Linear and Convex Optimization
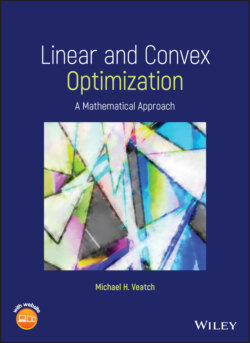
Реклама. ООО «ЛитРес», ИНН: 7719571260.
Оглавление
Michael H. Veatch. Linear and Convex Optimization
Table of Contents
List of Tables
List of Illustrations
Guide
Pages
Linear and Convex Optimization. A Mathematical Approach
Preface
About the Companion Website
1 Introduction to Optimization Modeling. 1.1 Who Uses Optimization?
1.2 Sending Aid to a Disaster
Components of an Optimization Problem
Solving a Linear Program Graphically
1.2.1 The Modeling Process
1.3 Optimization Terminology
1.4 Classes of Mathematical Programs
1.4.1 Linear Programs
General Form of a Linear Program
1.4.2 Integer Programs
General Form of an Integer Program
1.4.3 Nonlinear Programs
General Form of a Nonlinear Program
Problems
2 Linear Programming Models
2.1 Resource Allocation
2.1.1 Production Process Models
2.2 Purchasing and Blending
2.2.1 Online Advertising Markets
2.2.2 Blending Problems
2.3 Workforce Scheduling
2.4 Multiperiod Problems
2.4.1 Multiperiod Financial Models
2.5 Modeling Constraints
2.6 Network Flow
2.6.1 Transportation Problems
Transportation Problem
2.6.2 Minimum Cost Network Flow
Minimum Cost Network Flow Problem
2.6.3 Shortest Path Problems
Problems
3 Linear Programming Formulations
3.1 Changing Form
3.1.1 Slack Variables
Equivalent Forms of Linear Programs
3.2 Linearization of Piecewise Linear Functions
Minimax Problems
3.2.1 Linearization without Adding Constraints
3.2.2 Goal Programming
3.3 Dynamic Programming
Problems
4 Integer Programming Models
4.1 Quantitative Variables and Fixed Costs
4.1.1 Fixed Costs
4.2 Set Covering
4.3 Logical Constraints and Piecewise Linear Functions
4.3.1 Job Shop Scheduling
4.3.2 Linearization of Piecewise Linear Objectives
4.4 Additional Applications
4.4.1 Supermarket Markdowns
4.4.2 Warehouse Location and Transshipment
4.4.3 Locating Disaster Recovery Centers
4.5 Traveling Salesperson and Cutting Stock Problems
4.5.1 Cutting Stock Problem
Problems
5 Iterative Search Algorithms
5.1 Iterative Search and Constructive Algorithms
5.1.1 Constructive Algorithms
5.1.2 Exact and Heuristic Algorithms
5.1.3 Traveling Salesperson Heuristics
Nearest Neighbor
Cheapest Edge
Cheapest Insertion
2‐Opt
5.2 Improving Directions and Optimality
5.2.1 Feasible Directions and Step Size
5.2.2 Optimality Conditions
5.3 Computational Complexity and Correctness
5.3.1 Computational Complexity
Problems
6 Convexity
6.1 Convex Sets
6.2 Convex and Concave Functions
6.2.1 Combining Convex Functions
Problems
7 Geometry and Algebra of LPs
7.1 Extreme Points and Basic Feasible Solutions
Basic Solution
7.1.1 Degeneracy
Degenerate Basic Solution
7.2 Optimality of Extreme Points
Example 7.3
7.3 Linear Programs in Canonical Form
Standard Form of Linear Programs
Canonical Form of Linear Programs
7.3.1 Basic Solutions in Canonical Form
7.4 Optimality Conditions
7.5 Optimality for General Polyhedra
7.5.1 Representation of Polyhedra
Extreme Ray
Problems
8 Duality Theory
8.1 Dual of a Linear Program
Dual of a Linear Program
Rules for Forming a Dual
8.2 Duality Theorems
8.3 Complementary Slackness
8.4 Lagrangian Duality
Properties of the Lagrangian Dual
8.5 Farkas' Lemma and Optimality
Problems
9 Simplex Method
Simplex Method
9.1 Simplex Method From a Known Feasible Solution
9.1.1 Finding an Improving Direction
9.1.2 Determining the Step Size
Maximum Step Sizea
9.1.3 Updating the Basis
9.1.4 Simplex Method
Algorithm 9.1 (Simplex Method)
9.1.5 A Comment on Names
9.2 Degeneracy and Correctness
Smallest Subscript Pivoting Rule
9.3 Finding an Initial Feasible Solution
9.3.1 Artificial Variables in the Basis
9.3.2 Two‐Phase Simplex Method
Algorithm 9.2 (Two‐Phase Simplex Method)
9.4 Computational Strategies and Speed
9.4.1 Number of Iterations Required
9.4.2 Revised Simplex Method
Algorithm 9.3 (Revised Simplex Method)
9.4.3 Simplex Tableaus
Algorithm 9.4 (Tableau Simplex Method)
9.4.4 Other Implementation Issues
Problems
10 Sensitivity Analysis
10.1 Graphical Sensitivity Analysis
