Handbook of Web Surveys
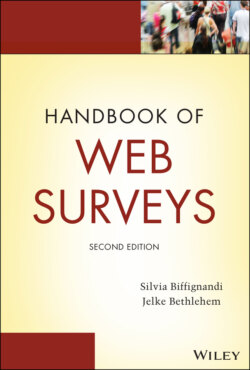
Реклама. ООО «ЛитРес», ИНН: 7719571260.
Оглавление
Jelke Bethlehem. Handbook of Web Surveys
Table of Contents
List of Tables
List of Illustrations
Guide
Pages
Handbook of Web Surveys
Preface
Chapter One The Road to Web Surveys. 1.1 Introduction
1.2 Theory. 1.2.1 THE EVERLASTING DEMAND FOR STATISTICAL INFORMATION
EXAMPLE 1.1 The representative method of Anders Kiaer
1.2.2 TRADITIONAL DATA COLLECTION
EXAMPLE 1.2 The first telephone survey in the Netherlands
1.2.3 THE ERA OF COMPUTER‐ASSISTED INTERVIEWING
1.2.4 THE CONQUEST OF THE WEB
EXAMPLE 1.3 The first e‐mail survey at Statistics Netherlands
EXAMPLE 1.4 The production statistics pilot at Statistics Netherlands
EXAMPLE 1.5 Designing questions in HTML 2.0
EXAMPLE 1.6 Experiment with a mixed‐mode surveys
1.2.5 WEB SURVEYS AND OTHER SOURCES
EXAMPLE 1.7 Web scraping, administrative data, and surveys
EXAMPLE 1.8 Social media and surveys
1.2.6 HISTORIC SUMMARY
1.2.7 PRESENT‐DAY CHALLENGES AND OPPORTUNITIES
1.2.8 CONCLUSIONS FROM MODERN‐DAY CHALLENGES
1.2.9 THRIVING IN THE MODERN‐DAY SURVEY WORLD
1.3 Application
1.3.1 BLAISE
1.4 Summary
KEY TERMS
EXERCISES
REFERENCES
Chapter Two About Web Surveys. 2.1 Introduction
EXAMPLE 2.1 A web survey on technological communication and links between enterprises
2.2 Theory
2.2.1 TYPICAL SURVEY SITUATIONS
EXAMPLE 2.2 The ICT survey pilot
2.2.2 WHY ONLINE DATA COLLECTION?
2.2.2.1 Advantages
2.2.2.2 Disadvantages and Problems
2.2.3 AREAS OF APPLICATION
EXAMPLE 2.3 Reliable web surveys
EXAMPLE 2.4 The Kauffman Firm Survey (KFS)
2.2.4 TRENDS IN WEB SURVEYS
2.3 Application
2.4 Summary
KEY TERMS
EXERCISES
REFERENCES
Chapter Three A Framework for Steps and Errors in Web Surveys. 3.1 Introduction
3.2 Theory
EXAMPLE 3.1 A metadata database: variables definitions
EXAMPLE 3.2 Compulsory question
EXAMPLE 3.3 Automatic control in web questionnaire
EXAMPLE 3.4 Paper versus web: errors comparison
3.3 Application
3.4 Summary
KEY TERMS
EXERCISES
REFERENCES
Notes
Chapter Four Sampling for Web Surveys. 4.1 Introduction
4.2 Theory. 4.2.1 TARGET POPULATION
EXAMPLE 4.1 A survey about road pricing
EXAMPLE 4.2 Target variables
4.2.2 SAMPLING FRAMES
EXAMPLE 4.3 Postal Address Files
EXAMPLE 4.4 Sample selection for the LISS panel
EXAMPLE 4.5 Over‐coverage or nonresponse?
