Multilevel Modeling in Plain Language
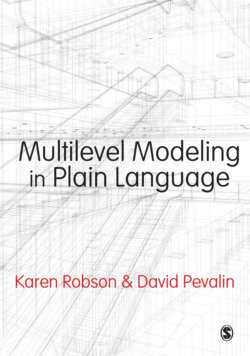
Реклама. ООО «ЛитРес», ИНН: 7719571260.
Оглавление
Karen Robson. Multilevel Modeling in Plain Language
Multilevel Modeling in Plain Language
Contents
About the Authors
ONE What Is Multilevel Modeling and Why Should I Use It? chapter contents
Mixing levels of analysis
Theoretical reasons for multilevel modeling
What are the advantages of using multilevel models?
Statistical reasons for multilevel modeling
Assumptions of OLS
Dependence among observations
Group estimates
Varying effects across contexts
Degrees of freedom and statistical significance
Software
How this book is organized
Chapter 1 takeaway points
TWO Random Intercept Models: When intercepts vary. chapter contents
A review of single-level regression
Nesting structures in our data
How many groups?
Sample sizes within groupings
What sorts of variables can be levels?
Getting starting with random intercept models
What do our findings mean so far?
Is this model a good one?
Calculating the ICC
Changing the grouping to schools
Is this model ‘better’?
Comparing ICCs
Adding Level 1 explanatory variables
Should we keep small Level 2 groupings?
Adding a dichotomous independent variable
Adding Level 2 explanatory variables
Group mean centring
Interactions
Level 1 dichotomous by Level 2 continuous
Level 1 continuous by Level 2 continuous
Model fit
What about R-squared?
Diagnostics
A further assumption and a short note on random and fixed effects
Chapter 2 takeaway points
Three Random Coefficient Models: When intercepts and coefficients vary. Contents
Getting started with random coefficient models
Trying a different random coefficient
Shrinkage
Fanning in and fanning out
Examining the variances
A dichotomous variable as a random coefficient
More than one random coefficient
A note on parsimony and fitting a model with multiple random coefficients
A model with one random and one fixed coefficient
Adding Level 2 variables
Residual diagnostics
First steps in model-building
Some tasters of further extensions to our basic models
Three-level models
Dichotomous dependent variables
Cross-classified models
Weighting
Where to next?
Chapter 3 takeaway points
Four Communicating Results to a Wider Audience. chapter contents
Creating journal-formatted tables
The fixed part of the model
The importance of the null model
Centring variables
Stata commands to make table-making easier
What do you talk about?
Models with random coefficients
What about graphs?
Cross-level interactions
Parting words
Chapter 4 takeaway points
References
Index
Отрывок из книги
Karen Robsonis Associate Professor of Sociology at York University. Her research areas include the barriers to postsecondary education for marginalized youth, intersectionality as a policy framework and critical race theory. She also has a strong interest in the analysis of large longitudinal data sets to examine issues around social mobility and the transition to postsecondary education. Dr Robson has also written key textbooks in the area of social research methods and the sociology of education, as well as several articles in various sociology journals.David Pevalinis Professor in the School of Health and Human Sciences and Dean of Postgraduate Research and Education at the University of Essex. His research focuses on macro and micro social inequalities in health. He co-authored (with Karen Robson) The Stata Survival Manual (Open University Press, 2009), co-edited (with David Rose) The Researcher’s Guide to the National Statistics Socio-economic Classification (SAGE, 2003), and authored research reports for the Department of Work and Pensions and the Health Development Agency. He has published papers in Journal of Health & Social Behavior, British Journal of Sociology, The Lancet, Public Health and Housing Studies.
This book is for a special type of user who is far more common than experts tend to recognize, or at least acknowledge. This book is for people who want to learn about this technique but are not all that interested in learning all the statistical equations and strange notations that are typically associated with teaching materials in this area. That is not to say we are flagrantly trying to promote bad research, because we are not. We are trying to demystify these types of approaches for people who are intimidated by technical language and mathematical symbols.
.....
b – unstandardized regression coefficients; s.e. – standard errors
aReference category is Australian Capital Territory
.....