Experimental Design and Statistical Analysis for Pharmacology and the Biomedical Sciences
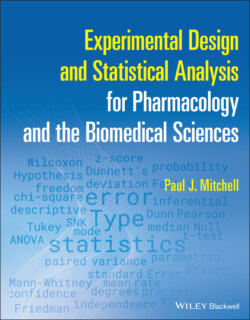
Реклама. ООО «ЛитРес», ИНН: 7719571260.
Оглавление
Paul J. Mitchell. Experimental Design and Statistical Analysis for Pharmacology and the Biomedical Sciences
Table of Contents
List of Tables
List of Illustrations
Guide
Pages
Experimental Design and Statistical Analysis for Pharmacology and the Biomedical Sciences
Biography
Acknowledgements. Homo Sapiens – Part 1
Statistical Packages
Homo Sapiens – Part 2
Foreword
Example 1:
Example 2:
Example 3:
1 Introduction. Experimental design: the important decision about statistical analysis
Experimental design process
Statistical analysis: why are statistical tests required? The eye‐ball test!
The structure of this book: Descriptive and Inferential Statistics
2 So, what are data?
Data handling and presentation
Text
Tables
Figures
3 Numbers; counting and measuring, precision, and accuracy
Precision and accuracy
Example 3.1
Errors in measurement
Independent observations or duplicate/triplicate/quadruplicate? That is the question! Example 3.2
Example 3.3
Example 3.4
Independent and paired data sets
4 Data collection: sampling and populations, different types of data, data distributions. Sampling and populations
The Central Limit Theorem
Types of data
Classification of data distributions
So why do we need to understand data distribution?
5 Descriptive statistics; measures to describe and summarise data sets
Parametric Descriptive Statistics and the Normal Distribution
Degrees of Freedom – a simple analogy
Variance
Standard Deviation
Standard Error of the Mean
Example output from statistical software
6 Testing for normality and transforming skewed data sets
Care!
Transforming skewed data sets to approximate a normal distribution
Transforming positive skew
Transforming negative skew
Removing outliers: Grubbs's test
QQ plots
Example output from statistical software
7 The Standard Normal Distribution
8 Non‐parametric descriptive statistics
Non‐parametric descriptive statistics
Example output from statistical software
9 Summary of descriptive statistics: so, what values may I use to describe my data? Introduction: the most important question to answer in statistical analysis!
What type of data do I have?
Taking the first steps to data description and analysis
Strategy for descriptive statistics
Example data. 1 Categorical data
2 Parametric data (use of arithmetic and geometric mean values)
a Calculation of individual drug potency by determining the EC50 values from the raw data for each tissue
b Calculation of the average concentration‐effect curve
c Calculation of the average EC50 value as a measure of drug potency
3 Non‐parametric data
Example output from statistical software
Decision Flowchart 1: Descriptive Statistics – Parametric v Non‐Parametric data
10 Introduction to inferential statistics. Overview
Hypothesis testing
Experimental design
One‐tailed or two‐tailed, that is the question!
Type 1 and Type 2 errors
Power analysis calculations and sample size
Single comparison between 2 groups
Comparing several groups of data
Association between 2 groups of data
Relationship between categorical variables
11 Comparing two sets of data – Independent t‐test. The Independent t-test
Equal group sizes
Unequal group sizes
Interpretation of the t statistic
Example 11.1 Equal group sizes
Example 11.2 Unequal group sizes
Example output from statistical software
12 Comparing two sets of data – Paired t‐test. The Paired t-test
Interpretation of the t statistic
Example 12.1 Paired data
Example output from statistical software
13 Comparing two sets of data – independent non‐parametric data
The Wilcoxon Rank Sum test and Mann-Whitney U-test. Example 13.1 Independent data
The Wilcoxon Rank-Sum test
The Mann–Whitney U‐test
Example output from statistical software
14 Comparing two sets of data – paired non‐parametric data
The Wilcoxon Signed‐Rank test
Example 14.1 Paired data
Example output from statistical software
15 Parametric one‐way analysis of variance. Introduction
One‐Way Analysis of Variance
Source of variance; Total, Between-Group, and Within-Group
Example 15.1
Total variance
Between‐Group variance
Within‐Group variance
Relationship between the F‐ratio and probability
So, what do we do next?
