Modern Characterization of Electromagnetic Systems and its Associated Metrology
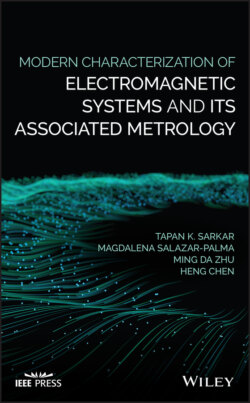
Реклама. ООО «ЛитРес», ИНН: 7719571260.
Оглавление
Magdalena Salazar-Palma. Modern Characterization of Electromagnetic Systems and its Associated Metrology
Table of Contents
List of Tables
List of Illustrations
Guide
Pages
Modern Characterization of Electromagnetic Systems and Its Associated Metrology
Preface
Acknowledgments
Tribute to Tapan K. Sarkar by Magdalena Salazar Palma, Ming Da Zhu, and Heng Chen
1 Mathematical Principles Related to Modern System Analysis. Summary
1.1 Introduction
1.2 Reduced‐Rank Modelling: Bias Versus Variance Tradeoff
1.3 An Introduction to Singular Value Decomposition (SVD) and the Theory of Total Least Squares (TLS) 1.3.1 Singular Value Decomposition
1.3.2 The Theory of Total Least Squares
1.4 Conclusion
References
2 Matrix Pencil Method (MPM) Summary
2.1 Introduction
2.2 Development of the Matrix Pencil Method for Noise Contaminated Data
2.2.1 Procedure for Interpolating or Extrapolating the System Response Using the Matrix Pencil Method
2.2.2 Illustrations Using Numerical Data. 2.2.2.1 Example 1
2.2.2.2 Example 2
2.3 Applications of the MPM for Evaluation of the Characteristic Impedance of a Transmission Line
2.4 Application of MPM for the Computation of the S‐Parameters Without any A Priori Knowledge of the Characteristic Impedance
2.5 Improving the Resolution of Network Analyzer Measurements Using MPM
2.6 Minimization of Multipath Effects Using MPM in Antenna Measurements Performed in Non‐Anechoic Environments
2.6.1 Application of a FFT‐Based Method to Process the Data
2.6.2 Application of MPM to Process the Data
2.6.3 Performance of FFT and MPM Applied to Measured Data
2.7 Application of the MPM for a Single Estimate of the SEM‐Poles When Utilizing Waveforms from Multiple Look Directions
2.8 Direction of Arrival (DOA) Estimation Along with Their Frequency of Operation Using MPM
2.9 Efficient Computation of the Oscillatory Functional Variation in the Tails of the Sommerfeld Integrals Using MPM
2.10 Identification of Multiple Objects Operating in Free Space Through Their SEM Pole Locations Using MPM
2.11 Other Miscellaneous Applications of MPM
2.12 Conclusion
Appendix 2A Computer Codes for Implementing MPM. MATLAB CODES. Example 2.1
Example 2.2
References
3 The Cauchy Method. Summary
3.1 Introduction
3.2 Procedure for Interpolating or Extrapolating the System Response Using the Cauchy Method
3.3 Examples to Estimate the System Response Using the Cauchy Method. 3.3.1 Example 1
3.3.2 Example 2
3.3.3 Example 3
3.4 Illustration of Extrapolation by the Cauchy Method. 3.4.1 Extending the Efficiency of the Moment Method Through Extrapolation by the Cauchy Method
3.4.2 Interpolating Results for Optical Computations
3.4.3 Application to Filter Analysis
3.4.4 Broadband Device Characterization Using Few Parameters
3.5 Effect of Noise Contaminating the Data and Its Impact on the Performance of the Cauchy Method
3.5.1 Perturbation of Invariant Subspaces
3.5.2 Perturbation of the Solution of the Cauchy Method Due to Additive Noise
3.5.3 Numerical Example
3.6 Generating High Resolution Wideband Response from Sparse and Incomplete Amplitude‐Only Data
3.6.1 Development of the Interpolatory Cauchy Method for Amplitude‐Only Data
3.6.2 Interpolating High Resolution Amplitude Response
3.7 Generation of the Non‐minimum Phase Response from Amplitude‐Only Data Using the Cauchy Method
3.7.1 Generation of the Non‐minimum Phase
3.7.2 Illustration Through Numerical Examples
3.8 Development of an Adaptive Cauchy Method. 3.8.1 Introduction
3.8.2 Adaptive Interpolation Algorithm
3.8.3 Illustration Using Numerical Examples
3.8.4 Summary
3.9 Efficient Characterization of a Filter
3.10 Extraction of Resonant Frequencies of an Object from Frequency Domain Data
3.11 Conclusion
Appendix 3A MATLAB Codes for the Cauchy Method
References
4 Applications of the Hilbert Transform – A Nonparametric Method for Interpolation/Extrapolation of Data. Summary
4.1 Introduction
4.2 Consequence of Causality and Its Relationship to the Hilbert Transform
4.3 Properties of the Hilbert Transform
4.4 Relationship Between the Hilbert and the Fourier Transforms for the Analog and the Discrete Cases
