Smart Systems for Industrial Applications
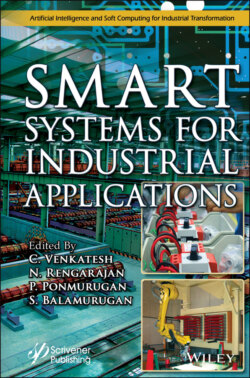
Реклама. ООО «ЛитРес», ИНН: 7719571260.
Оглавление
Группа авторов. Smart Systems for Industrial Applications
Table of Contents
Guide
List of Illustrations
List of Tables
Pages
Smart Systems for Industrial Applications
Preface
1. AI-Driven Information and Communication Technologies, Services, and Applications for Next-Generation Healthcare System
1.1 Introduction: Overview of Communication Technology and Services for Healthcare
1.2 AI-Driven Communication Technology in Healthcare
1.2.1 Technologies Empowering in Healthcare
1.2.2 AI in Diagnosis
1.2.3 Conversion Protocols
1.2.4 AI in Treatment Assistant
1.2.5 AI in the Monitoring Process
1.2.6 Challenges of AI in Healthcare
1.3 AI-Driven mHealth Communication System and Services
1.3.1 Embedding of Handheld Imaging Platforms With mHealth Devices
1.3.2 The Adaptability of POCUS in Telemedicine
1.4 AI-Driven Body Area Network Communication Technologies and Applications
1.4.1 Features
1.4.2 Communication Architecture of Wireless Body Area Networks
1.4.3 Role of AI in WBAN Architecture
1.4.4 Medical Applications
1.4.5 Nonmedical Applications
1.4.6 Challenges
1.5 AI-Driven IoT Device Communication Technologies and Healthcare Applications
1.5.1 AI’s and IoT’s Role in Healthcare
1.5.2 Creating Efficient Communication Framework for Remote Healthcare Management
1.5.3 Developing Autonomous Capability is Key for Remote Healthcare Management
1.5.4 Enabling Data Privacy and Security in the Field of Remote Healthcare Management
1.6 AI-Driven Augmented and Virtual Reality–Based Communication Technologies and Healthcare Applications
1.6.1 Clinical Applications of Communication-Based AI and Augmented Reality
1.6.2 Surgical Applications of Communication-Based on Artificial Intelligence and Augmented Reality
References
2. Pneumatic Position Servo System Using Multi-Variable Multi-Objective Genetic Algorithm–Based Fractional-Order PID Controller
2.1 Introduction
2.2 Pneumatic Servo System
2.3 Existing System Analysis
2.4 Proposed Controller and Its Modeling
2.4.1 Modeling of Fractional-Order PID Controller. 2.4.1.1 Fractional-Order Calculus
2.4.1.2 Fractional-Order PID Controller
2.5 Genetic Algorithm
2.5.1 GA Optimization Methodology
2.5.1.1 Initialization
2.5.1.2 Fitness Function
2.5.1.3 Evaluation and Selection
2.5.1.4 Crossover
2.5.1.5 Mutation
2.5.2 GA Parameter Tuning
2.6 Simulation Results and Discussion. 2.6.1 MATLAB Genetic Algorithm Tool Box
2.6.2 Simulation Results
2.6.2.1 Reference = 500 (Error)
2.6.2.2 Reference = 500
2.6.2.3 Reference = 1,500
2.6.2.4 Analysis Report
2.7 Hardware Results
2.7.1 Reference = 500
2.7.2 Reference = 1,500
2.8 Conclusion
References
3. Improved Weighted Distance Hop Hyperbolic Prediction–Based Reliable Data Dissemination (IWDH-HP-RDD) Mechanism for Smart Vehicular Environments
3.1 Introduction
3.2 Related Work
3.2.1 Extract of the Literature
3.3 Proposed Improved Weighted Distance Hop Hyperbolic Prediction–Based Reliable Data Dissemination (IWDH-HP-RDD) Mechanism for Smart Vehicular Environments
