Applied Biostatistics for the Health Sciences
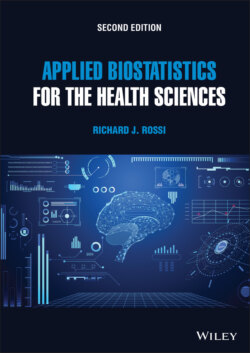
Реклама. ООО «ЛитРес», ИНН: 7719571260.
Оглавление
Richard J. Rossi. Applied Biostatistics for the Health Sciences
APPLIED BIOSTATISTICS FOR THE HEALTH SCIENCES
Contents
List of Illustrations
List of Tables
Guide
Pages
PREFACE
Topic Coverage
Special Features
Pedagogical Features
Teaching from this Book
Website
Acknowledgments
CHAPTER 1INTRODUCTION TO BIOSTATISTICS
1.1 What is Biostatistics?
1.2 Populations, Samples, and Statistics
1.2.1 The Basic Biostatistical Terminology
Example 1.1
Example 1.2
1.2.2 Biomedical Studies
Example 1.3
Example 1.4
Example 1.5
Example 1.6
Example 1.7
1.2.3 Observational Studies Versus Experiments
Example 1.8
Example 1.9
Example 1.10
1.3 Clinical Trials
1.3.1 Safety and Ethical Considerations in a Clinical Trial
1.3.2 Types of Clinical Trials
1.3.3 The Phases of a Clinical Trial
1.4 Data Set Descriptions
1.4.1 Birth Weight Data Set
1.4.2 Body Fat Data Set
1.4.3 Coronary Heart Disease Data Set
1.4.4 Prostate Cancer Study Data Set
1.4.5 Intensive Care Unit Data Set
1.4.6 Mammography Experience Study Data Set
1.4.7 Benign Breast Disease Study
1.4.8 Exerbike Data Sets
Glossary
Exercises
CHAPTER 2DESCRIBING POPULATIONS
2.1 Populations and Variables
2.1.1 Qualitative Variables
2.1.2 Quantitative Variables
2.1.3 Multivariate Data
2.2 Population Distributions and Parameters
2.2.1 Distributions
2.2.2 Describing a Population with Parameters
2.2.3 Proportions and Percentiles
2.2.4 Parameters Measuring Centrality
2.2.5 Measures of Dispersion
THE EMPIRICAL RULES
2.2.6 The Coefficient of Variation
2.2.7 Parameters for Bivariate Populations
2.3 Probability
THE AXIOMS OF PROBABILITY
2.3.1 Basic Probability Rules
2.3.2 Conditional Probability
2.3.3 Independence
INDEPENDENT EVENTS
2.3.4 The Relative Risk and the Odds Ratio
2.4 Probability Models
2.4.1 The Binomial Probability Model
THE BINOMIAL CONDITIONS
2.4.2 The Normal Probability Model
PROPERTIES OF A NORMAL DISTRIBUTION
COMPUTING STANDARD NORMAL PROBABILITIES
THE RELATIONSHIPS BETWEEN A STANDARD NORMAL AND A NON-STANDARD NORMAL
NON-STANDARD NORMAL PROBABILITIES
2.4.3 Z Scores
Glossary
Exercises
CHAPTER 3RANDOM SAMPLING
3.1 Obtaining Representative Data
3.1.1 The Sampling Plan
DESIGNING THE SAMPLING PLAN
3.1.2 Probability Samples
THE PROCEDURE FOR DRAWING A RANDOM SAMPLE
PROPERTIES OF A RANDOM SAMPLE
3.2 Commonly Used Sampling Plans
3.2.1 Simple Random Sampling
THE SIMPLE RANDOM SAMPLING PROCEDURE
PROPERTIES OF A SIMPLE RANDOM SAMPLE
3.2.2 Stratified Random Sampling
THE STRATIFIED RANDOM SAMPLING PROCEDURE
PROPERTIES OF A STRATIFIED RANDOM SAMPLE
