Evidence-Based Statistics
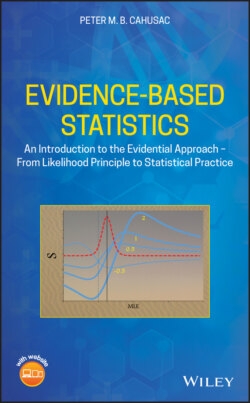
Реклама. ООО «ЛитРес», ИНН: 7719571260.
Оглавление
Peter M. B. Cahusac. Evidence-Based Statistics
Table of Contents
List of Tables
List of Illustrations
Guide
Pages
Evidence-Based Statistics. An Introduction to the Evidential Approach — from Likelihood Principle to Statistical Practice
Acknowledgements
About the Author
About the Companion Site
Introduction
References
1 The Evidence is the Evidence
1.1 Evidence-Based Statistics
1.1.1 The Literature
1.2 Statistical Inference – The Basics
1.2.1 Different Statistical Approaches
1.2.2 The Likelihood/Evidential Approach
1.2.3 Types of Approach Using Likelihoods
1.2.4 Pros and Cons of Likelihood Approach. Advantages:
Disadvantages:
1.3 Effect Size – True If Huge!
1.4 Calculations
1.5 Summary of the Evidential Approach
References
Notes
2 The Evidential Approach
2.1 Likelihood
2.1.1 The Principle
Definitions
2.1.2 Support
Using Logarithms
2.1.3 Example – One Sample
Getting Technical
2.1.4 Direction Matters
2.1.5 Maximum Likelihood Ratio
2.1.6 Likelihood Intervals
Summary of Example
2.1.7 The Support Function
2.1.8 Choosing the Effect Size
2.2 Misleading and Weak Evidence
2.3 Adding More Data and Multiple Testing
2.4 Sequence of Calculations Using t
2.5 Likelihood Terminology. Likelihood Terminology and Abbreviations
2.6 R Code for Chapter 2. 2.6.1 Calculating the Likelihood Function for a One Sample t
2.7 Exercises
References
Notes
3 Two Samples
3.1 Basics Using the t Distribution
3.1.1 Steps in Calculations
3.2 Related Samples
3.3 Independent Samples
3.3.1 Independent Samples with Unequal Variances
3.4 Calculation Simplification
3.5 If Variance Is Known, or Large Sample Size, Use z
3.6 Methodological and Pro Forma Analyses
3.7 Adding More Data
3.8 Estimating Sample Size
3.8.1 Sample Size for One Sample and Related Samples
3.8.2 Sample Size for Independent Samples
3.9 Differences in Variances
3.10 R Code For Chapter 3. 3.10.1 Calculating the Likelihood Function, the Likelihoods and Support for Independent Samples
3.10.2 Creating a Gardner–Altman Estimation Plot with Likelihood Function and Interval
3.11 Exercises
References
Notes
4 ANOVA
4.1 Multiple Means
4.1.1 The Modelling Approach
4.1.2 Model Complexity
4.2 Example – Fitness
4.2.1 Comparing Models
4.2.2 Specific Model Comparisons
4.2.2.1 A Non-Orthogonal Contrast
4.2.3 Unequal Sample Sizes
4.3 Factorial ANOVA
4.3.1 Example – Blood Clotting Times
4.3.2 Specific Analyses in Factorial ANOVA, Including Contrasts
4.4 Alerting r2
4.4.1 Alerting r2 to Compare Contrasts for Effect Size
4.5 Repeated Measures Designs
4.5.1 Mixed Repeated Measures with Between Participant Designs
4.5.2 Contrasts in Mixed Designs
4.6 Exercise
References
Notes
5 Correlation and Regression
5.1 Relationships Between Two Variables
5.2 Correlation
5.2.1 Likelihood Intervals for Correlation
5.3 Regression
The Akaike Information Criterion (AIC)
