Statistical Approaches for Hidden Variables in Ecology
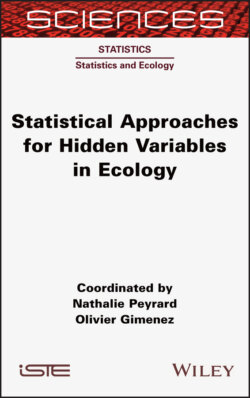
Реклама. ООО «ЛитРес», ИНН: 7719571260.
Оглавление
Nathalie Peyrard. Statistical Approaches for Hidden Variables in Ecology
Table of Contents
List of Tables
List of Illustrations
Guide
Pages
Statistical Approaches for Hidden Variables in Ecology
Introduction
I.1. Hidden variables in ecology
I.2. Hidden variables in statistical modeling
I.3. Statistical methods
I.4. Approach and structure of our work
I.5. Directions for further perspectives
I.6. References
1. Trajectory Reconstruction and Behavior Identification Using Geolocation Data
1.1. Introduction
1.1.1. Reconstructing a real trajectory from imperfect observations
1.1.2. Identifying different behaviors in movement
1.2. Hierarchical models of movement. 1.2.1. Trajectory reconstruction model. 1.2.1.1. Overview
1.2.1.2. Inference
1.2.1.3. Filtering and smoothing a trajectory
1.2.2. Activity reconstruction model. 1.2.2.1. Overview
1.2.2.2. Choice of observation metric
1.2.2.3. Covariates inclusion
1.2.2.4. Example: a three-state HMM with Gaussian emission
1.2.2.5. Inference
1.2.2.6. Reconstruction of hidden states
1.2.2.7. Choosing the number of activities
1.3. Case study: masked booby, Sula dactylatra (originals)
1.3.1. Data
1.3.2. Projection
1.3.3. Data smoothing
1.3.4. Identification of different activities through movement
1.3.4.1. Definition of metrics
1.3.4.2. Defining the starting point of the algorithm
1.3.5. Results. 1.3.5.1. Characterization of hidden states
1.3.5.2. State uncertainty
1.3.5.3. Inclusion of the nest distance covariate
1.3.5.4. Choosing a number of states
1.4. References
2. Detection of Eco-Evolutionary Processes in the Wild: Evolutionary Trade-Offs Between Life History Traits
2.1. Context
2.2. The correlative approach to detecting evolutionary trade-offs in natural settings: problems
2.2.1. Mechanistic and statistical modeling as a means of accessing hidden variables
2.3. Case study. 2.3.1. Costs of maturing and migration for survival: a theoretical approach
2.3.1.1. The system
2.3.1.2. Modeling life history decisions and proximal signals at the individual level
2.3.1.3. Modeling evolutionary trade-offs
2.3.1.4. Implementation and evaluation
2.3.1.5. Identifying trade-offs
2.3.2. Growth/reproduction trade-off in trees
2.3.2.1. The studied system
2.3.2.2. Resource simulation at individual level
2.3.2.3. Modeling evolutionary trade-offs
2.3.2.4. Implementation and fitting
2.3.2.5. Trade-off identification
2.4. References
3. Studying Species Demography and Distribution in Natural Conditions: Hidden Markov Models
3.1. Introduction
3.2. Overview of HMMs
3.3. HMM and demography. 3.3.1. General overview
3.3.2. Case study: estimating the prevalence of dog–wolf hybrids with uncertain individual identification
3.4. HMM and species distribution. 3.4.1. General case
3.4.2. Case study: estimating the distribution of a wolf population with species identification errors and heterogeneous detection
3.5. Discussion
3.6. Acknowledgments
3.7. References
4. Inferring Mechanistic Models in Spatial Ecology Using a Mechanistic-Statistical Approach
4.1. Introduction
4.2. Dynamic systems in ecology. 4.2.1. Temporal models
4.2.2. Spatio-temporal models without reproduction
4.2.3. Spatio-temporal models with reproduction
4.2.4. Numerical solution
4.3. Estimation. 4.3.1. Estimation principle
4.3.2. Parameter estimation
4.3.3. Estimation of latent processes
4.3.4. Mechanistic-statistical models
4.4. Examples. 4.4.1. The COVID-19 epidemic in France
4.4.2. Wolf (Canis lupus) colonization in southeastern France
4.4.3. Estimating dates and locations of the introduction of invasive strains of watermelon mosaic virus
4.5. References
5. Using Coupled Hidden Markov Chains to Estimate Colonization and Seed Bank Survival in a Metapopulation of Annual Plants
5.1. Introduction
5.2. Metapopulation model for plants: introduction of a dormant state. 5.2.1. Dependency structure in the model
5.2.2. Distributions defining the model
5.2.3. Parameterizing the model
5.2.4. Linking the parameters of the model with the ecological parameters of the dynamics of an annual plant
5.2.5. Estimation
5.2.6. Model selection
5.3. Dynamics of weed species in cultivated parcels. 5.3.1. Dormancy and weed management in agroecosystems
5.3.2. Description of the data set
5.3.3. Comparison with an HMM with independent patches
5.3.4. Influence of crops on weed dynamics
5.4. Discussion and conclusion
5.5. Acknowledgments
5.6. References
6. Using Latent Block Models to Detect Structure in Ecological Networks
6.1 Introduction
6.2. Formalism
6.3. Probabilistic mixture models for networks
6.3.1. SBMs for unipartite networks
6.3.2. Stochastic block model for bipartite networks
6.4. Statistical inference
6.4.1. Estimation of parameters and clustering
6.4.2. Model selection
6.5. Application
6.5.1. Food web
