Multi-Processor System-on-Chip 2
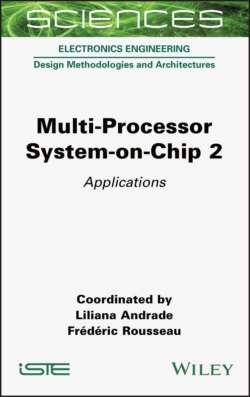
Реклама. ООО «ЛитРес», ИНН: 7719571260.
Оглавление
Liliana Andrade. Multi-Processor System-on-Chip 2
Table of Contents
List of Tables
List of Illustrations
Guide
Pages
Multi-Processor System-on-Chip 2. Applications
Foreword
Acknowledgments
1. From Challenges to Hardware Requirements for Wireless Communications Reaching 6G
1.1. Introduction
1.2. Breadth of workloads
1.2.1. Vision, trends and applications
1.2.2. Standard specifications
1.2.2.1. Processing deadline variability
1.2.2.2. Data throughput variability
1.2.2.3. Specification summary
1.2.3. Outcome of workloads
1.3. GFDM algorithm breakdown
1.3.1. Equation
1.3.2. Dataflow processing graph and matrix representation
1.3.3. Pseudo-code
1.4. Algorithm precision requirements and considerations
1.5. Implementation
1.5.1. Implementation considerations
1.5.2. Design space exploration
1.5.3. Measurements for low-end and high-end use cases
1.6. Conclusion
1.7. Acknowledgments
1.8. References
2. Towards Tbit/s Wireless Communication Baseband Processing: When Shannon meets Moore
2.1. Introduction
2.2. Role of microelectronics
2.3. Towards 1 Tbit/s throughput decoders
2.3.1. Turbo decoder
2.3.2. LDPC decoder
2.3.3. Polar decoder
2.4. Conclusion
2.5. Acknowledgments
2.6. References
3. Automation for Industry 4.0 by using Secure LoRaWAN Edge Gateways
3.1. Introduction
3.2. Security in IIoT
3.3. LoRaWAN security in IIoT
3.4. Threat model
3.4.1. LoRaWAN attack model
3.4.2. IIoT node attack model
3.5. Trusted boot chain with STM32MP1
3.5.1. Trust base of node
3.5.2. Trusted firmware in STM32MP1
3.5.3. Trusted execution environments and OP-TEE
3.5.4. OP-TEE scheduling considerations
3.5.5. OP-TEE memory management
3.5.6. OP-TEE client API
3.5.7. TEE internal core API
3.5.8. Root and chain of trust
3.5.9. Hardware unique key
3.5.10. Secure clock
3.5.11. Cryptographic operations
3.6. LoRaWAN gateway with STM32MP1
3.7. Discussion and future scope
3.8. Acknowledgments
3.9. References
4. Accelerating Virtualized Distributed NVMe Storage in Hardware
4.1. Introduction
4.1.1. Virtualization and traditional hypervisors
4.1.2. Hyperconverged versus disaggregated cloud architectures
4.1.2.1. Degree of resource virtualization
4.1.2.2. Scalability of disaggregation and HCI
4.1.2.3. Management software
4.1.3. NVMe flash storage
4.2. Motivation: NVMe storage for the cloud
4.2.1. Motivation for a new hypervisor
4.2.2. Motivation for accelerating disaggregated storage
4.3. Design
4.3.1. Optimizing the hypervisor I/O operations
4.3.1.1. Design of the NexVisor hypervisor
4.3.2. Design of accelerated disaggregated storage
4.3.2.1. Virtualization of physical storage drives
4.3.2.2. On-demand virtual disk management
4.3.2.3. Offloaded virtual disk replication
4.3.2.4. Offloaded virtual disk copy
4.3.2.5. Offloaded virtual disk snapshot
4.3.2.6. Networked protocol. 4.3.2.6.1. ATA over Ethernet (AoE)
4.3.2.6.2. Networked storage operations
4.3.2.7. Storage virtualization
4.3.2.7.1. LUN table
4.3.2.7.2. Virtual-to-physical translation table structure
4.3.2.7.3. Dirty extent bitmap
4.4. Implementation
4.4.1. The NexVisor platform
4.4.2. Accelerated disaggregated storage
4.4.2.1. Hardware specification
4.4.2.2. Architecture
4.4.2.3. Storage node
4.5. Results
4.5.1. Sequential reads
4.5.2. Sequential writes
4.5.3. Sequential reads on one NVMe drive
4.5.4. Network performance
4.6. Conclusion
4.7. References
5. Modular and Open Platform for Future Automotive Computing Environment
5.1. Introduction
5.2. Outline of this approach
5.2.1. Centralized computation, distributed data
5.2.2. Modularity and heterogeneity
5.2.2.1. Modularity and heterogeneity at the hardware level
5.2.2.2. Modularity and heterogeneity at the software level
