Biological Language Model
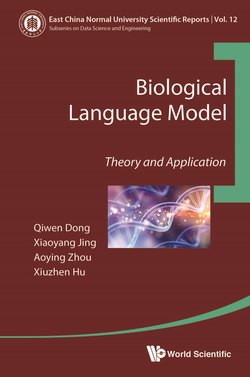
Реклама. ООО «ЛитРес», ИНН: 7719571260.
Оглавление
Qiwen Dong. Biological Language Model
Отрывок из книги
East China Normal University Scientific Reports
Subseries on Data Science and Engineering
.....
Amino acid encoding is the first step of protein structure and function prediction, and it is one of the foundations to achieve final success in those studies. In this chapter, we proposed the systematic classification of various amino acid encoding methods and reviewed the methods of each category. According to information sources and information extraction methodologies, these methods are grouped into five categories: binary encoding, physicochemical properties encoding, evolution-based encoding, structure-based encoding and machine-learning encoding. To benchmark and compare different amino acid encoding methods, we first selected 16 representative methods from those five categories. And then, based on the two representative protein-related studies, protein secondary structure prediction and protein fold recognition, we construct three machine learning models referring to the state-of-the-art studies. Finally, we encoded the protein sequence and implemented the same training and test phase on the benchmark datasets for each encoding method. The performance of each encoding method is regarded as the indicator of its potential in protein structure and function studies.
The assessment results show that the evolution-based position-dependent encoding method PSSM consistently achieves the best performance both on protein secondary structure prediction and protein fold recognition tasks, suggesting its important role in protein structure and function prediction. However, another evolution-based position-dependent encoding method — HMM — does not perform well, and the main reason for this could be that the remote homologous sequences only provide limited evaluation information for the target residue. For the one-hot encoding method, it is highly sparse and leads to complex machine learning models, while its two compressed representations, one-hot (6-bit) encoding and binary 5-bit encoding, lose more or less valuable information and cannot be widely used in related researches. More reasonable strategies to reduce the dimension of one-hot encoding need to be developed. For the physicochemical property encodings, the variety of properties and the extraction methodologies are two important factors needed to construct a valuable encoding. Structure-based encodings and machine-learning encodings achieve comparable or even better performances when compared with other widely used encodings, suggesting more attention needs to be paid to these two categories.
.....