Multiple pairwise comparisons; post hoc and a priori analysis
Example 15.2 Experiment 1: All Means comparisons
Example 15.3 Experiment 2: Control group comparisons
Data analysis step 1: one‐way ANOVA
Data analysis step 2: Post hoc analysis
Experiment 1:
Experiment 2:
Example output from statistical software
16 Repeated measure analysis of variance. Introduction
Repeated measures ANOVA
Assessing sphericity
Example 16.1 Repeated measures data
Grand mean and sum of squares
Grand variance
Within‐subject sum of squares and variance
The model sum of squares and variance
Residual sum of squares and variance
Mauchly's test
Post hoc tests
All Means comparisons
Control group comparisons
Example output from statistical software
17 Complex Analysis of Variance Models
Part A: choice of suitable Analysis of Variance models
Between‐Group Factors
Within‐Group Factors
Using spreadsheets in experimental design
Example 17.1 One Between‐Group Factor only
Example 17.2 Two Between‐Group Factors only
Example 17.3 Three Between‐Group Factors only
Example 17.4 Zero Between‐Group Factor plus one Within‐Group Factor
Example 17.5 One Between‐Group Factor plus one Within‐Group Factor
Example 17.6 Two Between‐Group Factors plus one Within‐Group Factor
Part B: choice of suitable post hoc pairwise comparisons
Example 17.7 Pairwise comparisons following one‐way ANOVA (see Ex 17.1)
Example 17.8 Pairwise comparisons following two‐way ANOVA (see Ex 17.2)
Main effect of Between‐Group Factor 1 (Acute Treatment)
Main effect of Between‐Group Factor 2 (Pre‐treatment)
Example 17.9 Pairwise comparisons following three‐way ANOVA (see Ex 17.3)
Example 17.10 Pairwise comparisons following Repeated Measures ANOVA (one Within‐Group Factor only) (see Ex 17.4)
Mixed ANOVA models
Example 17.11 Pairwise comparisons following one‐way ANOVA with Repeated Measures (see Ex 17.5)
Main effect of the Between‐Group Factor
Main effect of the Within‐Group Factor
Interaction between the Between‐Group and Within‐Group Factors
Example 17.12 Pairwise comparisons following two‐way ANOVA with Repeated Measures (see Ex 17.6)
Main Effects. Main Effect of Between‐Group Factor 1
Main effect of Between‐Group Factor 2
Main effect of Within‐Group Factor
Low‐order interactions. Between‐Group Factor 1 * Between‐Group Factor 2
Between‐Group Factor 1 * Within‐Group Factor
Between‐Group Factor 2 * Within‐Group Factor
Bonferroni and alternative correction procedures
Holme correction procedure
General comments on complex ANOVA models
Example output from statistical software. Example data: Two Between‐Group Factors = Two‐way ANOVA
Example data: one Between‐Group Factor plus one Within‐Group Factor = One-way ANOVA with Repeated Measures
18 Non‐parametric ANOVA. Overview
Example 18.1 Non‐parametric one‐way ANOVA: The Kruskal–Wallis test
Example 18.2 Non‐parametric two‐way ANOVA: The Scheirer–Ray–Hare extension
Total Sum of Squares; SSQtotal
Between‐Group sum of squares; SSQBetween
Within‐Group sum of squares (error term); SSQWithin
Rows sum of squares; SSQRows
Columns sum of squares; SSQColumns
Interaction sum of squares; SSQInteraction
Total variance
Example 18.3 Non‐parametric Repeated Measures ANOVA: The Friedman test
Limitations of non-parametric ANOVA models
Multiple pairwise comparisons following non‐parametric ANOVA
Multiple pairwise comparisons using the Mann–Whitney U‐test
Multiple pairwise comparisons using the Wilcoxon Signed‐Rank test
Multiple pairwise comparisons using a variant of Dunn's test
Independent Groups (following Kruskal–Wallis ANOVA) (see Example 18.1) Example 18.4 Multiple comparisons between all groups
Example 18.5 Multiple comparisons between a control group and test groups
Paired Groups (following Friedman's ANOVA) (see Example 18.3) Example 18.6 Multiple comparisons between all groups
Example 18.7 Multiple comparisons between a control group and test groups