3.4 Simulation Results and Analysis of the Proposed IWDH-HP-RDD Scheme
3.5 Conclusion
References
4. Remaining Useful Life Prediction of Small and Large Signal Analog Circuits Using Filtering Algorithms
4.1 Introduction
4.2 Literature Survey
4.3 System Architecture
4.4 Remaining Useful Life Prediction
4.4.1 Initialization
4.4.2 Proposal Distribution
4.4.3 Time Update
4.4.4 Relative Entropy in Particle Resampling
4.4.5 RUL Prediction
4.5 Results and Discussion
4.6 Conclusion
References
5. AI in Healthcare
5.1 Introduction
5.1.1 What is Artificial Intelligence?
5.1.2 Machine Learning – Neural Networks and Deep Learning
5.1.3 Natural Language Processing
5.2 Need of AI in Electronic Health Record
5.2.1 How Does AI/ML Fit Into EHR?
5.2.2 Natural Language Processing (NLP)
5.2.3 Data Analytics and Representation
5.2.4 Predictive Investigation
5.2.5 Administrative and Security Consistency
5.3 The Trending Role of AI in Pharmaceutical Development
5.3.1 Drug Discovery and Design
5.3.2 Diagnosis of Biomedical and Clinical Data
5.3.3 Rare Diseases and Epidemic Prediction
5.3.4 Applications of AI in Pharma
5.3.5 AI in Marketing
5.3.6 Review of the Companies That Use AI
5.4 AI in Surgery
5.4.1 3D Printing
5.4.2 Stem Cells
5.4.3 Patient Care
5.4.4 Training and Future Surgical Team
5.5 Artificial Intelligence in Medical Imaging
5.5.1 In Cardio Vascular Abnormalities
5.5.2 In Fractures and Musculoskeletal Injuries
5.5.3 In Neurological Diseases and Thoracic Complications
5.5.4 In Detecting Cancers
5.6 AI in Patient Monitoring and Wearable Health Devices
5.6.1 Monitoring Health Through Wearable’s and Personal Devices
5.6.2 Making Smartphone Selfies Into Powerful Diagnostic Tools
5.7 Revolutionizing of AI in Medicinal Decision-Making at the Bedside
5.8 Future of AI in Healthcare
5.9 Conclusion
References
6. Introduction of Artificial Intelligence
6.1 Introduction
6.1.1 Intelligence
6.1.2 Types of Intelligence
6.1.3 A Brief History of Artificial Intelligence From 1923 till 2000
6.2 Introduction to the Philosophy Behind Artificial Intelligence
6.2.1 Programming With and Without AI
6.3 Basic Functions of Artificial Intelligence
6.3.1 Categories of Artificial Intelligence
6.3.1.1 Reactive Machines
6.3.1.2 Limited Memory
6.3.1.3 Theory of Mind
6.3.1.4 Self-Awareness
6.4 Existing Technology and Its Review
6.4.1 Tesla’s Autopilot
6.4.2 Boxever
6.4.3 Fin Gesture
6.4.4 AI Robot
6.4.5 Vinci
6.4.6 AI Glasses
6.4.7 Affectiva
6.4.8 AlphaGo Beats
6.4.9 Cogito
6.4.10 Siri and Alexa
6.4.11 Pandora’s
6.5 Objectives
6.5.1 Major Goals
6.5.2 Need for Artificial Intelligence
6.5.3 Distinction Between Artificial Intelligence and Business Intelligence
6.6 Significance of the Study
6.6.1 Segments of Master Frameworks
6.6.1.1 User Interface
6.6.1.2 Expert Systems
6.6.1.3 Inference Engine
6.6.1.4 Voice Recognition
6.6.1.5 Robots
6.7 Discussion
6.7.1 Artificial Intelligence and Design Practice
6.8 Applications of AI
6.8.1 AI Has Been Developing a Huge Number of Tools Necessary to Find a Solution to the Most Challenging Problems in Computer Science
6.8.2 Future of AI
6.9 Conclusion
References
7. Artificial Intelligence in Healthcare: Algorithms and Decision Support Systems
7.1 Introduction
7.2 Machine Learning Work Flow and Applications in Healthcare
7.2.1 Formatting and Cleaning Data
7.2.2 Supervised and Unsupervised Learning
7.2.3 Linear Discriminant Analysis
7.2.4 K-Nearest Neighbor
7.2.5 K-Means Clustering
7.2.6 Random Forest
7.2.7 Decision Tree
7.2.8 Support Vector Machine
7.2.9 Artificial Neural Network
7.2.10 Natural Language Processing
7.2.11 Deep Learning
7.2.12 Ensembles
7.3 Commercial Decision Support Systems Based on AI IBM’s Watson
7.3.1 Personal Genome Diagnostics
7.3.2 Tempus
7.3.3 iCarbonX—Manage Your Digital Life
7.3.4 H2O.ai
7.3.5 Google DeepMind
7.3.6 Buoy Health
7.3.7 PathAI
7.3.8 Beth Israel Deaconess Medical Center
