Spatial Regression Models for the Social Sciences
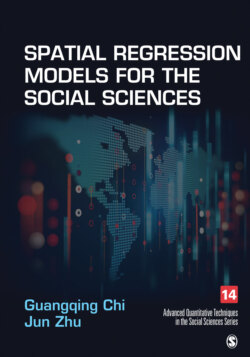
Реклама. ООО «ЛитРес», ИНН: 7719571260.
Оглавление
Jun Zhu. Spatial Regression Models for the Social Sciences
Spatial Regression Models for the Social Sciences
Brief Contents
Detailed Contents
List of Figures
Series Editor’s Introduction
Preface
Why This Book?
Who Is This Book for?
Acknowledgments
About the Authors
1 Introduction. Learning Objectives
1.1 Spatial Thinking in the Social Sciences
1.2 Introduction to Spatial Effects
1.2.1 Spatial Analysis, Spatial Data Analysis, and Geographic Analysis
1.2.2 Four Types of Spatial Data Analysis
1.3 Introduction to the Data Example
1.3.1 Population Change as a Spatial Process
1.3.2 The State of Wisconsin in the United States: The Study Area
1.3.3 Minor Civil Division (MCD): The Spatial Unit of Analysis
1.3.4 Population Change in Wisconsin
1.4 Structure of the Book
Descriptions of Images and Figures
2 Exploratory Spatial Data Analysis. Learning Objectives
2.1 Exploratory Data Analysis. 2.1.1 The Methods
2.1.2 Data Example
2.1.2.1 Explanatory Variable 1: Previous Growth (Population Growth Rate From 1990 to 2000)
2.1.2.2 Explanatory Variable 2: Old (the Percentage of the Old Population [Age Sixty-Five and Older] in 2000)
2.1.2.3 Explanatory Variable 3: Unemployment (Unemployment Rate in 2000)
2.1.2.4 Explanatory Variable 4: Airport (Proximity to the Nearest Major Commercial Airport)
2.1.2.5 Explanatory Variable 5: Forest (the Percentage of Forest Coverage)
2.1.2.6 Explanatory Variable 6: Land Developability (the Percentage of Land Available and Suitable for Development)
2.2. Neighborhood Structure and Spatial Weight Matrix. 2.2.1 What Is a Neighborhood Structure?
2.2.2 What Is a Spatial Weight Matrix?
2.2.3 Why Should We Use a Spatial Weight Matrix?
2.2.4 Which Spatial Weight Matrix Should We Use?
2.2.5 Issues With Spatial Weight Matrices and Solutions
2.2.6 Data Example
2.3 Spatial Autocorrelation, Dependence, and Heterogeneity
2.4 Exploratory Spatial Data Analysis
2.4.1 Visualizing Spatial Data
2.4.2 Moran’s I Statistic
2.4.3 Geary’s c Statistic
2.4.4 Local Indicator of Spatial Association (LISA)
2.3.4.1 Local Moran’s I Statistic
2.3.4.2 Local Geary’s c Statistic
2.3.4.3 G Statistic
2.4.5 Data Example
Descriptions of Images and Figures
3 Models Dealing With Spatial Dependence. Learning Objectives
3.1 Standard Linear Regression and Diagnostics for Spatial Dependence. 3.1.1 Standard Linear Regression
3.1.2 Diagnostics for Spatial Dependence
3.1.3 Model Evaluation
3.1.4 When Do We Need to Account for Spatial Dependence?
3.1.5 Data Example. 3.1.5.1 Population Change and Its Explanatory Variables
3.1.5.2 Model Diagnostics Using Standard Linear Regression Residuals
3.2 Spatial Lag Models
3.2.1 What Is a Spatial Lag Model?
3.2.2 Model Fitting via Maximum Likelihood
3.2.3 Model Diagnostics
3.2.4 When Is a Spatial Lag Model Needed?
3.2.5 Which Spatial Weight Matrix to Use in a Spatial Lag Model?
3.2.6 Cautions About Spatial Lag Models
3.2.7 Data Example
3.3 Spatial Error Models. 3.3.1 What Is a Spatial Error Model?
3.3.2 Model Fitting via Maximum Likelihood
3.3.3 Model Diagnostics
3.3.4 When Is a Spatial Error Model Needed?
3.3.5 Which Spatial Weight Matrix to Use in a Spatial Error Model?
3.3.6 Spatial Lag Models or Spatial Error Models?
3.3.7 Spatial Models: SAR, CAR, and SMA
3.3.8 Data Example
Descriptions of Images and Figures
4 Advanced Models Dealing With Spatial Dependence. Learning Objectives
4.1 Spatial Error Models With Spatially Lagged Responses. 4.1.1 What Is a Spatial Error Model With Spatially Lagged Responses?
