Renewable Integrated Power System Stability and Control
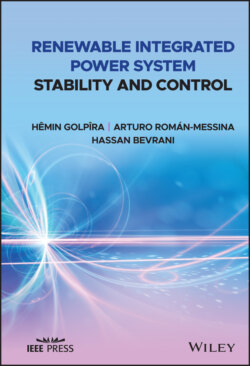
Реклама. ООО «ЛитРес», ИНН: 7719571260.
Оглавление
Hassan Bevrani. Renewable Integrated Power System Stability and Control
Table of Contents
List of Tables
List of Illustrations
Guide
Pages
Renewable Integrated Power System Stability and Control
Preface
Acknowledgments
Nomenclature
List of Abbreviations and Acronyms
1 Introduction
1.1 Power System Stability and Control
1.2 Current State of Power System Stability and Control
1.2.1 Frequency Control
1.2.2 Voltage Control
1.2.3 Oscillation Damping
1.3 Data‐Driven Wide‐Area Power System Monitoring and Control
1.4 Dynamics Modeling and Parameters Estimation
1.4.1 Modeling of Frequency, Voltage, and Angle Controls
1.4.2 Parameters Estimation
1.5 Summary
References
2 MG Penetrated Power Grid Modeling
2.1 Introduction
2.2 Basic Concepts. 2.2.1 Dynamic Equivalencing
2.2.2 Background on Study Zone and External System
2.3 Power Grid Modeling
2.3.1 The Notion of Center‐of Gravity (COG)
2.3.1.1 Key Concept
2.3.1.2 Basic Assumptions
2.3.1.3 Modeling Formulation
2.3.1.4 Local Frequency Estimation
2.3.1.5 Simulation Results
Two‐Area System
NYNE Test System
IEEE 50‐Machine Test System
2.3.2 An Enhanced COG‐Based Model. 2.3.2.1 Key Concept
2.3.2.2 Simulation Results
2.3.3 Generalized Equivalent Model. 2.3.3.1 Basic Logic
2.3.3.2 Simulation and Results
2.4 MG Equivalent Model
2.4.1 Islanded Mode. 2.4.1.1 Synchronous‐Based DG
2.4.1.2 Genset Model Validation
2.4.1.3 Inverter‐Based DG
2.4.1.4 Inverter‐Based DG Model Validation
2.4.2 Grid‐Connected Mode. 2.4.2.1 Basic Logic
2.4.2.2 Model Validation
2.5 Summary
References
3 Stability Assessment of Power Grids with High Microgrid Penetration
3.1 Introduction. 3.1.1 Motivation
3.1.2 Relations with Previous Literature
3.2 Frequency Stability Assessment
3.2.1 Background on Frequency Indices
3.2.1.1 Rate of Change of Frequency
3.2.1.2 Frequency Nadir
3.2.1.3 Delta Frequency Detection
3.2.2 Frequency Stability Assessment Under High MG Penetration Levels
3.2.3 Sensitivity Factors. 3.2.3.1 Frequency Response
3.2.3.2 Delta Frequency Detection
3.2.4 Simulation and Results
3.3 Maximum Penetration Level: Frequency Stability. 3.3.1 Basic Principle
3.3.2 Background on MG Modeling
3.3.3 Minimum Inertia Related to Frequency Nadir
3.3.4 Minimum Inertia Related to Delta Frequency Detection
3.3.5 Minimum Inertia Related to RoCoF
3.3.6 Maximum Penetration Level
3.3.7 Simulation and Results
3.3.7.1 Analysis Tools
3.3.7.2 Dynamical Simulation Results
3.4 Small‐Signal Stability Assessment. 3.4.1 Basic Definition
3.4.2 Key Concept
3.4.3 Simulation and Results
3.5 Maximum Penetration Level: Small‐Signal Stability. 3.5.1 Basic Idea
3.5.2 Simulation and Results
3.6 Voltage‐Based Realization of the MG‐Integrated Power Grid
3.6.1 Key Concepts
3.6.2 Jacobian Sensitivities
3.6.2.1 V‐P Sensitivity
3.6.2.2 V‐Q Sensitivity
3.6.3 Simulation and Results
3.7 Summary
References
4 Advanced Virtual Inertia Control and Optimal Placement
4.1 Introduction
4.2 Virtual Synchronous Generator
4.2.1 Concept and Structure
4.2.2 Basic Control Scheme and Applications
4.2.3 Application in Power System Dynamic Enhancement
4.2.3.1 Scenario 1: 10‐MW Load Increase at Bus 9
4.2.3.2 Scenario 2: 20‐MW Power Command Decrease of G3
4.2.4 Application to Power Grids with HVDC Systems
4.3 Dispatchable Inertia Placement
4.3.1 Frequency Dynamics Enhancement
4.3.1.1 Background: Literature Review
4.3.1.2 Virtual Inertia Modeling. Main Idea
MUSIC Analysis: Methodology and Application
4.3.1.3 Experimental Verification
4.3.1.4 Economic Modeling
Costs of Energy Storages and Technologies
Formulation of the Objective Function and Constraints
Determining the Bounds of Constraints
Rate of Change of Frequency
Frequency Nadir
4.3.1.5 Simulation and Results. Linear System
Two‐Area Power System
New York New England System
4.3.1.6 Sensitivity Analysis
4.3.2 Small‐Signal Stability. 4.3.2.1 Objective Function
4.3.2.2 Simulation Results
4.4 Summary
References
5 Wide‐Area Voltage Monitoring in High‐Renewable Integrated Power Systems
5.1 Introduction
5.2 Voltage Control Areas: A Background
5.2.1 Voltage Sensitivities
5.2.2 Electrical Distances
5.2.3 Reactive Control Zones and Pilot Nodes
5.2.3.1 Selection of Optimal Pilot Buses
5.2.3.2 Selection of Control Plants
5.2.4 Other Approaches
5.3 Data‐driven Approaches
5.3.1 Wide‐Area Voltage and Reactive Power Regulation