7.3.9 Bioxcel Therapeutics
7.3.10 BERG
7.3.11 Enlitic
7.3.12 Deep Genomics
7.3.13 Freenome
7.3.14 CloudMedX
7.3.15 Proscia
7.4 Conclusion
References
8. Smart Homes and Smart Cities
8.1 Smart Homes. 8.1.1 Introduction
8.1.2 Evolution of Smart Home
8.1.3 Smart Home Architecture
8.1.3.1 Smart Electrical Devices or Smart Plugs
8.1.3.2 Home Intelligent Terminals or Home Area Networks
8.1.3.3 Master Network
8.1.4 Smart Home Technologies
8.1.5 Smart Grid Technology
8.1.6 Smart Home Applications
8.1.6.1 Smart Home in the Healthcare of Elderly People
8.1.6.2 Smart Home in Education
8.1.6.3 Smart Lighting
8.1.6.4 Smart Surveillance
8.1.7 Advantages and Disadvantages of Smart Homes
8.2 Smart Cities. 8.2.1 Introduction
8.2.2 Smart City Framework
8.2.3 Architecture of Smart Cities
8.2.4 Components of Smart Cities
8.2.4.1 Smart Technology
8.2.4.2 Smart Infrastructure
8.2.4.3 Smart Mobility
8.2.4.4 Smart Buildings
8.2.4.5 Smart Energy
8.2.4.6 Smart Governance
8.2.4.7 Smart Healthcare
8.2.5 Characteristics of Smart Cities
8.2.6 Challenges in Smart Cities
8.2.7 Conclusion
References
9. Application of AI in Healthcare
9.1 Introduction
9.1.1 Supervised Learning Process
9.1.2 Unsupervised Learning Process
9.1.3 Semi-Supervised Learning Process
9.1.4 Reinforcement Learning Process
9.1.5 Healthcare System Using ML
9.1.6 Primary Examples of ML’s Implementation in the Healthcare. 9.1.6.1 AI-Assisted Radiology and Pathology
9.1.6.2 Physical Robots for Surgery Assistance
9.1.6.3 With the Assistance of AI/ML Techniques, Drug Discovery
9.1.6.4 Precision Medicine and Preventive Healthcare in the Future
9.2 Related Works. 9.2.1 In Healthcare, Data Driven AI Models
9.2.2 Support Vector Machine
9.2.3 Artificial Neural Networks
9.2.4 Logistic Regression
9.2.5 Random Forest
9.2.6 Discriminant Analysis
9.2.7 Naïve Bayes
9.2.8 Natural Language Processing
9.2.9 TF-IDF
9.2.10 Word Vectors
9.2.11 Deep Learning
9.2.12 Convolutional Neural Network
9.3 DL Frameworks for Identifying Disease. 9.3.1 TensorFlow
9.3.2 High Level APIs
9.3.3 Estimators
9.3.4 Accelerators
9.3.5 Low Level APIs
9.4 Proposed Work. 9.4.1 Application of AI in Finding Heart Disease
9.4.2 Data Pre-Processing and Classification of Heart Disease
9.5 Results and Discussions
9.6 Conclusion
References
10. Battery Life and Electric Vehicle Range Prediction
10.1 Introduction
10.2 Different Stages of Electrification of Electric Vehicles. 10.2.1 Starting and Stopping
10.2.2 Regenerative Braking
10.2.3 Motor Control
10.2.4 EV Drive
10.3 Estimating SoC
10.3.1 Cell Capacity
10.3.2 Calendar Life
10.3.3 Cycling Life
10.3.4 SoH Based on Capacity Fade
10.3.5 SoH Based on Power Fade
10.3.6 Open Circuit Voltage
10.3.7 Impedance Spectroscopy
10.3.8 Model-Based Approach
10.4 Kalman Filter
10.4.1 Sigma Point Kalman Filter
10.4.2 Six Step Process
10.5 Estimating SoH
10.6 Results and Discussion
10.7 Conclusion
References
11. AI-Driven Healthcare Analysis
11.1 Introduction
11.2 Literature Review
11.3 Feature Extraction
11.3.1 GLCM Feature Descriptors
11.4 Classifiers
11.4.1 Stochastic Gradient Descent Classifier
11.4.2 Naïve Bayes Classifier
11.4.3 K-Nearest Neighbor Classifier
11.4.4 Support Vector Machine Classifier
11.4.5 Random Forest Classifier
11.4.6 Working of Random Forest Algorithm
11.4.7 Convolutional Neural Network
11.4.7.1 Activation Function
11.4.7.2 Pooling Layer
11.4.7.3 Fully Connected Layer (FC)
11.5 Results and Conclusion
11.5.1 5,000 Images
11.5.2 10,000 Images
References
12. A Novel Technique for Continuous Monitoring of Fuel Adulteration
12.1 Introduction
12.1.1 Literature Review
12.1.2 Overview
12.1.3 Objective
12.2 Existing Method
12.2.1 Module-1 Water
12.2.2 Module-2 Petrol
12.2.3 Petrol Density Measurement
12.2.4 Block Diagram
12.2.5 Components of the System
12.2.5.1 Pressure Instrument
12.2.5.2 Sensor
12.2.6 Personal Computer