4.1.2 Why and When Is an SEMSLR Needed?
4.1.3 Model Fitting
4.1.4 Which Spatial Weight Matrices Should Be Used in an SEMSLR?
4.1.5 Data Example
4.2 Spatial Cross-Regressive Models. 4.2.1 What Is a Spatial Cross-Regressive Model?
4.2.2 Model Fitting
4.2.3 When Is a Spatial Cross-Regressive Model Needed?
4.2.4 Which Spatial Weight Matrices Should Be Used in a Spatial Cross-Regressive Model?
4.2.5 Data Example
4.3 Multilevel Linear Regression
4.3.1 What Is an MLR Model?
4.3.2 MLR Model Diagnostics
4.3.3 Cautions About MLR Models
4.3.4 Data Example
Descriptions of Images and Figures
5 Models Dealing With Spatial Heterogeneity. Learning Objectives
5.1 Aspatial Regression Methods. 5.1.1 The Methods
5.1.2 Data Example
5.2 Spatial Regime Models. 5.2.1 The Methods
5.2.2 Model Fitting
5.2.3 Data Example
5.3 Geographically Weighted Regression. 5.3.1 The Limitations of Spatial Regime Models
5.3.2 Model Specifications of GWR
5.3.3 Spatial Weight Matrices for GWR Models
5.3.4 Model Fitting
5.3.5 Model Diagnostics
5.3.6 Strength and Limitation of GWR
5.3.7 Data Example
Descriptions of Images and Figures
6 Models Dealing With Both Spatial Dependence and Spatial Heterogeneity. Learning Objectives
6.1 Spatial Regime Lag Models
6.2 Spatial Regime Error Models
6.3 Spatial Regime Error and Lag Models
6.4 Model Fitting
6.5 Data Example
Descriptions of Images and Figures
7 Advanced Spatial Regression Models. Learning Objectives
7.1 Spatio-temporal Regression Models
7.1.1 Why Use Spatio-temporal Regression Models?
7.1.2 Two General Approaches for Spatio-temporal Regression Modeling
7.1.3 From Standard Linear Regression to Spatio-temporal Regression
7.1.4 How to Fit the Spatio-temporal Regression Model
7.1.5 Data Example
7.2 Spatial Regression Forecasting Models
7.2.1 What Is a Standard Regression Forecasting Model?
7.2.2 What Is a Spatial Regression Forecasting Model?
7.2.3 What Spatial Weight Matrices Should We Use in a Spatial Regression Forecasting Model?
7.2.4 Practical Considerations in Forecasting
7.2.5 Data Example
7.3 Geographically Weighted Regression for Forecasting. 7.3.1 The Methods
7.3.2 Data Example
Descriptions of Images and Figures
8 Practical Considerations for Spatial Data Analysis. Learning Objectives
8.1 Data Example of U.S. Poverty in R
8.1.1 Standard Exploratory Data Analysis
8.1.2 Standard Linear Regression. 8.1.2.1 Model Fitting
8.1.2.2 Model Selection
8.1.2.3 Model Diagnostics
8.1.3 Neighborhood Structure and Spatial Weight Matrix
8.1.4 Exploratory Spatial Data Analysis
8.1.4.1 Moran’s I and Geary’s c
8.1.4.2 Local Moran’s I
8.1.5 Spatial Linear Regression. 8.1.5.1 Diagnostics for Spatial Dependence
8.1.5.2 Spatial Lag Models
8.1.5.3 Spatial Error Models
8.1.5.4 Spatial SAR Models
8.1.5.5 Spatial CAR Models
8.2 General Procedure for Spatial Social Data Analysis
8.2.1 Reducing Data Dimensions
8.2.2 Selecting an Appropriate Spatial Weight Matrix
8.2.3 Spatial Regression Modeling With Spatial Dependence and Spatial Heterogeneity
8.2.4 Multitemporal Comparison of Variable Presence and Coefficient Influence
Descriptions of Images and Figures
Appendix A Spatial Data Sources
Traditional Websites
Interactive Web GIS
Glossary
References
Index
Отрывок из книги
I am very pleased to introduce Guangqing Chi and Jun Zhu’s volume Spatial Regression Models for the Social Sciences. Over the past few decades there has been a rapid increase in the use of spatial data to discern spatial effects in social science research due to the upsurge in the availability of geographically referenced data and the availability of user-friendly spatial data analysis software packages. However, many of the empirical studies remain at a stage that is descriptive and exploratory in nature, because many studies are geo-mapping or spatial overlay using geocoding technology. Regression-typed analyses exploring causal or correlational spatial effects are relatively sparse. Some studies employed multilevel regression models with random effects but failed to address the departure from the independent errors assumption at high levels. For contiguous geographic units, spatial lag models, spatial error models, spatial regime models, geographically weighted regression, spatio-temporal regression models, and geographically weighted regression for forecasting are more salient than the multilevel regression model. In essence, these models address the spatial dependence and heterogeneity issues more sophisticatedly by fully exploring the neighborhood structure embedded in an appropriately developed spatial weight matrix.
This volume describes all these latest advances in spatial regression models in a relatively accessible fashion for a broad range of readers conducting social scientific research. It describes the statistical principles, main features, strengths and limitations, and illustrating examples in a clear, succinct, and comprehensive fashion. The book is well organized to discuss lattice (or areal) data analysis using spatial regression methods.
.....
Our case study focuses on population changes during the period of 1970 to 2010 at the MCD level in the state of Wisconsin in relation to the spatial and temporal variations of a multitude of explanatory variables. Wisconsin, located in the upper Midwest of the United States, borders Minnesota, Iowa, Illinois, and Michigan, as well as the Mississippi River, Lake Michigan, and Lake Superior (Figure 1.1). Wisconsin contains 34.8 million acres (excluding the Mississippi River and Great Lakes areas in the state), and inland lakes constitute 3 percent of the state’s total surface area (Wisconsin Legislative Reference Bureau, 2005).
Figure 1.1 ⬢ The geography of Wisconsin, United States
.....