Human Motion Capture and Identification for Assistive Systems Design in Rehabilitation
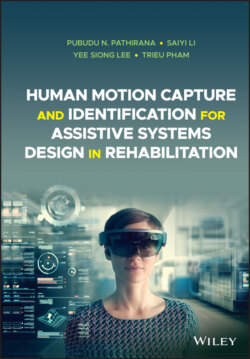
Реклама. ООО «ЛитРес», ИНН: 7719571260.
Оглавление
Pubudu N. Pathirana. Human Motion Capture and Identification for Assistive Systems Design in Rehabilitation
Table of Contents
List of Tables
List of Illustrations
Guide
Pages
Human Motion Capture and Identification for Assistive Systems Design in Rehabilitation
1. Introduction. 1.1 Human Body – Kinematic Perspective
1.2 Musculoskeletal Injuries and Neurological Movement Disorders
1.2.1 Musculoskeletal injuries
1.2.2 Neuromuscular disorders
Upper motor neurone disorders–
Lower motor neurone disorders – spinal muscular atrophies
Neuropathies
Neuromuscular junction disorders
1.3 Sensors in Telerehabilitation
1.3.1 Opto‐electronic sensing
1.3.2 RGB camera and microphone
1.3.3 Inertial measurement unit (IMU)
1.4 Model‐based State Estimation and Sensor Fusion
1.4.1 Summary and challenges
1.5 Human Motion Encoding in Telerehabilitation
1.5.1 Human motion encoders in action recognition
1.5.2 Human motion encoders in physical telerehabilitation
1.5.3 Summary and challenge
1.6 Patients' Performance Evaluation
1.6.1 Questionnaire‐based assessment scales
1.6.2 Automated kinematic performance assessment
1.6.3 Summary and challenge
2. Kinematic Performance Evaluation with Non‐wearable Sensors. 2.1 Introduction
2.2 Fusion. 2.2.1 Introduction
2.2.2 Linear model of human motion multi‐Kinect system
Rotation and translation of the Kinect©
2.2.3 Model‐based state estimation
2.2.4 Fusion of information
2.2.5 Mitigation of occlusions and optimised positioning
Occlusions and incomplete information
Kinect© optimal positioning
2.2.6 Computer simulations and hardware implementation
Computer simulations. Improvement due to fusion
System cost and complexity in applied tele‐rehabilitation
Hardware experiment. Improvement due to multiple Kinects©
Robustness due to occlusions
Discussion
Application in tele‐rehabilitation
2.3 Encoder. 2.3.1 Introduction
2.3.2 The two‐component encoder theory
2.3.3 Encoding methods
Temporal index
Spatial index
2.3.4 Dealing with noise
Least‐squares Gaussian filter
The Savitzky‐Golay filter
Kalman filter
2.3.5 Complex motion decomposition using switching continuous hidden Markov models
Building model for atomic motions
Complex motion decomposition
2.3.6 Canonical actions and the action alphabet
2.3.7 Experiments and results
Comparison between the Savitzky‐Golay filter and the least‐squares Gaussian filter
The computability of the two‐component model
Comparisons between the combinations of various indexing schemes and filtering approaches
Motion decomposition using switching continuous hidden Markov model (SCHMM)
2.4 ADL Kinematic Performance Evaluation. 2.4.1 Introduction
2.4.2 Methodology. Severity levels definition
Feature extraction
2.4.3 Experiment setup. Simulation data collection
Real‐data experiment data collection
2.4.4 Data analysis and results. Computer simulation data analysis
Computer simulation result
Healthy subjects simulation data analysis
Healthy subjects simulation result
Discussion and conclusion
2.5 Summary
3. Biokinematic Measurement with Wearable Sensors. 3.1 Introduction
3.2 Introduction to Quaternions
3.3 Wahba's Problem
3.3.1 Solutions to the Wahba problem. TRIAD
Singular value decomposition method
3.3.2 Davenport's q method
3.3.3 Quaternion Estimation Algorithm (QUEST)
Reference frame rotation in the QUEST method
3.3.4 Fast optimal attitude matrix (FOAM)
3.3.5 Estimator of the optimal quarternion (ESOQ or ESOQ1) method
3.4 Quaternion Propagation
3.5 MARG (Magnetic Angular Rates and Gravity) Sensor Arrays‐based Algorithm
3.6 Model‐based Estimation of Attitude with IMU Data
