Multi-Processor System-on-Chip 1
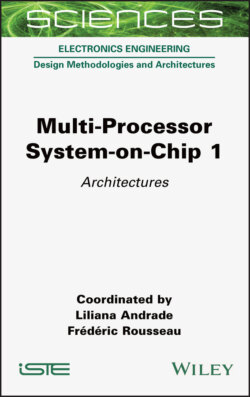
Реклама. ООО «ЛитРес», ИНН: 7719571260.
Оглавление
Liliana Andrade. Multi-Processor System-on-Chip 1
Table of Contents
List of Tables
List of Illustrations
Guide
Pages
Multi-Processor System-on-Chip 1. Architectures
Foreword
Acknowledgments
1. Processors for the Internet of Things
1.1. Introduction
1.2. Versatile processors for low-power IoT edge devices. 1.2.1.Control processing, DSP and machine learning
1.2.2.Configurability and extensibility
1.3. Machine learning inference
1.3.1.Requirements for low/mid-end machine learning inference
1.3.1.1. Neural network processing
1.3.1.2. Implementation requirements
1.3.2.Processor capabilities for low-power machine learning inference
1.3.3.A software library for machine learning inference
1.3.4.Example machine learning applications and benchmarks
1.4. Conclusion
1.5. References
2. A Qualitative Approach to Many-core Architecture
2.1. Introduction
2.2. Motivations and context. 2.2.1.Many-core processors
2.2.2.Machine learning inference
2.2.3.Application requirements
2.3. The MPPA3 many-core processor. 2.3.1.Global architecture
2.3.2.Compute cluster
2.3.3.VLIW core
2.3.4.Coprocessor
2.4. The MPPA3 software environments. 2.4.1.High-performance computing
2.4.2.KaNN code generator
2.4.3.High-integrity computing
2.5. Conclusion
2.6. References
3. The Plural Many-core Architecture – High Performance at Low Power
3.1. Introduction
3.2. Related works
3.3. Plural many-core architecture
3.4. Plural programming model
3.5. Plural hardware scheduler/synchronizer
3.6. Plural networks-on-chip
3.6.1.Scheduler NoC
3.6.2.Shared memory NoC
3.7. Hardware and software accelerators for the Plural architecture
3.8. Plural system software
3.9. Plural software development tools
3.10. Matrix multiplication algorithm on the Plural architecture
3.11. Conclusion
3.12. References
4. ASIP-Based Multi-Processor Systems for an Efficient Implementation of CNNs
4.1. Introduction
4.2. Related works
4.3. ASIP architecture
4.4. Single-core scaling
4.5. MPSoC overview
4.6. NoC parameter exploration
4.7. Summary and conclusion
4.8. References
5. Tackling the MPSoC Data Locality Challenge
5.1. Motivation
5.2. MPSoC target platform
5.3. Related work
5.4. Coherence-on-demand: region-based cache coherence
5.4.1.RBCC versus global coherence
5.4.2.OS extensions for coherence-on-demand
5.4.3.Coherency region manager
5.4.4.Experimental evaluations
5.4.5.RBCC and data placement
5.5. Near-memory acceleration
5.5.1.Near-memory synchronization accelerator
5.5.2.Near-memory queue management accelerator
5.5.3.Near-memory graph copy accelerator
5.5.4.Near-cache accelerator
5.6. The big picture
5.7. Conclusion
5.8. Acknowledgments
5.9. References
6. mMPU: Building a Memristor-based General-purpose In-memory Computation Architecture
6.1. Introduction
6.2. MAGIC NOR gate
6.3. In-memory algorithms for latency reduction
6.4. Synthesis and in-memory mapping methods
6.4.1.SIMPLE
6.4.2.SIMPLER
6.5. Designing the memory controller
6.6. Conclusion
6.7. References
7. Removing Load/Store Helpers in Dynamic Binary Translation
7.1. Introduction
7.2. Emulating memory accesses
7.3. Design of our solution
7.4. Implementation
7.4.1.Kernel module
7.4.2.Dynamic binary translation
7.4.3.Optimizing our slow path
7.5. Evaluation
7.5.1. QEMUemulation performance analysis
7.5.2.Our performance overview
7.5.3.Optimized slow path
7.6. Related works
7.7. Conclusion
7.8. References
8. Study and Comparison of Hardware Methods for Distributing Memory Bank Accesses in Many-core Architectures
8.1. Introduction. 8.1.1.Context
8.1.2.MPSoC architecture
8.1.3.Interconnect
8.2. Basics on banked memory. 8.2.1.Banked memory
8.2.2.Memory bank conflict and granularity
8.2.3.Efficient use of memory banks: interleaving
8.2.3.1. Access patterns
8.2.3.1.1. Random access pattern
8.2.3.1.2. Sequential access pattern
8.2.3.1.3. Stride access pattern
8.3. Overview of software approaches
8.3.1.Padding
8.3.2.Static scheduling of memory accesses
8.3.3.The need for hardware approaches
8.4. Hardware approaches. 8.4.1.Prime modulus indexing
8.4.2.Interleaving schemes using hash functions
8.4.2.1. XOR scheme
8.4.2.2. PRIM – pseudo-randomly interleaved memory
8.4.2.2.1. How it works
8.4.2.3. PRIM example – Intel LLC Complex Addressing
8.5. Modeling and experimenting
8.5.1.Simulator implementation