5.3.2 PMU‐Based Voltage Monitoring
5.4 Theoretical Framework
5.4.1 Dynamic Trajectories
5.4.2 Spectral Graph Theory
5.4.3 Kernel Methods
5.4.3.1 Markov Matrices
5.4.3.2 The Markov Clustering Algorithm
5.4.4 Spatiotemporal Clustering
5.5 Case Study
5.5.1 Sensitivity Studies
5.5.2 Data‐Driven Analysis
5.5.3 Measurement‐Based Reactive Control Areas. 5.5.3.1 Diffusion Maps
5.5.4 Direct Clustering
5.5.5 Correlation Analysis
5.5.5.1 Direct Analysis of Concatenated Data
5.5.5.2 Two‐Way Correlation Analysis
5.5.5.3 Partial Least Squares Correlation
5.6 Summary
References
6 Advanced Control Synthesis
6.1 Introduction
6.2 Frequency Dynamics Enhancement. 6.2.1 Background: The Concept of Flexible Inertia
6.2.2 Frequency Dynamics Propagation
6.2.3 Inertia‐Based Control Scheme
6.2.4 Flexible Inertia: Practical Considerations
6.2.5 Results and Discussions
6.3 Small Signal Stability Enhancement. 6.3.1 Key Concept
6.3.2 Control Scheme Design
6.3.3 Simulation and Results
6.4 Summary
References
7 Small‐Signal and Transient Stability Assessment Using Data‐Driven Approaches
7.1 Background and Motivation
7.2 Modal Characterization Using Data‐Driven Approaches
7.2.1 Modal Decomposition
7.2.2 Multisignal Prony Analysis
7.2.2.1 Standard Prony Analysis
7.2.2.2 Modified Least‐Squares Algorithm
7.2.2.3 Multichannel Prony Analysis
7.2.2.4 Hankel‐SVD Methods
7.2.3 Koopman and Dynamic Mode Decomposition Representations
7.2.3.1 The Koopman Operator
7.2.4 Dynamic Mode Decomposition
7.2.4.1 SVD‐Based Methods
7.2.4.2 The Companion Matrix Approach
7.2.4.3 Energy Criteria
7.3 Studies of a Small‐Scale Power System Model
7.3.1 System Data and Operating Scenarios
7.3.2 Exploratory Small‐Signal Analysis
7.3.3 Large System Performance
7.3.3.1 Cases B–C
7.3.3.2 Case D
7.3.4 Mode Shape Identification
7.3.5 Temporal Clustering
7.4 Large‐Scale System Study
7.4.1 Case Study Description
7.4.2 Renewable Generator Modeling
7.4.3 Effect of Inverter‐Based DGs on Oscillatory Stability
7.4.4 Large System Performance
7.4.5 Model Validation
7.4.5.1 Reconstructed Flow Fields
7.4.6 Identification of Mode Shapes Using DMD
7.5 Analysis Results and Discussion
References
8 Solar and Wind Integration Case Studies
8.1 General Context and Motivation
8.2 Study System
8.3 Wind Power Integration in the South Systems
8.3.1 Study Region
8.3.2 Existing System Limitations
8.4 Impact of Increased Wind Penetration on the System Performance
8.4.1 Study Considerations and Scenario Development
8.4.2 Base Case Assessment
8.4.2.1 System Oscillatory Response
8.4.3 High Wind Penetration Case
8.5 Frequency Response
8.5.1 Frequency Variations
8.5.2 Wind and Hydropower Coordination
8.5.3 Response to Loss‐of‐Generation Events
8.6 Effect of Voltage Control on System Dynamic Performance
8.6.1 Voltage Support and Reactive Power Dispatch
8.6.2 Effect of Voltage Control Characteristics
8.7 Summary
References
Index. a
b
d
e
f
g
h
i
j
k
l
m
n
o
p
q
r
s
t
u
v
w
z
WILEY END USER LICENSE AGREEMENT
Отрывок из книги
IEEE Press 445 Hoes Lane Piscataway, NJ 08854
.....
In comparison of frequency and voltage control, a higher number of reports have been published in PMU‐based oscillation damping (rotor angle control) field. However, most of the reported approaches require the detailed and accurate knowledge of the complete network model (both topology and parameter values), that is unavailable or corrupted in practice as a result of communication failures, bad data in state estimation etc. In addition, the impact of disturbances on the inter‐area oscillations cannot be well captured by these methods.
Power grids modeling and control has become a more challenging issue due to the increasing penetration of RESs, changing system structure and the integration of new storage systems, controllable loads and power electronics technologies, and reduction of system inertia. Conventional modeling and control designs may not be any more effective to satisfy all specified objectives in various operation modes of modern power grids. These challenging issues set new demand for the development of more flexible, rapid, effective, precise, and adaptive approaches for power system dynamic monitoring, stability/security analysis, and control problems. Thanks to recent advances in control, communication, and computing technologies, it is possible to tackle mentioned challenges by implementing a data‐driven‐based modeling and control framework as shown in Figure 1.2.
.....