
5.1 Introduction
5.2 Methods
5.2.1 Body Weight Measurement
5.2.2 Electrocardiographic (ECG) Measurement
5.2.3 Bioimpedance Monitoring
5.2.4 Pedometers and Activity Trackers
5.2.5 Implanted Pressure Sensors
5.2.6 Lung Ultrasound
5.2.7 Sound Sensors
5.2.8 Seismocardiography
5.2.9 Ballistocardiography
5.2.10 Photoplethysmography
5.2.11 Monitoring Natriuretic Peptides Levels
5.3 Clinical Trials on Remote Monitoring Using Non-Invasive Sensors
5.4 Discussion
5.5 Conclusion
Acknowledgments
References
6 Artificial Intelligence Techniques in Cardiology An Overview
6.1 Introduction
6.2 Artificial Intelligence Techniques. 6.2.1 Artificial Intelligence and Statistical Models
6.2.2 Machine Learning
6.2.2.1 Supervised Learning
6.2.2.1.1 Classification
6.2.2.1.2 Regression
6.2.2.1.3 Examples of Supervised Learning in Cardiology
6.2.2.2 Unsupervised Learning
6.2.2.2.1 Examples of Unsupervised Learning in Cardiology
6.2.2.3 Semi-Supervised Learning
6.2.2.3.1 Examples of Semi-Supervised Learning in Cardiology
6.2.2.4 Reinforcement Learning
6.2.3 Deep Learning
6.2.3.1 Examples of Deep Learning in Cardiology
6.2.4 Natural Language Processing
6.3 Steps in Building an AI Algorithm
6.4 Limitations, Challenges, and Opportunities
6.4.1 Limitations of Existing Studies
6.4.2 Pitfalls with the Technology
6.4.3 Challenges in Clinical Translation
6.5 Conclusions
References
7 Utilizing Data Mining Classification Algorithms for Early Diagnosis of Heart Diseases
7.1 Introduction
7.2 Previous Studies
7.3 Choice of Machine Learning Algorithms
7.3.1 Decision Tree
7.3.2 Naive Bayes
7.3.3 K-Nearest Neighbor
7.3.4 Random Forest
7.3.5 Support Vector Machine
7.4 Research Methodology
7.4.1 Data Sets Description
7.4.2 Data Preprocessing
7.4.3 Performance Measures
7.5 Results
7.6 Conclusion and Future Work
Acknowledgment
References
8 Applications of Machine Learning for Predicting Heart Failure
8.1 Introduction
8.2 Brief Explanation of ML Techniques for Medicine
8.3 Detection and Diagnosis of HF
8.4 Prediction of HF
8.5 ML Techniques for Detection and Diagnosis of HF
8.6 HF Diagnosis Using Biopsy Images
8.7 HF Case Identification from EHR Data
8.8 ML Techniques for Predicting HF
8.8.1 Prediction of In-Hospital Mortality
8.8.2 Prediction of 30-Day HF Readmission
8.9 Future Direction
8.10 Conclusion
Acknowledgments
References
9 Machine Learning Techniques for Predicting and Managing Heart Failure
9.1 State of the Art of Machine Learning Applications in HF
9.1.1 Introduction. 9.1.1.1 Background
9.1.1.2 HF Classifications
9.1.1.3 Evaluation and Diagnosis
9.1.2 Applications of ML in HF. 9.1.2.1 ML in Medicine
9.1.2.2 ML Applications in HF Diagnosis/Prediction
9.1.2.3 ML Applications in Severity Estimation
9.1.2.4 ML Applications in Clinical Prognosis
9.1.2.5 ML Applications in Hospital Readmission Prediction
9.1.2.6 ML Applications in Mortality Prediction
9.1.2.7 ML Applications in Mortality or Readmission Prediction
9.2 Two Prominent Applications
9.2.1 HF Diagnosis. 9.2.1.1 The Data Set
9.2.1.2 Methodology
9.2.1.3 Results
9.2.2 Severity Estimation and Adverse Events Prediction. 9.2.2.1 The Data Set
9.2.2.2 Methodology
9.2.2.3 Results for HF Severity Estimation
9.2.2.4 Results for the Prediction of Adverse Events
9.3 Conclusions
References
10 Clinical Applications of Artificial Intelligence in Early and Accurate Detection of Low-Concentration CVD Biomarkers
10.1 Introduction
10.2 Risk Assessment and Early Detection of CVDs Using Biosensors
10.3 Different Types of Cardiac Biosensors
10.3.1 Optical Biosensors
10.3.2 Electrochemical Biosensors
10.3.2.1 Application of Nanomaterials in Electrochemical Biosensors
10.3.3 Magnetic Biosensors
10.4 Limitations of Biosensor Technology in the Detection of CVDs
10.5 Application of AI in CVD Detection
10.5.1 Telemedicine and Mobile Health
10.5.2 Internet of Things
10.5.3 DL and ML
10.5.4 Magnetic Resonance Imaging