4.2.3 BASIC CONCEPTS OF SAMPLING
4.2.4 SIMPLE RANDOM SAMPLING
EXAMPLE 4.6 Effect of sample size on the precision of an estimator
EXAMPLE 4.7 Estimating a percentage
4.2.5 DETERMINING THE SAMPLE SIZE
4.2.5.1 The Sample Size for Estimating a Percentage
EXAMPLE 4.8 The sample size for an opinion poll
4.2.5.2 The Sample Size for Estimating a Mean
4.2.6 SOME OTHER SAMPLING DESIGNS
4.2.6.1 Stratified Sampling
EXAMPLE 4.9 Business surveys in the Netherlands
4.2.6.2 Unequal Probability Sampling
EXAMPLE 4.10 Sampling addresses for a web survey: a case of unequal probabilities
4.2.6.3 Cluster Sampling
4.2.6.4 Two‐Stage Sampling
EXAMPLE 4.11 The Safety Monitor of Statistics Netherlands
4.2.7 ESTIMATION PROCEDURES
4.2.7.1 The Ratio Estimator
4.2.7.2 The Regression Estimator
EXAMPLE 4.12 A dairy farm survey
4.2.7.3 The Post‐stratification Estimator
4.2.7.4 The Generalized Regression Estimator
4.3 Application
4.4 Summary
KEY TERMS
EXERCISES
REFERENCES
Chapter Five. Errors in Web Surveys. 5.1 Introduction
EXAMPLE 5.1 Selection probabilities in an address sample
EXAMPLE 5.2 A survey about road pricing
5.2 Theory
5.2.1 MEASUREMENT ERRORS
5.2.1.1 Satisficing
5.2.1.2 Response Order Effects
5.2.1.3 Acquiescence
EXAMPLE 5.3 Bias caused by acquiescence
5.2.1.4 Endorsing the Status Quo
EXAMPLE 5.4 Including or excluding a middle option
5.2.1.5 Non‐differentiation
5.2.1.6 Don't Know
5.2.1.6.1 Offer “don't know” explicitly
5.2.1.6.2 Offer “don't know” explicitly, but less obvious
5.2.1.6.3 Offer “don't know” implicitly
5.2.1.6.4 Do not offer “don't know”
EXAMPLE 5.5 Using a filter question for “don't know”
5.2.1.7 Arbitrary Answer
5.2.1.8 Socially Desirable Answers
5.2.1.9 Some Web Survey Design Issues
5.2.1.10 Other Measurement Errors
5.2.2 NONRESPONSE
5.2.2.1 The Nonresponse Problem
EXAMPLE 5.6 The Dutch Housing Demand Survey nonresponse
5.2.2.2 Causes of Nonresponse
5.2.2.3 Response Rate
EXAMPLE 5.7 Response rates in the LISS panel
5.2.2.4 The Effect of Nonresponse
5.2.2.5 Analysis and Correction of Nonresponse
EXAMPLE 5.8 Nonresponse in the LISS panel
5.3 Application. 5.3.1 THE SAFETY MONITOR
5.3.2 MEASUREMENT ERRORS
5.3.3 NONRESPONSE
5.4 Summary
KEY TERMS
EXERCISES
REFERENCES
Chapter Six Web Surveys and Other Modes of Data Collection. 6.1 Introduction. 6.1.1 MODES OF DATA COLLECTION