4.5 Methodology to Extrapolate/Interpolate Data in the Frequency Domain Using a Nonparametric Methodology
4.6 Interpolating Missing Data
4.7 Application of the Hilbert Transform for Efficient Computation of the Spectrum for Nonuniformly Spaced Data
4.7.1 Formulation of the Least Square Method
4.7.2 Hilbert Transform Relationship
4.7.3 Magnitude Estimation
4.8 Conclusion
References
5 The Source Reconstruction Method. Summary
5.1 Introduction
5.2 An Overview of the Source Reconstruction Method (SRM)
5.3 Mathematical Formulation for the Integral Equations
5.4 Near‐Field to Far‐Field Transformation Using an Equivalent Magnetic Current Approach
5.4.1 Description of the Proposed Methodology
5.4.2 Solution of the Integral Equation for the Magnetic Current
5.4.3 Numerical Results Utilizing the Magnetic Current
5.4.4 Summary
5.5 Near‐Field to Near/Far‐Field Transformation for Arbitrary Near‐Field Geometry Utilizing an Equivalent Electric Current
5.5.1 Description of the Proposed Methodology
5.5.2 Numerical Results Using an Equivalent Electric Current
5.5.3 Summary
5.6 Evaluating Near‐Field Radiation Patterns of Commercial Antennas
5.6.1 Background
5.6.2 Formulation of the Problem
5.6.3 Results for the Near‐field To Far‐field Transformation. 5.6.3.1 A Base Station Antenna
5.6.3.2 NF to FF Transformation of a Pyramidal Horn Antenna
5.6.3.3 Reference Volume of a Base Station Antenna for Human Exposure to EM Fields
5.6.4 Summary
5.7 Conclusions
References
6 Planar Near‐Field to Far‐Field Transformation Using a Single Moving Probe and a Fixed Probe Arrays. Summary
6.1 Introduction
6.2 Theory
6.3 Integral Equation Formulation
6.4 Formulation of the Matrix Equation
6.5 Use of an Magnetic Dipole Array as Equivalent Sources
6.6 Sample Numerical Results
6.7 Summary
6.8 Differences between Conventional Modal Expansion and the Equivalent Source Method for Planar Near‐Field to Far‐Field Transformation. 6.8.1 Introduction
6.8.2 Modal Expansion Method
6.8.3 Integral Equation Approach
6.8.4 Numerical Examples
6.8.5 Summary
6.9 A Direct Optimization Approach for Source Reconstruction and NF‐FF Transformation Using Amplitude‐Only Data
6.9.1 Background
6.9.2 Equivalent Current Representation
6.9.3 Optimization of a Cost Function
6.9.4 Numerical Simulation
6.9.5 Results Obtained Utilizing Experimental Data
6.9.6 Summary
6.10 Use of Computational Electromagnetics to Enhance the Accuracy and Efficiency of Antenna Pattern Measurements Using an Array of Dipole Probes
6.10.1 Introduction
6.10.2 Development of the Proposed Methodology
6.10.3 Philosophy of the Computational Methodology
6.10.4 Formulation of the Integral Equations
6.10.5 Solution of the Integro‐Differential Equations
6.10.6 Sample Numerical Results
6.10.6.1 Example 1
6.10.6.2 Example 2
6.10.6.3 Example 3
6.10.6.4 Example 4
6.10.7 Summary
6.11 A Fast and Efficient Method for Determining the Far Field Patterns Along the Principal Planes Using a Rectangular Probe Array
6.11.1 Introduction
6.11.2 Description of the Proposed Methodology
6.11.3 Sample Numerical Results
6.11.3.1 Example 1
6.11.3.2 Example 2
6.11.3.3 Example 3
6.11.3.4 Example 4
6.11.4 Summary
6.12 The Influence of the Size of Square Dipole Probe Array Measurement on the Accuracy of NF‐FF Pattern
6.12.1 Illustration of the Proposed Methodology Utilizing Sample Numerical Results
6.12.1.1 Example 1
6.12.1.2 Example 2
6.12.1.3 Example 3
6.12.1.4 Example 4
6.12.2 Summary
6.13 Use of a Fixed Probe Array Measuring Amplitude‐Only Near‐Field Data for Calculating the Far‐Field
6.13.1 Proposed Methodology
6.13.2 Sample Numerical Results. 6.13.2.1 Example 1
6.13.2.2 Example 2
6.13.2.3 Example 3
6.13.2.4 Example 4
6.13.3 Summary
6.14 Probe Correction for Use with Electrically Large Probes
6.14.1 Development of the Proposed Methodology
6.14.2 Formulation of the Solution Methodology
6.14.3 Sample Numerical Results
6.15 Conclusions
References
7 Spherical Near‐Field to Far‐Field Transformation. Summary
7.1 An Analytical Spherical Near‐Field to Far‐Field Transformation
7.1.1 Introduction
7.1.2 An Analytical Spherical Near‐Field to Far‐Field Transformation
7.1.3 Numerical Simulations. 7.1.3.1 Synthetic Data
7.1.3.2 Experimental Data
