Data Control
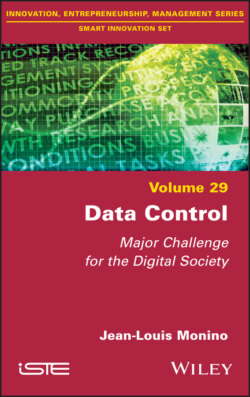
Реклама. ООО «ЛитРес», ИНН: 7719571260.
Оглавление
Jean-Louis Monino. Data Control
Table of Contents
List of Illustrations
Guide
Pages
Data Control. Major Challenge for the Digital Society
Foreword
Acknowledgements
Introduction
Notes
1 From Data to Decision-Making: A Major Pathway
1.1. Background on economic intelligence
1.2. Strategic economic intelligence revisited
1.2.1. The three major steps for decision support
1.2.2. Modeling the concept of strategic business intelligence
1.2.2.1. Data
1.2.2.2. From data to information: monitoring
1.2.2.3. From intelligence to economic intelligence
1.2.2.4. Information
1.2.2.5. From information to knowledge: economic intelligence
1.2.2.6. Knowledge
1.2.2.7. From knowledge to decision-making: strategic business intelligence
1.3. Conclusion
Notes
2 Data: An Indispensable Platform for Companies
2.1. The key figures of digital technology
Box 2.1.The platform of the international agency We Are Social
2.1.1. Figures on social networks
2.1.2. Numbers: Big Data
Box 2.2.“Zero Marginal Cost Economy”
2.1.3. Key figures: the Internet of Things
Box 2.3.An example of data access
2.2. The power of data: a major challenge
Box 2.4.How to organize and use data
2.3. The Big Data revolution, “Mega Data”
2.3.1. Understanding the world of Big Data
2.3.1.1. What changes in data analysis?
2.3.1.2. Challenges around Big Data
Box 2.5.Study on mastery of data processing techniques
2.3.1.3. Making data warehouses intelligent
Box 2.6.The McKinsey Global Institute Report on Big Data and Smart Data
Box 2.7.The data scientist
2.3.2. Open data: a new challenge
2.3.2.1. Why open data?
Box 2.8.Definition of open data
Box 2.9.Three criteria for open data
2.3.2.2. Making the data reusable
Box 2.10.Producers and re-users, types of actors
Box 2.11. The benefits of opening up data
2.3.2.3. Open data and Big Data
Box 2.12.Proposed approach
2.3.2.4. Valuation and reuse of data
2.4. Developing the culture of data sharing
Box 2.13.Open data and job creation
2.5. Storage of data in databases
Box 2.14.Different types of databases. From an article on the LeBigData platform (Bastien 2019)
2.6. The appearance of buzzwords: Big, Open, Viz, etc
2.7. Conclusion
Box 2.15.Economic domain in three categories
Notes
3 From Data to Information: Essential Transformations. 3.1. Value creation from data processing1
Box 3.1.INSEE and the grid pattern
Box 3.2.A start-up population tracking application AUTOUR.CIM
Box 3.3.The coloring model proposed by Monino-Boya
3.2. Value creation and analysis of open databases
Box 3.4.The OECD identifies five areas of potential value creation
Box 3.5.The eight principles of data accessibility
3.3. From data to information: the “DataViz” or data visualization
3.4. From data to information: statistical processing
3.4.1. Phases of data processing
3.4.2. Processing the data
3.4.2.1. Processing of non-digitized data
3.4.2.2. Processing of digitized data
3.4.2.3. Statistical processing of data
Box 3.6.Data visualization
3.5. Turning mass data into an opportunity for innovation
Box 3.7.The 10 most innovative firms
Box 3.8.Ranking of the world's most attractive cities
3.6. Development of company assets in the web of data
3.7. Conclusion
Notes
4 Information: Contextualized and Materialized Data
4.1. What is information?
4.1.1. How can we define information?3
Box 4.1.Different meanings of information
Box 4.2.Overall approach to information governance
4.2. Internal and external information
4.2.1. Internal information
4.2.2. External information
4.3. Formal and informal information
4.3.1. Formal information
4.3.2. Informal information
4.4. Importance of information
4.4.1. White information
4.4.2. Gray information
4.4.3. Black information
4.5. Décodex5 set up by Le Monde
Box 4.3.The DÉCODEX set up by Le Monde
4.6. Conclusion
Notes
5 From Information to Knowledge: Valuing and Innovating
5.1. Innovation as a driving force of growth
5.1.1. Innovation and the intangible economy
5.2. Knowledge: the key to innovation
Box 5.1.Knowledge and new knowledge
5.3. Building knowledge: economic intelligence
5.3.1. The EI process and the transition from information to knowledge
5.3.2. Managing the data warehouse to extract knowledge and insight
5.4. Data mining, Statistica and Tibco1
5.5. Information an economic good?
5.5.1. Innovation as a driving force of growth
5.5.2. Strategic business intelligence
Box 5.2.Business intelligence and new risks for the 21st Century4
Box 5.3.McKinsey and Company
5.6. What is data science?
5.7. Conclusion
Note
6. From Knowledge to Strategic Business Intelligence: Decision-Making
6.1. Data valuation mechanisms
6.2. How do you value data
Box 6.1.Digital data centers or factories and number of data centers worldwide by country in 2018 (source: Statista)
Box 6.2.An example of data processing for the start-up 123PRESTA in 2010
Box 6.3.Time series forecasting with neural networks. Turnover of mass retail stores
Box 6.4.Chaos, Hurst and bootstrap exhibitors. An example applied to the Paris Stock Exchange (Matouk and Monino 2005)
6.3. Data governance: a key factor in valuation
Box 6.5.The challenges of multiple sources
Box 6.6.El short presentation videos
6.4. EI: protection and enhancement of digital heritage
Box 6.7.The place of geolocation data
6.5. Data analysis techniques: data mining/text mining
Box 6.8.An example of data processing a database for a start-up with TIBCO's Statistica
6.6. Conclusion
Notes
Conclusion
Box C.1.Creating value from data - suggestions
Note
Glossary
References
Webography
Index. A, B, C
D, E, F
G, H, I
K, M, N, P
Q, S, T,V
Other titles from. in Innovation, Entrepreneurship and Management. 2020
2019
2018
2017
2016
2015
2014
2013
2012
2011
2010
2009
2008
2007
2006
WILEY END USER LICENSE AGREEMENT
Отрывок из книги
Smart Innovation Set coordinated by Dimitri Uzunidis
Volume 29
.....
In this context, companies must have the capacity to absorb all available data, enabling them to assimilate and reproduce knowledge. This capacity presupposes the existence of specific skills that enable the use of this knowledge. The training of “data scientists” is therefore essential in order to be able to identify useful approaches to opening up or internal exploitation of data and to quantify the benefits in terms of innovation and competitiveness, since Big Data is only one element of a new set of tools and techniques called “data science”.
The Data Scientist's mission is to extract knowledge from company data. They will be called upon to perform strategic functions within the Commission. To do so, they must master the necessary tools. They must also be more pedagogical and increase their command of data mining, because the volume of data requires an increase in the range of techniques to be mastered.
.....