3.7 Robust Optimisation‐based Approach for Orientation Estimation
3.8 Implementation of the Orientation Estimation
3.8.1 Extended Kalman filter‐based approach
3.8.2 Robust extended Kalman filter implementation
3.8.3 Robust extended Kalman filter with linear measurements
3.9 Computer Simulations
3.10 Experimental Setup
3.11 Results and Discussion. 3.11.1 Computer simulations
3.11.2 Experiment
3.12 Conclusion
4. Capturing Finger Movements. 4.1 Introduction
4.2 System Overview
4.3 Accuracy Improvement of Total Active Movement and Proximal Interphalangeal Joint Angles
4.4 Simulation
4.5 Trial Procedure
4.6 Results. 4.6.1 Concurrence validity
4.6.2 Internal reliability
4.6.3 Time efficiency
4.7 Discussions
4.8 Approaching Finger Movement with a New Perspective
4.9 Reachable Space
4.10 Boundary of the Reachable Space
4.11 Area of the Reachable Space
4.12 Experiments
4.13 Results and Discussion
4.14 Conclusion and Future Work
5. Non‐contact Measurement of Respiratory Function via Doppler Radar. 5.1 Introduction
5.2 Fundamental Operation of Microwave Doppler Radar. 5.2.1 Velocity and frequency
5.2.2 Correction of I/Q amplitude and phase imbalance
5.3 Signal Processing Approach. 5.3.1 Respiration rate
Fast Fourier transform (FFT)
Continuous wavelet transform (CWT)
5.3.2 Extracting respiratory signatures
The Piecewise Linear Fitting Method
The Savitzky‐Golay Method and Fourier Filtering
Breathing signal decomposition
Identification of dynamic time warping
5.3.3 Low‐pass filtering (LPF)
5.3.4 Discrete wavelet transform
5.4 Common Data Acquisitions Setup
5.5 Capturing the Dynamics of Respiration
5.5.1 Normal breathing
5.5.2 Fast breathing
5.5.3 Slow inhalation–fast exhalation
5.5.4 Fast inhalation–slow exhalation
5.5.5 Capturing abnormal breathing patterns
5.5.6 Breathing component decomposition, analysis and classification
I:E ratio analysis
5.6 Capturing Special Breathing Patterns
5.6.1 Correlation of radar signal with spirometer in tidal volume estimations
5.6.2 Experiment setup
5.6.3 Results
Normal breathing
Central sleep apnoea
Ataxic breathing and Biot's breathing
Cheyne‐Stokes respiration
Cheyne‐Stokes variant
Dysrhythmic breathing
Kussmaul's breathing
5.6.4 Motion signature from Doppler radar
5.6.5 Measurement of volume in (inhalation) and volume out (exhalation)
5.7 Removal of Motion Artefacts from Doppler Radar‐based Respiratory Measurements
5.7.1 Experimental verification
5.7.2 Results and discussion
5.7.3 Summary
5.8 Separation of Doppler Radar‐based Respiratory Signatures
5.8.1 Respiration sensing using Doppler radar
5.8.2 Signal processing source separation (ICA)
5.8.3 Experiment protocol for real data sensing
5.8.4 Two simulated respiratory sources
5.8.5 Experiment involving real subjects
5.8.6 Separation of hand motion
5.8.7 Conclusion
6. Appendix. 6.1 Static Estimators. 6.1.1 Least‐squares estimation
Linear regression
Non‐linear regression
6.1.2 Maximum likelihood estimation
6.2 Model‐based Estimators
6.2.1 Kalman filter (KF)
6.3 Particle Filter
6.3.1 Robust filtering with linear measurements
Robustness of the estimation
6.3.2 Constrained optimisation
Bibliography
Index. a
b
c
d
e
f
g
h
i
j
k
l
m
n
o
p
q
r
s
t
v
w
x
WILEY END USER LICENSE AGREEMENT
Отрывок из книги
Pubudu N. Pathirana
Saiyi Li
.....
In light of the above, in 1998, the term “telerehabilitation” was “first raised” in a scientific article, attempting to overcome the shortage in conventional rehabilitation services [303]. Co‐existing with the opportunities for telerehabilitation, such as economy of scale, interactive and motivation, reduced healthcare costs, patients' privacy prevention and so on [57], a number of challenges can be seen from an engineering point of view as well. These challenges are also closely related to the aim of this book.
.....