6.5.2. A bipartite plant–pollinator network
6.6. Conclusion
6.7. References
7. Latent Factor Models: A Tool for Dimension Reduction in Joint Species Distribution Models
7.1. Introduction
7.2. Joint species distribution models
7.3. Dimension reduction with latent factors
7.4. Inference
7.5. Ecological interpretation of latent factors
7.6. On the interpretation of JSDMs
7.7. Case study. 7.7.1. Introduction of the dataset
7.7.2. R package used
7.7.3. Implementation and convergence diagnosis
7.7.4. Results and discussion
7.8. Conclusion
7.9. References
8. The Poisson Log-Normal Model: A Generic Framework for Analyzing Joint Abundance Distributions
8.1. Introduction
8.2. The Poisson log-normal model. 8.2.1. The model
8.2.1.1. Covariates and offsets
8.2.1.2. Modeling the variance-covariance matrix
8.2.1.3. Additional notation
8.2.2. Inference method
8.2.2.1. Inference in latent variable models
8.2.2.2. Variational approximation
8.2.3. Dimension reduction
8.2.3.1. Presentation of the model
8.2.3.2. Model inference
8.2.3.3. Using the results of the estimation process
8.2.4. Inferring networks of interaction
8.2.4.1. PLN as a Gaussian graphical model
8.2.4.2. Inference
8.3. Data analysis: marine species. 8.3.1. Description of the data
8.3.2. Effects due to site and date
8.3.3. Dimension reduction
8.3.4. Inferring ecological interactions
8.3.4.1. Penalty effect
8.3.4.2. Robustness of edges
8.3.4.3. Inferred network
8.4. Discussion
8.5. Acknowledgments
8.6. References
9. Supervised Component-Based Generalized Linear Regression: Method and Extensions
9.1. Introduction
9.2. Models and methods. 9.2.1. Supervised component-based generalized linear regression
9.2.1.1. The SCGLR approach
9.2.1.2. Notion of structural relevance
9.2.1.3. Estimation method for a single-component model
9.2.1.4. Construction of higher rank components
9.2.2. Thematic supervised component-based generalized linear regression (THEME-SCGLR)
9.2.2.1. One component per thematic group
9.2.2.2. Multiple components per thematic group
9.2.3. Mixed SCGLR. 9.2.3.1. Beyond independence
9.2.3.2. Presentation of the model
9.2.3.3. Estimation method
9.3. Case study: predicting the abundance of 15 common tree species in the forests of Central Africa
9.3.1. The SCGLR method: a direct approach
9.3.2. THEME-SCGLR: improved characterization of predictive components
9.3.3. Mixed-SCGLR: taking account of the concession effect
9.4. Discussion
9.5. References
10. Structural Equation Models for the Study of Ecosystems and Socio-Ecosystems
10.1. Introduction. 10.1.1. Ecological background
10.1.2. Methodological problem
10.1.3. Case study: biodiversity in a managed forest
10.2. Structural equation model
10.2.1. Hypotheses and general structure of an SEM. 10.2.1.1. Data set
10.2.1.2. Formalization of the measure model and relational model. 10.2.1.2.1. Measurement model
10.2.1.2.2. Relational model
10.2.2. Likelihood and estimation in an SEM
10.2.2.1. Likelihood of parameters (A, B, ΣF ) in an SEM
10.2.2.2. Maximum likelihood estimator in an SEM
10.2.2.2.1. Parameter constraints
10.2.2.2.2. Identifiability problem
10.2.3. Fit quality and nested SEM tests
10.2.3.1. Goodness-of-fit test
10.2.3.1.1. Asymptotic test
10.2.3.1.2. Re-sampling test
10.2.3.2. Comparing two nested SEMs using likelihood ratios and re-sampling
10.3. Case study: biodiversity in managed forests. 10.3.1. Preliminary steps
10.3.2. Evaluating the measurement model alone
10.3.3. Evaluating the relational model
10.3.3.1. Convergence of estimation
10.3.3.2. Comparison with confirmatory factor analysis
10.3.4. Significance of parameters in the relational model
10.3.5. Findings
10.4. Discussion
10.4.1. A confirmatory approach
10.4.2. Gaussian framework
10.4.3. Centered-reduced observed variables
10.4.4. Structural constraints
10.4.5. Use of resampling
10.5. Acknowledgments
10.6. References
List of Authors
Index
WILEY END USER LICENSE AGREEMENT
Отрывок из книги
Statistics, Field Directors – Nikolaos Limnios, Kerrie Mengersen
.....
Nevertheless, in the case of a new species or study environment, it can be hard to establish an initial idea of the number of hidden states; in this case, an approach based on statistical, rather than biological, criteria may be preferred. In statistics, this is known as a model choice problem, with “model” corresponding to a number of components.
One well-known model choice criterion is the Akaike information criteria (AIC) (Akaike 1973), which can be used to ensure that the number of parameters fits the data as well as possible. The aim is not simply to identify a parsimonious model, which fits the data; states need to be as different as possible, meaning that the problem is also one of classification. A new state should only be added if it is sufficiently distinct from other states. In this case, the integrated complete likelihood (ICL) criterion may be used (Biernacki et al. 2000; Bacci et al. 2014).
.....