12.2.7 Petrol Density Measurement Instrument Setup
12.2.7.1 Setup 1
12.2.7.2 Setup 2
12.2.7.3 Setup 3
12.2.7.4 Setup 4
12.2.7.5 Final Setup
12.3 Interfacing MPX2010DP with INA114
12.3.1 I2C Bus Configuration for Honeywell Sensor
12.3.2 Pressure and Temperature Output Through I2C
12.4 Results and Discussion
12.5 Conclusion
References
13. Improved Merkle Hash and Trapdoor Function–Based Secure Mutual Authentication (IMH-TF-SMA) Mechanism for Securing Smart Home Environment
13.1 Introduction
13.2 Related Work
13.3 Proposed Improved Merkle Hash and Trapdoor Function–Based Secure Mutual Authentication (IMH-TF-SMA) Mechanism for Securing Smart Home Environment
13.3.1 Threat Model
13.3.2 IMH-TF-SMA Mechanism
13.3.2.1 Phase of Initialization
13.3.2.2 Phase of Addressing
13.3.2.3 Phase of Registration
13.3.2.4 Phase of Login Authentication
13.3.2.5 Phase of Session Agreement
13.4 Results and Discussion
13.5 Conclusion
References
14. Smart Sensing Technology
14.1 Introduction. 14.1.1 Sensor
14.1.1.1 Real-Time Example of Sensor
14.1.1.2 Definition of Sensors
14.1.1.3 Characteristics of Sensors
14.1.1.4 Classification of Sensors
14.1.1.5 Types of Sensors
14.1.1.5.1 Temperature Sensor
14.1.1.5.2 Proximity Sensor
14.1.1.5.3 Accelerometer
14.1.1.5.4 IR Sensor
14.1.1.5.5 Pressure Sensor
14.1.1.5.6 Water Quality Sensor
14.1.1.5.7 Chemical Sensor
14.1.1.5.8 Image Sensors
14.1.1.5.9 Motion Detectors
14.1.1.5.10 Smoke, Gas, and Alcohol Sensors
14.1.1.5.11 Humidity Sensor
14.1.1.5.12 Flow and Level Sensor
14.1.2 IoT (Internet of Things) 14.1.2.1 Trends and Characteristics
14.1.2.2 Definition
14.1.2.3 Flow Chart of IoT. 14.1.2.3.1 What is MQTT Broker?
14.1.2.4 IoT Phases
14.1.2.5 Phase Chart
14.1.2.6 IoT Protocol
14.1.2.6.1 1 IoT Needs Internet
14.1.3 WPAN
14.1.3.1 IEEE 802.15.1 Overview
14.1.3.2 Bluetooth
14.1.3.3 History of Bluetooth
14.1.3.4 How Bluetooth Works
14.1.3.5 Bluetooth Specifications
14.1.3.5.1 Core Specification
14.1.3.5.2 Profile Specification
14.1.3.6 Advantages of Bluetooth Technology
14.1.3.7 Applications
14.1.4 Zigbee (IEEE 802.15.4) 14.1.4.1 Introduction
14.1.4.2 Architecture of Zigbee
14.1.4.3 Zigbee Devices
14.1.4.4 Operating Modes of Zigbee
14.1.4.5 Zigbee Topologies
14.1.4.6 Applications of Zigbee Technology
14.1.5 WLAN. 14.1.5.1 Introduction
14.1.5.2 Advantages of WLANs
14.1.5.3 Drawbacks of WLAN
14.1.6 GSM. 14.1.6.1 Introduction
14.1.6.2 Composition of GSM Networks
14.1.6.3 Security
14.1.7 Smart Sensor
14.1.7.1 Development History of Smart Sensors
14.1.7.2 Internal Parts of Smart Transmitter
14.1.7.2.1 Hardware Components
14.1.7.2.2 Software Components
14.1.7.3 Applications
14.1.8 Conclusion
References
Index
Also of Interest
WILEY END USER LICENSE AGREEMENT
Отрывок из книги
Scrivener Publishing 100 Cummings Center, Suite 541J Beverly, MA 01915-6106
.....
In non-medical applications, WBAN is used in sports where devices can be wearable. It is effective to monitor the physiological activities of the wearer like heart rate, temperature, blood pressure, and posture of any attitude in sports. Navigation, timer, and distance can also be measured with the help of WBAN sensors.
Medical sensors are used to monitor a patient’s body continually and collect information so they should be active all the time; hence energy consumption is high. In body communication, sensors are implanted in vital areas of the body, so if the batteries are consumed fully, the patient has to undergo body surgery to replace a new one. Since the collection of data requires more energy than sending data through wireless time out Mac protocol, which is used in WBAN. Transmission of data is affected by jamming, bit error rate, and link quality. This can be minimized by using Cooperate Network Coding (CNC) since it does not require any retransmission when there is any failure in any of the nodes.
.....