5.2.3. Tools for specification, configuration and integration
5.3. Results
5.3.1. Hardware platform
5.3.1.1. Physical computing unit
5.3.1.2. Physical interface unit
5.3.1.3. Network ETH/TSN
5.3.2. FACE SW architecture. 5.3.2.1. Layered stack structure
5.3.2.1.1. Board support package
5.3.2.1.2. Hypervisor
5.3.2.1.3. Operating system
5.3.2.1.4. Middleware
5.3.2.1.5. The application
5.3.2.2. Virtual prototyping framework
5.3.3. FACE Tool Suite
5.3.3.1. The functional architecture modeling feature
5.3.3.2. About model validation features
5.3.3.3. About the deployment feature
5.3.3.4. About the integration validation feature
5.4. Use case
5.4.1. Adaptive braking system
5.5. Conclusion
5.6. References
6. Post-Moore Datacenter Server Architecture
6.1. Introduction
6.2. Background: today’s blades are from the desktops of the 1980s
6.3. Memory-centric server design
6.4. Data management accelerators
6.5. Integrated network controllers
6.6. References
7. SESAM: A Comprehensive Framework for Cyber–Physical System Prototyping
7.1. Introduction
7.2. An overview of the SESAM platform
7.2.1. Multi-abstraction system prototyping
7.2.2. Assessing extra-functional system properties. 7.2.2.1. Power modeling
7.2.2.2. Reliability
7.3. VPSim: fast and easy virtual prototyping
7.3.1. Writing peripherals in Python
7.3.2. The ModelProvider interface
7.3.3. QEMU support
7.3.3.1. Integration methodology
7.3.3.2. Improvements to QEMU
7.3.4. Online simulation monitoring
7.3.5. Acceleration methods
7.4. Hybrid prototyping
7.4.1. Co-simulation mode
7.4.2. Co-emulation mode
7.4.3. Runtime performance analysis and debugging features
7.5. FMI for co-simulation
7.5.1. Functional mock-up interface
7.5.2. VPSim integration in FMI co-simulation
7.6. Conclusion
7.7. References
8. StaccatoLab: A Programming and Execution Model for Large-scale Dataflow Computing
8.1. Introduction
8.2. Static dataflow
8.2.1. Synchronous dataflow
8.2.2. Cyclo-static dataflow
8.2.3. Dataflow graph transformations
8.3. Dynamic dataflow
8.3.1. Data-dependent dataflow
8.3.2. Non-determinate dataflow
8.4. Dataflow execution models
8.4.1. A brief review of dataflow theory
8.4.2. The StaccatoLab execution model
8.5. StaccatoLab
8.5.1. Dataflow graph description and analysis
8.5.2. Verilog synthesis
8.6. Large-scale dataflow computing?
8.6.1. What kind of applications?
8.6.2. Why effective?
8.6.3. Why efficient?
8.7. Acknowledgments
8.8. References
9. Smart Cameras and MPSoCs
9.1. Introduction
9.2. Early VLSI video processors
9.3. Video signal processors
9.4. Accelerators
9.5. From VSP to MPSoC
9.6. Graphics processing units
9.7. Neural networks and tensor processing units
9.8. Conclusion
9.9. References
10. Software Compilation and Optimization Techniques for Heterogeneous Multi-core Platforms
10.1. Introduction
10.2. Dataflow modeling. 10.2.1. General concepts
10.2.2. Process networks
10.2.3. C for process networks
10.2.3.1. Channels
10.2.3.2. Processes
10.2.3.3. Parallelism types
10.3. Source-to-source-based compiler infrastructure
10.3.1. Design rationale
10.3.2. Implementation strategy
10.4. Software distribution
10.4.1. KPN analysis
10.4.2. Static KPN mapping
10.4.3. Hybrid KPN mapping
10.5. Results
10.5.1. Applications and experiences
10.5.2. Retargetability
10.6. Conclusion
10.7. References
List of Authors
Author Biographies
Index
A
B, C
D
E
F
G
H
I
L
M
N
O
P
Q, R
S
T
V
W
WILEY END USER LICENSE AGREEMENT
Отрывок из книги
To my parents, sisters and husband, the loves and pillars of my life.
Liliana ANDRADE
.....
Figure 1.9 with a new set of coefficients that invert the first filtering, followed by DFT. Finally, after successful demodulation, the demodulated stream is compared with the reference and the error vector magnitude is measured.
.....