Convex Optimization
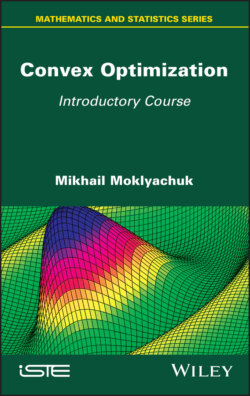
Реклама. ООО «ЛитРес», ИНН: 7719571260.
Оглавление
Mikhail Moklyachuk. Convex Optimization
Table of Contents
List of Illustrations
Guide
Pages
Convex Optimization. Introductory Course
Notations
Introduction
1. Optimization Problems with Differentiable Objective Functions. 1.1. Basic concepts
1.2. Optimization problems with objective functions of one variable
1.3. Optimization problems with objective functions of several variables
1.4. Constrained optimization problems. 1.4.1. Problems with equality constraints
1.4.2. Problems with equality and inequality constraints
1.5. Exercises
2. Convex Sets. 2.1. Convex sets: basic definitions
2.2. Combinations of points and hulls of sets
2.3. Topological properties of convex sets
2.4. Theorems on separation planes and their applications. 2.4.1. Projection of a point onto a set
2.4.2. Separation of two sets
2.5. Systems of linear inequalities and equations
2.6. Extreme points of a convex set
2.7. Exercises
3. Convex Functions. 3.1. Convex functions: basic definitions
3.2. Operations in the class of convex functions
3.3. Criteria of convexity of differentiable functions
3.4. Continuity and differentiability of convex functions
3.5. Convex minimization problem
3.6. Theorem on boundedness of Lebesgue set of a strongly convex function
3.7. Conjugate function
3.8. Basic properties of conjugate functions
3.9. Exercises
4. Generalizations of Convex Functions. 4.1. Quasi-convex functions
4.1.1. Differentiate quasi-convex functions
4.1.2. Operations that preserve quasi-convexity
4.1.3. Representation in the form of a family of convex functions
4.1.4. The maximization problem for quasi-convex functions
4.1.5. Strictly quasi-convex functions
4.1.6. Strongly quasi-convex functions
4.2. Pseudo-convex functions
4.3. Logarithmically convex functions
4.3.1. Properties of logarithmically convex functions
4.3.2. Integration of logarithmically concave functions
4.4. Convexity in relation to order
4.5. Exercises
5. Sub-gradient and Sub-differential of Finite Convex Function
5.1. Concepts of sub-gradient and sub-differential
5.2. Properties of sub-differential of convex function
5.3. Sub-differential mapping
5.4. Calculus rules for sub-differentials
5.5. Systems of convex and linear inequalities
5.6. Exercises
6. Constrained Optimization Problems. 6.1. Differential conditions of optimality
6.2. Sub-differential conditions of optimality
6.3. Exercises
6.4. Constrained optimization problems. 6.4.1. Principle of indeterminate Lagrange multipliers
6.4.2. Differential form of the Kuhn-Tucker theorem
6.4.3. Second-order conditions of optimality
6.5. Exercises
6.6. Dual problems in convex optimization
6.6.1. Kuhn-Tucker vector
6.6.2. Dual optimization problems
6.6.3.Kuhn-Tucker theorem for non-differentiable functions
6.6.4. Method of perturbations
6.6.5. Economic interpretations of the Kuhn-Tucker vector
6.7. Exercises
Solutions, Answers and Hints. Solutions for Chapter 1
Solutions for Chapter 2
Solutions for Chapter 3
References
Index. A
C
D
E
F,H
I, J
K
L
M, N
O,P
Q, R
S
T, V, W, Y, Z
WILEY END USER LICENSE AGREEMENT
Отрывок из книги
Series Editor Nikolaos Limnios
.....
be not equal to zero. Then there exist numbers ε > 0, δ > 0, K > 0 such that for any y = (y1, … , ys), ∥y∥ ≤ ε we can find x = (x1, … , xs), which satisfies conditions ∥x∥ < δ, , ∥x∥ ≤ K ∥y∥.
PROOF.– We prove the Lagrange theorem by contradiction. Suppose that the stationarity condition
.....