Analysing Quantitative Data
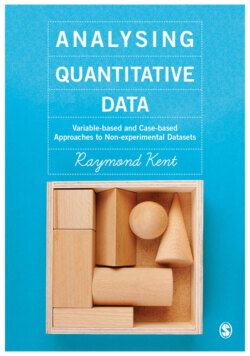
Реклама. ООО «ЛитРес», ИНН: 7719571260.
Оглавление
Raymond A Kent. Analysing Quantitative Data
Analysing Quantitative Data
Contents
List of Figures, Tables and Boxes. Figures
Tables
Boxes
About the Author
Companion Website
Preface
1 Data structure. Learning objectives
Introduction
Data and their construction
Key points and wider issues
The structure of quantitative data
Cases
Key points and wider issues
Properties
Key points and wider issues
Values
Key points and wider issues
Error in data construction
Box 1.1 The interpretation of Cronbach’s coefficient alpha
Key points and wider issues
The implications of this chapter for the alcohol marketing dataset
Chapter summary
Exercises and questions for discussion
Further reading
2 Data Preparation. Learning objectives
Introduction
Checking and editing
Coding
Assembling
Box 2.1 Entering data into IBM SPSS Statistics
Key points and wider issues
Transforming
Regrouping values
Box 2.2 Regrouping values in SPSS
Creating class intervals
Box 2.3 Creating class intervals in SPSS
Computing totals
Box 2.4 Computing totals in SPSS
Multiple response questions
Box 2.5 Multiple response items in SPSS
Upgrading or downgrading measures
Handling missing values and ‘Don’t know’ responses
Box 2.6 Missing values in SPSS
Open-ended questions
Key points and wider issues
Implications of this chapter for the alcohol marketing data
Chapter summary
Exercises and questions for discussion
Further reading
3 Approaches to data analysis. Learning objectives
Introduction
Datasets and data matrices
Key points and wider issues
Data analysis
Key points and wider issues
Ethical issues
Implications of this chapter for the alcohol marketing dataset
Chapter summary
Exercises and questions for discussion
Further reading
4 Univariate Analysis. Learning objectives
Introduction
Univariate data display: categorical variables
Frequency tables
Simple bar charts and pie charts
Univariate data display: metric variables
Frequency tables
Metric tables
Histograms and line graphs
Box 4.1 Producing frequency tables, charts and histograms using SPSS
Key points and wider issues
Data summaries: categorical variables
Data summaries: metric variables
Central tendency
Dispersion
Distribution shape
Key points and wider issues
Box 4.2 Data summaries using SPSS
Statistical inference for univariate hypotheses
Estimation
Confidence intervals for metric variables
Confidence intervals for categorical variables
Key points and wider issues
Testing hypotheses for statistical significance
Testing hypotheses for metric variables
Testing hypotheses for categorical variables
Key points and wider issues
Box 4.3 Statistical inference using SPSS
Other statistics and survey analysis packages
Implications of this chapter for the alcohol marketing dataset
Chapter summary
Exercises and questions for discussion
Further reading
5 Bivariate analysis. Learning objectives
Introduction
The variety of relationships between two variables
Key points and wider issues
Bivariate data display
Box 5.1 Using SPSS to produce crosstabulations
Key points and wider issues
Bivariate data summaries: categorical variables
Differences
Category clustering
Covariation
Box 5.2 Using SPSS to obtain measures of association for categorical variables
Key points and wider issues
Bivariate data summaries: metric variables
Box 5.3 Correlation and regression on SPSS
Key points and wider issues
Testing bivariate hypotheses
Testing bivariate hypotheses for categorical variables
Testing bivariate hypotheses for metric variables
Testing metric differences for categories
Box 5.4 Using SPSS to test for statistical significance
Key points and wider issues
Statistical inference and bivariate data summaries
Key points and wider issues
Implications of this chapter for the alcohol marketing dataset
Chapter summary
Exercises and questions for discussion
Further reading
6 Multivariate Analysis. Learning objectives
Introduction
The limitations of bivariate analysis
Key points and wider issues
What is multivariate analysis?