Example output from statistical software
19 Correlation analysis
Bivariate correlation analysis of parametric data
Example 19.1 Positive correlation
Example 19.2 Negative correlation
Example 19.3 Mixed correlation
Correlation analysis of non‐parametric data. Example 19.4 Non‐parametric correlation with tied ranks
Spearman Rank Correlation analysis with tied ranks
Example 19.5 Non‐parametric rank correlation with no tied ranks. Spearman Rank Correlation analysis with no tied ranks
Example 19.6 Kendall's non‐parametric rank correlation
Example output from statistical software
20 Regression analysis
Linear regression
Example 20.1 Linear regression without data transformation
Example 20.2 Linear regression with single variable transformation
Example 20.3 Linear regression with dual variable transformation
Example output from statistical software
21 Chi‐square analysis
Assumptions of chi‐square analysis
Example 21.1 3 × 3 contingency table and χ 2
Care!
Example 21.2 χ 2 and expected frequencies
Fisher's Exact test
Example 21.3 2 × 2 contingency tables
Yate's correction
Risk, relative risk, and odds ratio
Example 21.4 Patterning across contingency tables and χ 2
Example output from statistical software
Decision Flowchart 3: Inferential Statistics – Tests of Association
22 Confidence intervals
Overview
Example 22.1 Calculating confidence intervals for large sample sizes (n ≥ 30)
Example 22.2 Calculating confidence intervals for small sample sizes (n< 30)
Statistical significance of confidence intervals
Example 22.3 Confidence interval of differences between two independent groups
Example 22.4 Confidence interval of differences between two paired groups
Example 22.5 Confidence intervals and correlation
23 Permutation test of exact inference
Rationale
Example 23.1 A simple hypothetical data set
24 General Linear Model
The General Linear Model and Descriptive Statistics
The General Linear Model and Inferential Statistics
Appendix A Data distribution: probability mass function and probability density functions
A.1 Binomial distribution (Chapter 4.iii, Figure 4.4): Probability mass function
A.2 Exponential distribution (Chapter 4.v1, Figure 4.5): Probability density function
A.3 Normal distribution (Chapter 4.vii, Figure 4.7): Probability density function
A.4 Chi‐square distribution (Chapter 4.viii, Figure 4.8): Probability density function
A.5 Student t‐distribution (Chapter 4.ix, Figure 4.9): Probability density function
A.6 F distribution (Chapter 4.x, Figure 4.10): Probability density function
Appendix B Standard normal probabilities
Appendix C Critical values of the t‐distribution
Appendix D Critical values of the Mann–Whitney U‐statistic
Appendix E Critical values of the F distribution
Appendix F Critical values of chi‐square distribution
Appendix G Critical z values for multiple non‐parametric pairwise comparisons
Appendix H Critical values of correlation coefficients
Summary Decision Flowchart
Index
WILEY END USER LICENSE AGREEMENT
Отрывок из книги
Paul J. Mitchell
Department of Pharmacy and Pharmacology University of Bath Bath, UK
.....
So, sit back and tighten your safety belt as we start our journey into the realm of data.
In this example, the cell population in each flask is measured in quadruplicate. This is a process whereby the precision of the final population value is improved by taking more than just one reading. It is important to note, however, that these are not independent readings as in each case the four samples are taken at the same time from the same flask. Consequently, the four values are averaged to provide a single value which is the best estimate of the population of cells in each flask, i.e. n = 1 for each flask. Such a process has often been erroneously misinterpreted as providing an n = 4, but this is incorrect simply because the four values for each flask are not independent. [See also Chapter 20, Example 20.3.]
.....