7.1.4 Summary
7.2 Radial Field Retrieval in Spherical Scanning for Current Reconstruction and NF–FF Transformation
7.2.1 Background
7.2.2 An Equivalent Current Reconstruction from Spherical Measurement Plane
7.2.3 The Radial Electric Field Retrieval Algorithm
7.2.4 Results Obtained Using This Formulation
7.2.4.1 Simulated Data
7.2.4.2 Using Measured Data
7.3 Conclusion
Appendix 7A A Fortran Based Computer Program for Transforming Spherical Near‐Field to Far‐Field
References
8 Deconvolving Measured Electromagnetic Responses. Summary
8.1 Introduction
8.2 The Conjugate Gradient Method with Fast Fourier Transform for Computational Efficiency. 8.2.1 Theory
8.2.2 Numerical Results
8.3 Total Least Squares Approach Utilizing Singular Value Decomposition. 8.3.1 Theory
8.3.2 Total Least Squares (TLS)
8.3.3 Numerical Results
8.4 Conclusion
References
9 Performance of Different Functionals for Interpolation/Extrapolation of Near/Far‐Field Data. Summary
9.1 Background
9.2 Approximating a Frequency Domain Response by Chebyshev Polynomials
9.3 The Cauchy Method Based on Gegenbauer Polynomials
9.3.1 Numerical Results and Discussion
9.3.1.1 Example of a Horn Antenna
9.3.1.2 Example of a 2‐element Microstrip Patch Array
9.3.1.3 Example of a Parabolic Antenna
9.4 Near‐Field to Far‐Field Transformation of a Zenith‐Directed Parabolic Reflector Using the Ordinary Cauchy Method
9.5 Near‐Field to Far‐Field Transformation of a Rotated Parabolic Reflector Using the Ordinary Cauchy Method
9.6 Near‐Field to Far‐Field Transformation of a Zenith‐Directed Parabolic Reflector Using the Matrix Pencil Method
9.7 Near‐Field to Far‐Field Transformation of a Rotated Parabolic Reflector Using the Matrix Pencil Method
9.8 Conclusion
References
10 Retrieval of Free Space Radiation Patterns from Measured Data in a Non‐Anechoic Environment. Summary
10.1 Problem Background
10.2 Review of Pattern Reconstruction Methodologies
10.3 Deconvolution Method for Radiation Pattern Reconstruction
10.3.1 Equations and Derivation
10.3.2 Steps Required to Implement the Proposed Methodology
10.3.3 Processing of the Data
10.3.4 Simulation Examples
10.3.4.1 Example I: One PEC Plate Serves as a Reflector
10.3.4.2 Example II: Two PEC Plates Now Serve as Reflectors
10.3.4.3 Example III: Four Connected PEC Plates Serve as Reflectors
10.3.4.4 Example IV: Use of a Parabolic Reflector Antenna as the AUT
10.3.5 Discussions on the Deconvolution Method for Radiation Pattern Reconstruction
10.4 Effect of Different Types of Probe Antennas
10.4.1 Numerical Examples
10.4.1.1 Example I: Use of a Yagi Antenna as the Probe
10.4.1.2 Example II: Use of a Parabolic Reflector Antenna as the Probe
10.4.1.3 Example III: Use of a Dipole Antenna as the Probe
10.5 Effect of Different Antenna Size
10.6 Effect of Using Different Sizes of PEC Plates
10.7 Extension of the Deconvolution Method to Three‐Dimensional Pattern Reconstruction
10.7.1 Mathematical Characterization of the Methodology
10.7.2 Steps Summarizing for the Methodology
10.7.3 Processing the Data
10.7.4 Results for Simulation Examples
10.7.4.1 Example I: Four Wide PEC Plates Serve as Reflectors
10.7.4.2 Example II: Four PEC Plates and the Ground Serve as Reflectors
10.7.4.3 Example III: Six Plates Forming an Unclosed Contour Serve as Reflectors
10.7.4.4 Example IV: Antenna Measurement in a Closed PEC Box
10.7.4.5 Example V: Six Dielectric Plates Forming a Closed Contour Simulating a Room
10.8 Conclusion
Appendix A: Data Mapping Using the Conversion between the Spherical Coordinate System and the Cartesian Coordinate System
Appendix B: Description of the 2D‐FFT during the Data Processing
References
Index. a
b
c
d
e
f
g
h
i
k
l
m
n
o
p
q
r
s
t
w
y
z
WILEY END USER LICENSE AGREEMENT
Отрывок из книги
Tapan K. Sarkar†
.....
where U1 is a column matrix of size 20×1 and so is V1. The rank one approximation of A is seen in Figure 1.2.
The advantage of the SVD is that an error in the reconstruction of the image can be predicted without actually knowing the actual solution. This is accomplished by looking at the second largest singular value. The result is not good and we did not expect it to be. So now if we perform a Rank‐2 reconstruction for the image, it will be given by
.....