6.1.2 THE CHOICE OF THE MODES OF DATA COLLECTION
EXAMPLE 6.1 Accuracy and reliability of a bathroom scale
6.2 Theory. 6.2.1 FACE‐TO‐FACE SURVEYS
6.2.2 TELEPHONE SURVEYS
6.2.3 MAIL SURVEYS
6.2.4 WEB SURVEYS
6.2.5 MOBILE WEB SURVEYS
6.3 Application
6.4 Summary
KEY TERMS
EXERCISES
REFERENCES
Chapter Seven. Designing a Web Survey Questionnaire. 7.1 Introduction
7.2 Theory
7.2.1 THE ROAD MAP TOWARD A WEB QUESTIONNAIRE
EXAMPLE 7.1 The library study
EXAMPLE 7.2 A progress indicator
7.2.2 THE LANGUAGE OF QUESTIONS
EXAMPLE 7.3 Use of pictures in questions
7.2.3 BASIC CONCEPTS OF VISUALIZATION. 7.2.3.1 Answer Spaces
EXAMPLE 7.4 Adding a frame to an answer space
EXAMPLE 7.5 Asking values in R&D survey
7.2.3.2 Use of Color
7.2.3.3 Use of Images
EXAMPLE 7.6 Survey about mobile phones
7.2.4 ANSWERS TYPES (RESPONSE FORMAT)
7.2.4.1 Radio Buttons
EXAMPLE 7.7 Answering a closed question
EXAMPLE 7.8 Asking about the use of mobile phones
EXAMPLE 7.9 Question structure
7.2.4.2 Drop‐Down Boxes
7.2.4.3 Checkboxes
7.2.4.4 Text Boxes and Text Areas
7.2.5 WEB QUESTIONNAIRES AND PARADATA. 7.2.5.1 Definition of Paradata
EXAMPLE 7.10 Audit trials
7.2.5.2 Use of Paradata
7.2.6 TRENDS IN WEB QUESTIONNAIRE DESIGN AND VISUALIZATION. 7.2.6.1 The Cognitive Approach to Web Questionnaire Design
EXAMPLE 7.11 Cognitive interviewing
7.3 Application
7.4 Summary
KEY TERMS
EXERCISES
REFERENCES
Chapter Eight Adaptive and Responsive Design. 8.1 Introduction
8.2 Theory
8.2.1 TERMINOLOGY
EXAMPLE 8.1 Adaptive survey design
EXAMPLE 8.2 Adaptive survey design
8.2.2 QUALITY AND COST FUNCTIONS
8.2.3 STRATEGY ALLOCATION AND OPTIMIZATION
EXAMPLE 8.3 Estimating response propensities
EXAMPLE 8.4 Quality functions
EXAMPLE 8.5 Estimating costs
EXAMPLE 8.6 Experiment in the Dutch Survey of Consumer Sentiments
EXAMPLE 8.7 Experiment in the High School Longitudinal Study
EXAMPLE 8.8 Optimization problem
8.3 Application
8.4 Summary
KEY TERMS
EXERCISES
REFERENCES
Chapter Nine. Mixed‐mode Surveys. 9.1 Introduction
EXAMPLE 9.1 A mixed‐mode survey on customer satisfaction
9.2 The Theory. 9.2.1 WHAT IS MIXED‐MODE?
EXAMPLE 9.2 Understanding Society longitudinal panel
9.2.2 WHY MIXED‐MODE?
9.2.2.1 Response Rates
EXAMPLE 9.3 Mixed‐mode and incentives
EXAMPLE 9.4 European Health Interview Survey (EHIS), in Germany
9.2.2.2 Costs
9.2.2.3 Data Quality
9.2.2.4 Coverage Problems
9.2.2.5 Selection Errors
9.2.2.6 Cognitive Efforts
9.3 Methodological Issues
9.3.1 PREVENTING MODE EFFECTS THROUGH QUESTIONNAIRE DESIGN
9.3.2 HOW TO MIX MODES?
EXAMPLE 9.5 The Safety Monitor Pilot
9.3.3 HOW TO COMPUTE RESPONSE RATES?
EXAMPLE 9.6 Computing response rates
9.3.4 AVOIDING AND ADJUSTING MODE EFFECTS FOR INFERENCE
EXAMPLE 9.7 Estimation effects in mixed‐mode surveys
EXAMPLE 9.8 A longitudinal study
9.3.5 MIXED‐MODE BY BUSINESSES AND HOUSEHOLDS. 9.3.5.1 Mixed‐mode for Business Surveys
EXAMPLE 9.9 Some experiences in the United States and in Europe
EXAMPLE 9.10 A mixed‐mode survey of manufacturing firms
EXAMPLE 9.11 Mixed‐mode in the Italian SCI survey
9.3.5.2 Mixed‐mode for Surveys Among Households and Individuals
EXAMPLE 9.12 Response rates in mixed‐mode surveys
EXAMPLE 9.13 Surveys in the Netherlands
EXAMPLE 9.14 Measurement errors in the ESS (European Social Survey) mixed‐mode experiment
9.4 Application
9.5 Summary
KEY TERMS
EXERCISES
REFERENCES
Chapter Ten The Problem of Under‐coverage. 10.1 Introduction
EXAMPLE 10.1 A web survey with telephone recruitment
10.2 Theory. 10.2.1 THE INTERNET POPULATION
10.2.2 A RANDOM SAMPLE FROM THE INTERNET POPULATION
10.2.3 REDUCING THE NON‐COVERAGE BIAS
10.2.4 MIXED‐MODE DATA COLLECTION
10.3 Application
10.4 Summary
KEY TERMS
EXERCISES
REFERENCES
Chapter Eleven. The Problem of Self‐Selection. 11.1 Introduction
EXAMPLE 11.1 Opinion polls in the Netherlands
EXAMPLE 11.2 The presidential elections in the United States in 2016
11.2 Theory. 11.2.1 BASIC SAMPLING THEORY
11.2.2 A SELF‐SELECTION SAMPLE FROM THE INTERNET POPULATION
EXAMPLE 11.3 The bias worst case in Dutch surveys
11.2.3 REDUCING THE SELF‐SELECTION BIAS
EXAMPLE 11.4 The LISS panel
11.3 Applications
11.3.1 APPLICATION 1: SIMULATING SELF‐SELECTION POLLS
11.3.2 APPLICATION 2: SUNDAY SHOPPING IN ALPHEN A/D RIJN
11.4 Summary
KEY TERMS
EXERCISES
REFERENCES
