Self-Service Data Analytics and Governance for Managers
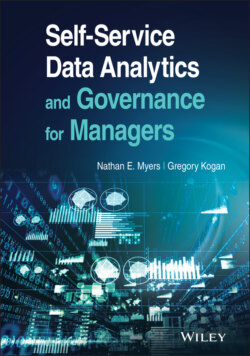
Реклама. ООО «ЛитРес», ИНН: 7719571260.
Оглавление
Nathan E. Myers. Self-Service Data Analytics and Governance for Managers
Table of Contents
List of Exhibits
Guide
Pages
Self-Service Data Analytics and Governance for Managers
Preface
Acknowledgments
About the Authors
Introduction
CHAPTER 1 Setting the Stage. Impact
Emergence of Data Analytics
Self-Service Data Analytics
Employee/Analyst/Operator Perspective
Managers' Perspectives
Executives' Strategic Perspectives
Arguments for Self-Service Data Analytics Tooling
Need for Self-Service Data Analytics Governance
CHAPTER 2 Emerging AI and Data Analytics Tooling and Disciplines
Introduction to Data Analytics Tooling
Internet of Things
Cloud Storage and Cloud Computing
Artificial Intelligence
Blockchain and Distributed Ledger Technology
Robotic Process Automation
Machine Learning
Optical Character Recognition/Intelligent Character Recognition
Natural Language Processing
Self-Service Data Analytics
Dashboarding and Visualization
Discussion with Paul Paris – CEO, Lash Affair
Conclusion
CHAPTER 3 Why Governance Is Essential and the Self-Service Data Analytics Governance Gap
Governance Is Essential
Inputs
Process Quality
Firm-Wide Build Inventory
Risk Assessments
Rationalizing Fragmented Governance
Mature Governance Frameworks
Data Governance
COBIT Framework for IT Enterprise Governance
Sarbanes–Oxley Act
COSO Internal Control Framework
Applicable COSO Monitoring principles:
COSO ERM (2017) – Integrating Strategy with Performance
SAS No. 1, Section 210
SAS 70, SSAE 16, SysTrust Engagements
Model Risk Governance
Self-Service Data Analytics Governance Gap
Structures Needed to Fill the Governance Gap
Governance and Oversight
Planning and Alignment
Policies and Procedures
Development Standards
Conclusion
Note
CHAPTER 4 Self-Service Data Analytics Project Governance
Securing Sponsorship and Establishing the Governance Committee
Extending Governance Precepts from Established Frameworks
Ensuring Input Integrity
Data Governance Committee
Data Asset Inventory
Data Lineage
Data Quality Review Procedures
Resolution Sequence
Opportunity Capture, Benefits Case, Sizing, and Prioritization
Self-Service Data Analytics Build Inventory Must Be Maintained
Self-Service Data Analytics Tooling Is Tactical by Nature
Project Development Audit Trail Must Be Captured and Retained
Reference End-User Analytics Tooling and Workflows in Process Documentation
Model Risk Governance
Organizational and Functional Goal Alignment
Adequate Training for Developers and End-Users
Establishment of Risk Assessment Criteria
Discussion with Jitesh Ghai, Chief Product Officer at Informatica
Conclusion
Notes
CHAPTER 5 Self-Service Data AnalyticsRisk Governance
Setting Risk Appetite in an Environment of Changing Performance Expectations
Data Analytics Risk Governance Enhances Value Creation
Data Analytics Tool Selection Drives the Level of Partnership with IT
Alignment of Finance Function Goals with Digital Transformation Capabilities
Data Analytics Risk Governance
Assessing Risks in the Analytics and Automation Environment
Seven Relevant Risks to Data Analytics
Impact Scale for the Analytics Risk Environment
Likelihood Scale for the Analytics Risk Environment
Vulnerability Scale for the Automation and Analytics Risk Environment
Speed of Onset Scale for the Automation and Analytics Risk Environment
Developing the Portfolio View of Risk
Developing Risk Responses and Controls in the Analytics and Automation Environment
Interview with Two Big Four Audit Executives
Conclusion
Notes
CHAPTER 6 Self-Service Data Analytics Capabilities in Action with Alteryx
Alteryx Functionality. Understanding the Data
Importing the Data
Cleansing the Data
Blending and Joining
Enriching Data
Data Transformation
Data Visualization
Alteryx in Action. Whole Market Trend Analysis
Industries: Comparative Performance
Information Technology: Trend Analysis Over Five Years
Top Performers in the Information Technology Industry
Apple Inc.: Sales and Earnings Trend Analysis
Interview with Dean Stoecker, Co-Founder and Executive Chairman, Alteryx, Inc
Conclusion
CHAPTER 7 Process Discovery: Identify Opportunities, Evaluate Feasibility, and Prioritize
Business Case for Systems versus Self-Service Data Analytics
Process Discovery Phases and Methodology
Top-Down: Organizational Context
SWOT Analysis
Porter's Five Forces
Porter's Value Chain
Top-Down: Functional View
Suppliers, Inputs, Processes, Outputs, and Customers: Functional-Level
Middle Management: Define the Processes
Suppliers, Inputs, Processes, Outputs, and Customers: Process-Level
Bottom-Up: Map and Measure
Process Map (Swim Lanes)
Measure (Elapsed Time)
Automated Process Discovery
Conclusion
Notes
CHAPTER 8 Opportunity Capture and Heatmaps
Opportunity Inventory Matrix
Capturing Problem Statements
Uncovering Use Cases
Solutioning
Benefits Cases
Sizing Build Efforts
Project Acceptance Criteria and Organizational Constraints
Automation Heatmap and Prioritization
Workflow Tooling
Use Case Library
Multiplier Effect of Replication Opportunities on Project Benefits Case
Conclusion
Glossary
Index
WILEY END USER LICENSE AGREEMENT
Отрывок из книги
NATHAN E. MYERS
GREGORY KOGAN
.....
Skip ahead two years and suddenly you feel exposed. Which builds are being relied upon by regulators? Which builds are relied upon by customers? Did the individual who put them in place have adequate knowledge of the underlying processes to build reliably and effectively? Were they well versed with the data analytics tools and technologies deployed? Were such builds adequately tested? Precisely how many builds exist across the organization? If key software vendors raise the price of basic licenses, is any of the work salvageable for migration to a new platform? You are being challenged by key internal clients on the quality of the financial deliverables that your team prepares, but you learn your team has simply been taking analytics build outputs at face value. They no longer understand the longhand processing steps that have been automated, as the team has experienced significant turnover over the last two years. This has resulted in the tools effectively becoming “black boxes,” where the transformation steps embedded in them are obscured and difficult to decipher. You fear that your organization has fallen into a common trap; by moving away from regimented technology release cycles toward a decentralized change model, you have lost control.
Governance, or lack thereof, is perhaps the strongest harbinger of control and stability, in an environment where self-service data analytics is prevalent. Effective governance is particularly critical due to the expected growth pattern of data analytics adoption, once the floodgates are opened. Without the benefit of governance to keep pace with the decentralization of development capabilities, organizations can find themselves struggling to demonstrate process effectiveness; they may not have clear visibility into the degree to which they are dependent on off-the-shelf software applications; they may lack adequate information upon which to base risk assessments; or they may get it abjectly wrong. Governance must provide guidelines aimed at ensuring the quality and integrity of processing inputs; that processing solutions implemented are appropriate, adequately tested, and operate effectively; that minimum standards of project documentation are met; and that risk assessment and mitigation activities can be demonstrated in the thoughtful deployment of analytics tooling.
.....