Multivariate analysis for categorical variables
Three-way and n-way tables
Box 6.1 Three-way analyses using SPSS
Log-linear analysis
Box 6.2 Log-linear analysis in SPSS
Key points and wider issues
Multivariate analysis for metric variables
Dependence techniques
Multiple regression
Box 6.3 Multiple regression using SPSS
Key points and wider issues
Logistic regression
Box 6.4 Logistic regression using SPSS
Key points and wider issues
Multivariate analysis of variance
Box 6.5 Multivariate analysis of variance using SPSS
Key points and wider issues
Multivariate analysis for metric variables: interdependence techniques
Factor analysis
Box 6.6 Factor analysis using SPSS
Key points and wider issues
Other interdependence techniques
Implications of this chapter for the alcohol marketing dataset
Chapter summary
Exercises and questions for discussion
Further reading
7 Set-Theoretic Methods and Configurational Data Analysis. Learning objectives
Introduction
The assessment of set membership
Set membership assessment for the alcohol marketing study
Key points and wider issues
Set relationships
Key points and wider issues
Configurational data analysis
The fuzzy set analysis software
Box 7.1 Using fsQCA: entering data
The truth table
Box 7.2 Avoiding fuzzy set values of [0.5]
Box 7.3 Using fsQCA: constructing a truth table
Box 7.4 Using fsQCA: obtaining the results
The analysis of logical necessity
Box 7.5 The analysis of necessary conditions in fsQCA
Key points and wider issues
Pitfalls in the analysis and interpretation of fsQCA findings
Skewed set memberships
Lack of diversity
Box 7.6 Counterfactual analysis
Contradiction
Requirements and limitations
Standards of good practice
Key points and wider issues
Fuzzy set analysis and time
Other fuzzy set analysis software
Implications of this chapter for the alcohol marketing dataset
Chapter summary
Exercises and questions for discussion
Further reading
Notes
8 Cluster and Discriminant Analysis. Learning objectives
Introduction
Approaches to cluster analysis
Cluster analysis limitations
Cluster analysis and the alcohol marketing dataset
Box 8.1 Cluster analysis using SPSS
Key points and wider issues
Cluster analysis and fuzzy set analysis
Discriminant analysis
Discriminant analysis and the alcohol marketing dataset
Box 8.2 Discriminant analysis using SPSS
Key points and wider issues
Implications of this chapter for the alcohol marketing dataset
Chapter summary
Exercises and questions for discussion
Further reading
9 Comparing and Mixing Methods. Learning objectives
Introduction
What variable-based analyses are good at Data reduction
Making comparisons
Showing covariation
Establishing the relative importance of independent variables
Exploration and verification
The limitations of variable-based analyses. Many cases are needed
Cases become invisible
Asymmetrical patterns are ignored
Regression is linear and additive
Limited diversity gets overlooked
Too much focus on statistical inference
Not good at establishing causality
What case-based analyses are good at. Keeping the focus on cases
Handling small-n research
Facilitating causal analysis
Controlling counterfactual assumptions
Producing recipes for achieving a desired outcome
The limitations of case-based analyses. CDA can be used only when a number of conditions are met
Solutions are very sensitive to the decisions and assumptions made by the researcher
CDA is not well suited to either exploration or verification
There are dangers of triviality, irrelevance or contradiction
Key points and wider issues
Mixed methods
Mixed data
Key points and wider issues
Implications of this chapter for the alcohol marketing dataset
Chapter summary
Exercises and questions for discussion
Further reading
10 Evaluating Hypotheses, Explaining and Communicating Results. Learning objectives
Introduction
Evaluating hypotheses
Key points and wider issues
Establishing causality
Key points and wider issues
Explaining findings
Experimental rhetoric
Statistical rhetoric
Quantitative case-based rhetoric
Qualitative case-based rhetoric
Key points and wider issues
Presenting results
Key points and wider issues
Implications of this chapter for the alcohol marketing dataset
Chapter summary
Exercises and questions for discussion
Further reading
Answers to Exercises and Questions for Discussion. Chapter 1. Exercise 1
Exercise 2
Exercise 3
Exercise 4
Exercise 5
Exercise 6
Chapter 2. Exercise 1
Exercise 2
Exercise 3
Exercise 4
Exercise 5
Exercise 6
Chapter 3. Exercise 1
Exercise 2
Exercise 3
Chapter 4. Exercise 1
Exercise 2
Exercise 3
Exercise 4
Exercise 5
Chapter 5. Exercise 1
Exercise 2
Exercise 3
Exercise 4
Chapter 6. Exercise 1
Exercise 2
Exercise 3
Exercise 4
Chapter 7. Exercise 1
Exercise 2
Exercise 3
Exercise 4
Exercise 5
Exercise 6
Exercise 7
Exercise 8
Chapter 8. Exercise 1
Exercise 2
Exercise 3
Exercise 4
Exercise 5
Chapter 9. Exercise 1
Exercise 2
Exercise 3
Chapter 10. Exercise 1
Exercise 2
Exercise 3
Glossary
References
Index
Отрывок из книги
Variable-based and Case-based Approaches to Non-experimental Datasets
In addition, there is:
.....
Nominal variables are sometimes converted into binary variables so that, for example, the dichotomy A/B becomes A/not A and B/not B. A trichotomy becomes A/not A, B/not B and C/not C. Statisticians sometimes call these dummy variables and they are useful because they have particular properties and can be used in some statistical procedures where nominal variables are inappropriate.
A key feature of nominal variables is that where there are three or more categories, the order in which the values appear in a table makes no difference to any statistical calculations that may appropriately be applied to the data. The values do need to be listed in some sequence (which might, for example, be alphabetical), but it is not a graduated series from ‘high’ to ‘low’ or ‘large’ to ‘small’. Some variables, however, define the relationships between values not just in terms of categories that are exhaustive and mutually exclusive, but the categories are also arranged in relationships of greater than or less than, although there is no metric that will indicate by how much. Thus product usage can be classified into ‘Heavy’, ‘Medium’, ‘Light’ and ‘Non-user’; there is an implied order, but no measure of the actual usage involved. The various social classes, social grades or socio-economic groups used in various European countries are good examples of such ordered category variables. The individual items used to generate summated rating scales such as the Likert scale, which were explained in the previous section, are also common examples of ordered categories.
.....