Chapter Twelve. Weighting Adjustment Techniques
12.1 Introduction
EXAMPLE 12.1 A web survey based on an address sample
12.2 Theory. 12.2.1 THE CONCEPT OF REPRESENTATIVITY
12.2.2 POST‐STRATIFICATION
EXAMPLE 12.2 Computing weights by means of post‐stratification
EXAMPLE 12.3 The variance of post‐stratification estimator
EXAMPLE 12.4 Using post‐stratification for reducing nonresponse bias
EXAMPLE 12.5 Using post‐stratification for reducing under‐coverage bias
EXAMPLE 12.6 Using post‐stratification for reducing self‐selection bias
EXAMPLE 12.7 Incomplete population information
12.2.3 GENERALIZED REGRESSION ESTIMATION
EXAMPLE 12.8 Post‐stratification as a special case of generalized regression estimation
EXAMPLE 12.9 Generalized regression estimation using only marginal distributions
EXAMPLE 12.10 Using generalized regression estimation for reducing self‐selection bias
12.2.4 RAKING RATIO ESTIMATION
EXAMPLE 12.11 Raking ratio estimation
12.2.5 CALIBRATION ESTIMATION
12.2.6 CONSTRAINING THE VALUES OF WEIGHTS
12.2.7 CORRECTION USING A REFERENCE SURVEY
EXAMPLE 12.12 Using a reference survey for reducing under‐coverage bias
EXAMPLE 12.13 Using a reference survey for reducing self‐selection bias
12.3 Application
12.4 Summary
KEY TERMS
EXERCISES
REFERENCES
Chapter Thirteen Use of Response Propensities. 13.1 Introduction
EXAMPLE 13.1 Response propensities using a reference survey
13.2 Theory
13.2.1 A SIMPLE RANDOM SAMPLE WITH NONRESPONSE
13.2.2 A SELF‐SELECTION SAMPLE
13.2.3 THE RESPONSE PROPENSITY DEFINITION
13.2.4 MODELS FOR RESPONSE PROPENSITIES
EXAMPLE 13.2 Estimating response propensities
EXAMPLE 13.3 Constructing a model for response propensities
13.2.5 CORRECTION METHODS BASED ON RESPONSE PROPENSITIES
13.2.5.1 Response Propensity Weighting
13.2.5.2 Response Propensity Stratification
EXAMPLE 13.4 Constructing response propensity strata
13.3 Application
13.3.1 GENERATION OF THE POPULATION
13.3.2 GENERATION OF RESPONSE PROBABILITIES
13.3.3 GENERATION OF THE SAMPLE
13.3.4 COMPUTATION OF RESPONSE PROPENSITIES
13.3.5 MATCHING RESPONSE PROPENSITIES
13.3.6 ESTIMATION OF POPULATION CHARACTERISTICS
13.3.7 EVALUATING THE RESULTS
13.3.8 MODEL SENSITIVITY
13.4 Summary
KEY TERMS
EXERCISES
REFERENCES
Chapter Fourteen. Web Panels
14.1 Introduction
EXAMPLE 14.1 Examples of web panels
EXAMPLE 14.2 The Dutch online panel study
EXAMPLE 14.3 Sources of quality guidelines
14.2 Theory. 14.2.1 UNDER‐COVERAGE
14.2.2 RECRUITMENT
EXAMPLE 14.4 Panel recruitment
Example 14.5 Panel manipulation
14.2.3 NONRESPONSE
EXAMPLE 14.6 Nonresponse in the LISS Panel
14.2.3.1 The Recruitment Rate
EXAMPLE 14.7 Computing recruitment rate
14.2.3.2 The Profile Rate
EXAMPLE 14.8 Computing the profile rate
14.2.3.3 The Absorption Rate
14.2.3.4 The Completion Rate
14.2.3.5 The Break‐Off Rate
14.2.3.6 The Screening Completion Rate and the Study‐Specific Rate
14.2.3.7 Cumulative Response Rates
14.2.3.8 The Attrition Rate
14.2.4 REPRESENTATIVITY
14.2.5 WEIGHTING ADJUSTMENT FOR PANELS
EXAMPLE 14.9 Comparing web panels
14.2.6 PANEL MAINTENANCE
14.2.6.1 Frequency of Surveys
14.2.6.2 Panel Refreshment
14.2.6.3 Maximum Stay
14.3 Applications
14.3.1 APPLICATION 1: THE WEB PANEL PILOT OF STATISTICS NETHERLANDS
14.3.2 APPLICATION 2: THE U.K. POLLING DISASTER
14.4 Summary
KEY TERMS
EXERCISES
REFERENCES
Index
WILEY END USER LICENSE AGREEMENT
Отрывок из книги
Second Edition
Silvia Biffignandi
.....
General population web surveys were rare in the first period of existence of the Internet. This was due to the low Internet penetration among households. This prevented conducting representative surveys. However, there were polls on the Internet. Recruitment of respondents was based on self‐selection and not on probability sampling. Users could even create their own polls on websites like Survey Central, Open Debate, and Internet Voice (see O'Connell, 1998).
Also in 1998, the Survey2000 project was carried out. This was a large self‐selection web survey on the website of the National Geographic Society. This was a survey on mobility, community, and cultural identity. In a period of two months, over 80,000 respondents completed the questionnaire. See Witte, Amoroso, and Howard (2000) for more details about this project.
.....