10.5.5 Echocardiography
10.5.6 Cardiac CT
10.6 Conclusions and Future Perspectives
References
11 Commercial Non-Invasive and Invasive Devices for Heart Failure Prediction A Review
11.1 Introduction
11.2 Non-Invasive Devices for Monitoring Heart Failure Patients
11.2.1 Electrocardiography
11.2.1.1 Electrocardiography-Based Monitoring Systems
11.2.2 BP and Heart Failure
11.2.3 Body Weight Monitoring and Heart Failure
11.2.4 Bioimpedance Monitoring and Heart Failure
11.2.5 Smart Wearable and Pocket-Sized Devices for Monitoring of the Heart
11.2.6 Telemonitoring of Heart Failure Using Multiple Commercially Available Non-Invasive Devices
11.2.7 Role of Mobile Health Apps in Heart Failure Self-Care
11.3 Invasive Devices for Monitoring Heart Failure Patients
11.3.1 RV Pressure Monitoring
11.3.2 PA Pressure Sensors
11.3.3 LA Pressure Sensors
11.3.4 Intrathoracic Impedance Monitoring
11.3.5 Commercially Available Devices for Heart Failure Treatment
11.4 Discussion
11.5 Future Outlook
11.6 Conclusion
References
12 Artificial Intelligence Based Commercial Non-Invasive and Invasive Devices for Heart Failure Diagnosis and Prediction
12.1 Introduction
12.2 Search Strategy
12.3 ML Algorithms
12.4 ML and DL Algorithms for Prediction of HF
12.5 Invasive Monitoring Devices for Prediction of HF
12.6 Non-Invasive Wearable Monitoring Devices for Prediction of HF
12.7 Future Outlook on the Use of AI
12.8 Conclusion
Funding Support
References
13 Future Techniques and Perspectives on Implanted and Wearable Heart Failure Detection Devices
13.1 Introduction
13.2 Applications of Implantable Devices in HF Detection
13.3 Wearable Devices in HF Detection Application
13.4 Integrating Wearables Technology into the Healthcare System
13.4.1 Activity Monitors
13.4.2 Heart Rate Monitors
13.4.3 Wearable Devices for HF Prognostic Application
13.4.4 Monitoring Blood Pressure
13.4.5 Arrhythmia Detection
13.4.6 Technology in Development for Early Detection of HF Decompensation
13.4.6.1 Practical Challenges to Wearable Devices
13.5 Summary of the Cardiac and Wearable Device Data Sets
13.6 Machine Learning for Improving Cardiac Health of HF Patients
13.7 Future Directions of Wearables for Early Prediction of HF
Acknowledgment
References
Index
WILEY END USER LICENSE AGREEMENT
Отрывок из книги
Edited by
Kishor Kumar Sadasivuni
.....
There is a need to develop a system in order to collect patient data and make diagnoses based on them. These systems are generally called decision support systems. Decision support systems work by analyzing the data collected from the terminal units in accordance with the needs in the center to support decision making and presenting the results. Decision support systems can sometimes be developed for the field of health and sometimes for another field. Regardless of the field, the task of decision support systems is to infer with the help of the collected data and the rules at hand.
Decision support systems developed specifically for the health field are often referred to as clinical decision support systems. Clinical decision support systems sometimes help experts diagnose disease and recommend treatment methods based on health information. The concept of computer-aided clinical decision making came to the fore many years ago [15]. The working principle of clinical decision support systems is simply as follows: information such as blood values and enzyme values of the patient is obtained, this information is evaluated according to the knowledge base of the health institution, and an inference is made about the patient. When a direct relationship can be established between the inputs and the final decision during inference, this is known as an open box decision support system; when the correct relationship cannot be established between the inputs and the final decision, it is called a closed box decision support system [16].
.....