10.1.1 Changes in the Right‐hand Side
Sensitivity to Constraint Right‐hand Sides
10.1.2 Changes in the Objective Function
10.2 Shadow Prices and Reduced Costs
10.2.1 Changes in the Right‐hand Side
10.2.2 Sign of Shadow Prices
10.2.3 Changes in the Objective Function
10.2.4 Sensitivity Analysis Examples
10.2.5 Degeneracy
10.2.6 Parametric Programming
10.3 Economic Interpretation of the Dual
Problems
11 Algorithmic Applications of Duality
11.1 Dual Simplex Method
Algorithm 11.1 (Dual Simplex Method)
11.2 Network Simplex Method
11.2.1 Node‐arc Incidence Matrix
11.2.2 Tree Solutions
11.2.3 Network Simplex
Algorithm 11.2 (Simplex Method for Network Flow Problems)
11.2.4 Transportation Problem
Example 11.6
11.3 Primal‐Dual Interior Point Method
11.3.1 Newton's Method
11.3.2 Step Size
11.3.3 Choosing the Complementary Slackness Parameter
Algorithm 11.3 (Primal‐Dual Path Following Algorithm)
11.3.4 Barrier Function Interpretation
Problems
12 Integer Programming Theory
12.1 Linear Programming Relaxations
Lemma 12.1
12.2 Strong Formulations
12.2.1 Aggregate Constraints
12.2.2 Bounding Integer Variables
12.3 Unimodular Matrices
12.3.1 Network Flow Problems
12.3.2 Unimodularity
Problems
13 Integer Programming Algorithms
13.1 Branch and Bound Methods
13.1.1 Branching and Trees
13.1.2 Choosing a Subproblem
Algorithm 13.1 (Branch and Bound)
13.1.3 Mixed Integer Programs
13.1.4 Integer Lagrangian Relaxations
13.2 Cutting Plane Methods
13.2.1 Gomory Cutting Plane Method
Algorithm 13.2 (Gomory's Cutting Plane Algorithm)
13.2.2 Generating Valid Inequalities by Rounding
Example 13.4
13.2.3 Valid Inequalities for Binary Variables
Problems
14 Convex Programming: Optimality Conditions
14.1 KKT Optimality Conditions
14.1.1 Equality Constraints
14.1.2 Inequality Constraints
14.1.3 Convexity
Convex Program
14.1.4 KKT Conditions with Nonnegativity Constraints
14.1.5 Examples
14.2 Lagrangian Duality
14.2.1 Geometry of Primal and Dual Functions
14.2.2 Sensitivity Analysis
Problems
15 Convex Programming: Algorithms
15.1 Convex Optimization Models
15.2 Separable Programs
15.3 Unconstrained Optimization
15.3.1 Gradient Search
Algorithm 15.1 (Gradient Search (Maximization))
15.3.2 Newton's Method
15.3.3 Quasi‐Newton Methods
15.4 Quadratic Programming
15.5 Primal‐dual Interior Point Method
15.5.1 Barrier Problem
15.5.2 The Newton Step
15.5.3 Step Size and Slackness Parameter
15.5.4 Primal‐dual Interior Point Algorithm
Algorithm 15.2 (Primal‐dual Interior Point Algorithm)
Problems
A Linear Algebra and Calculus Review. A.1 Sets and Other Notation
A.2 Matrix and Vector Notation
A.3 Matrix Operations
A.4 Matrix Inverses
A.5 Systems of Linear Equations
A.6 Linear Independence and Rank
A.7 Quadratic Forms and Eigenvalues
A.8 Derivatives and Convexity
Bibliography
Index
WILEY END USER LICENSE AGREEMENT
Отрывок из книги
Michael H. Veatch
Gordon College
.....
Now we introduce more terminology for constrained optimization problems, as well as summarizing the terms introduced in the last section. These problems are called mathematical programs. The variables that we optimize over are the decision variables. They may be continuous variables, meaning that they can take on values in the real numbers, including fractions, or they may discrete variables that can only take on integer values. The function being optimized is the objective function. The domain over which the function is optimized is the set of points that satisfy the constraints, which can be equations or inequalities. We distinguish two kinds of constraints. Functional constraints can have any form, while variable bounds restrict the allowable values of one variable. Many problems have nonnegativity constraints as their variable bounds. In (1.1), the food constraint is listed with the functional constraints, making three functional constraints plus two nonnegativity constraints (variable bounds), but it is also a bound, so the problem can also be said to have two functional constraints and three bounds.
.....