5.3.1 Obtaining Evidence from F values
5.3.2 Examining Non-linearity
5.4 Logistic Regression
5.5 Exercises
References
Notes
6 Categorical Data
6.1 Types of Categorical Data
6.1.1 How Is the χ2 Test Used?
6.2 Binomial
6.2.1 Likelihood Intervals for Binomial
Technique
6.2.2 Comparing Different π
6.2.3 The Support Function
6.3 Poisson
6.4 Rate Ratios
6.5 One-Way Categorical Data
6.5.1 One-Way Categorical Comparing Different Expected Values
6.5.2 One-Way with More than Two Categories
6.6 2 × 2 Contingency Tables
6.6.1 Paired 2 × 2 Categorical Analysis
6.6.2 Diagnostic Tests
6.6.2.1 Sensitivity and Specificity
6.6.2.2 Positive and Negative Predictive Values
6.6.2.3 Likelihood Ratio and Post-test Probability
6.6.2.4 Comparing Sensitivities and Specificities of Two Diagnostic Procedures
6.6.3 Odds Ratio
Odds and Probabilities
6.6.3.1 Likelihood Function for the Odds Ratio
6.6.4 Likelihood Function for Relative Risk with Fixed Entries
6.7 Larger Contingency Tables
6.7.1 Main Effects
6.7.2 Evidence for Linear Trend
6.7.3 Higher Dimensions?
6.8 Data That Fits a Hypothesis Too Well
6.9 Transformations of the Variable
6.10 Clinical Trials – A Tragedy in 3 Acts
6.11 R Code for Chapter 6
6.11.1 One-Way Categorical Data Support Against Specified Proportions
6.11.2 Calculating the Odds Ratio Likelihood Function and Support
6.11.3 Calculating the Likelihood Function and Support for Relative Risk with Fixed Entries
6.11.4 Calculating Interaction and Main Effects for Larger Contingency Tables
6.11.5 Log-Linear Modelling for Multi-way Tables
6.12 Exercises
References
Notes
7 Nonparametric Analyses
7.1 So-Called ‘Distribution-Free’ Statistics
7.2 Hacking SM
7.3 One Sample and Related Samples
7.4 Independent Samples
7.5 More than Two Independent Samples
7.6 Permutation Analyses
7.7 Bootstrap Analyses for One Sample or Related Samples
7.7.1 Bootstrap Analyses for Independent Samples
7.8 R Code for Chapter 7
7.8.1 Calculating Relative Support for One Sample
7.8.2 Calculating Relative Support for Differences in Two Independent Samples
7.8.3 Calculating Relative Support for Differences in Three Independent Samples
7.8.4 Calculating Relative Support Using Permutations Analysis
7.8.5 Bootstrap Analyses for One Sample
7.8.6 Bootstrap Analyses for Two Independent Samples
7.9 Exercises
References
8 Other Useful Techniques
8.1 Other Techniques
8.2 Critical Prior Interval
8.3 False Positive Risk
8.4 The Bayes Factor and the Probability of the Null Hypothesis
8.4.1 Example
8.5 Bayesian t Tests
8.6 The Armitage Stopping Rule
8.7 Counternull Effect Size
References
Notes
Appendix A Orthogonal Polynomials
Appendix B Occam's Bonus
Reference
Appendix C Problems with p Values
C.1 The Misuse of p Values
C.1.1 p Value Fallacies
C.2 The Use of p Values
C.2.1 Two Contradictory Traditions
C.2.2 Whither the p Value?
C.2.3 Remedies
References
Index
WILEY END USER LICENSE AGREEMENT
Отрывок из книги
Peter M. B. Cahusac
www.wiley.com/go/evidencebasedstatistics
.....
Estimation, a key element in statistical analysis, has often been ignored in the face of dichotomous decisions reached from statistical tests. If results are reported as non-significant, it is assumed that there is no effect or difference between population parameters. Alternatively, highly significant results based on large samples are assumed to represent large effects. The increased use of confidence intervals [26] is a great improvement that allows us to see how large or small the magnitude of the effects are, and hence whether they are of practical/clinical importance. These advances have increased the credibility of well-reported studies and facilitated our understanding of research results. The confidence interval is illustrated in the middle portion of Figure 1.1. This is centred on the sample mean (shown by the end-stopped line) and gives a range of plausible values for the population mean [26]. The interval has a frequentist interpretation: 95% of such intervals, calculated from random samples taken from the population of interest, will contain the population statistic. The confidence interval focusses our attention on the obtained sample mean value, and the 95% limits indicate how far this value is from parameter values of interest, especially the null. The interval helps us determine whether the data we have is of practical importance.
Figure 1.1 From sampling distribution to likelihood function. The top curve shows the sampling distribution used for testing statistical significance. It is centred on the null hypothesis value (often 0) and the standard error used to calculate the curve comes from the observed data. Below this in the middle is shown the 95% confidence interval. This uses the sample mean and standard error from the observed data. At the bottom shows the likelihood function, within which is plotted the S-2 likelihood function. Both the likelihood function and the likelihood interval use the observed data like the confidence interval.
.....