8.5.2.Implementation of the Kalray MPPA cluster interconnect
8.5.3.Objectives and method
8.5.4.Results and discussion. 8.5.4.1. Mapping MOD 16
8.5.4.2. Mapping MOD 17
8.5.4.3. Mapping PRIM 47
8.5.4.4. Addition of memory banks
8.5.4.5. Access reordering
8.5.4.6. Combination of access reordering and addition of memory banks
8.5.4.7. Discussion
8.6. Conclusion
8.7. References
9. Network-on-Chip (NoC): The Technology that Enabled Multi-processor Systems-on-Chip (MPSoCs)
9.1. History: transition from buses and crossbars to NoCs
9.1.1.NoC architecture
9.1.1.1. NoC layers
9.1.1.1.1. NoC layered approach benefits
9.1.1.2. NoC modularity and hierarchical architecture
9.1.1.3. NoC trade-offs
9.1.2.Extending the bus comparison to crossbars
9.1.3.Bus, crossbar and NoC comparison summary and conclusion
9.2. NoC configurability
9.2.1.Human-guided design flow
9.2.2.Physical placement awareness and NoC architecture design
9.3. System-level services
9.3.1.Quality-of-service (QoS) and arbitration
9.3.2.Hardware debug and performance analysis
9.3.3.Functional safety and security. 9.3.3.1. NoC functionality, failure modes and safety mechanisms
9.3.3.1.1. NoC functional safety analysis and FMEDA automation
9.3.3.2. NoC contributions to system-level security
9.4. Hardware cache coherence
9.4.1.NoC protocols, semantics and messaging
9.5. Future NoC technology developments
9.5.1.Topology synthesis and floorplan awareness
9.5.2.Advanced resilience and functional safety for autonomous vehicles
9.5.3.Alternatives to von Neumann architectures for SoCs
9.5.3.1. Massively parallel AI/machine learning architectures
9.5.3.2. Hierarchical cache coherence
9.5.4.Chiplets and multi-die NoC connectivity
9.5.5.Runtime software automation
9.5.6.Instrumentation, diagnostics and analytics for performance, safety and security
9.6. Summary and conclusion
9.7. References
10. Minimum Energy Computing via Supply and Threshold Voltage Scaling
10.1. Introduction
10.2. Standard-cell-based memory for minimum energy computing
10.2.1.Overview of low-voltage on-chip memories. 10.2.1.1. Low-voltage SRAM
10.2.1.2. Standard-cell-based memory (SCM)
10.2.2.Design strategy for area- and energy-efficient SCMs
10.2.2.1. Architectural-level design strategy
10.2.2.2. Physical placement optimization
10.2.2.3. Cell-level optimization
10.2.3.Hybrid memory design towards energy- and area-efficient memory systems
10.2.4.Body biasing as an alternative to power gating
10.3. Minimum energy point tracking
10.3.1.Basic theory. 10.3.1.1. Necessary conditions for minimum energy computing
10.3.1.2. Sub- and near-threshold regions
10.3.1.3. Super-threshold region
10.3.2.Algorithms and implementation
10.3.3.OS-based approach to minimum energy point tracking
10.4. Conclusion
10.5. Acknowledgments
10.6. References
11. Maintaining Communication Consistency During Task Migrations in Heterogeneous Reconfigurable Devices
11.1. Introduction. 11.1.1.Reconfigurable architectures
11.1.2.Contribution
11.2. Background
11.2.1.Definitions
11.2.1.1. Hardware context switch
11.2.1.2. Hardware task context
11.2.1.3. Communication channel
11.2.2.Problem scenario and technical challenges
11.3. Related works. 11.3.1.Hardware context switch
11.3.2.Communication management
11.4. Proposed communication methodology in hardware context switching
11.5. Implementation of the communication management on reconfigurable computing architectures
11.5.1.Reconfigurable channels in FIFO
11.5.2.Communication infrastructure
11.6. Experimental results. 11.6.1.Setup
11.6.2.Experiment scenario
11.6.3.Resource overhead
11.6.4.Impact on the total execution time
11.6.5.Impact on the context extract and restore time
11.6.6.System responsiveness to context switch requests
11.6.7.Hardware task migration between heterogeneous FPGAs
11.7. Conclusion
11.8. References
List of Authors
Author Biographies
Index. A
B, C
D
E, F
G, H
I
L
M
N
P
Q, R
S
T, V
WILEY END USER LICENSE AGREEMENT
Отрывок из книги
To my parents, sisters and husband, the loves and pillars of my life.
.....
After selecting the right processor, the next question is how to arrive at an efficient software implementation of the targeted machine learning inference application. For this purpose, we present a library of reusable software modules to show how these are implemented efficiently on the ARC EM9D processor.
Table 1.2. Supported kernels in the embARC MLI library
.....