3.2.3 Cluster Sampling
THE CLUSTER SAMPLING PROCEDURE
PROPERTIES OF A RANDOM CLUSTER SAMPLE
3.2.4 Systematic Sampling
THE SYSTEMATIC SAMPLE PROCEDURE
3.3 Determining the Sample Size
3.3.1 The Sample Size for Simple and Systematic Random Samples
3.3.2 The Sample Size for a Stratified Random Sample
Glossary
Exercises
CHAPTER 4SUMMARIZING RANDOM SAMPLES
4.1 Samples and Inferential Statistics
THE COMPONENTS OF A STATISTICAL ANALYSIS
4.2 Inferential Graphical Statistics
4.2.1 Bar and Pie Charts
Example 4.1
4.2.2 Boxplots
CONSTRUCTING A SIMPLE BOXPLOT
Example 4.2
Example 4.3
CONSTRUCTING AN OUTLIER BOXPLOT
Example 4.4
A Procedure for Dealing with Outliers
Example 4.5
Example 4.6
Example 4.7
4.2.3 Histograms
CONSTRUCTING A RELATIVE FREQUENCY HISTOGRAM
Example 4.8
DENSITY HISTOGRAM
Example 4.9
Example 4.10
SOME COMMENTS ON USING HISTOGRAMS
Example 4.11
4.2.4 Normal Probability Plots
THE FAT PENCIL TEST
Example 4.12
4.3 Numerical Statistics for Univariate Data Sets
4.3.1 Estimating Population Proportions
ESTIMATING A PROPORTION
Example 4.13
Example 4.14
Example 4.15
Example 4.16
Example 4.17
Example 4.18
Example 4.19
4.3.2 Estimating Population Percentiles
ESTIMATING A PERCENTILE
Example 4.20
4.3.3 Estimating the Mean, Median, and Mode
PARAMETERS OF CENTRALITY AND DISTRIBUTIONAL SHAPES
ESTIMATING A MODE
Example 4.21
ESTIMATING THE MEAN
Example 4.22
Example 4.23
ESTIMATING THE MEDIAN
Example 4.24
Example 4.25
4.3.4 Estimating the Variance and Standard Deviation
ESTIMATING THE VARIANCE AND STANDARD DEVIATION
BoxComputing the Value of s2
Example 4.26
COMPUTATIONAL FORMULAS FOR s2
Example 4.27
ESTIMATING THE INTERQUARTILE RANGE
Example 4.28
4.3.5 Linear Transformations
Example 4.29
LINEAR TRANSFORMATIONS OF PARAMETERS
LINEAR TRANSFORMATIONS OF THE ESTIMATED PARAMETERS
Example 4.30
Example 4.31
4.3.6 The Plug-in Rule for Estimation
Example 4.32
4.4 Statistics for Multivariate Data Sets
Example 4.33
4.4.1 Graphical Statistics for Bivariate Data Sets
4.4.2 Numerical Summaries for Bivariate Data Sets
THE POPULATION CORRELATION COEFFICIENT
FACTS ABOUT THE CORRELATION COEFFICIENT
FACTS ABOUT THE SAMPLE CORRELATION COEFFICIENT
BoxComputing the Sample Correlation Coefficient
INVESTIGATING PAIRWISE RELATIONSHIPS IN A MULTIVARIATE DATA SET
Example 4.34
4.4.3 Fitting Lines to Scatterplots
THE LEAST SQUARES REGRESSION LINE
Example 4.35
Glossary
Exercises
CHAPTER 5MEASURING THE RELIABILITY OF STATISTICS
5.1 Sampling Distributions
PROPERTIES OF SIMPLE RANDOM SAMPLES
Example 5.1
5.1.1 Unbiased Estimators
Example 5.2
UNBIASED ESTIMATORS
5.1.2 Measuring the Accuracy of an Estimator
Example 5.3
5.1.3 The Bound on the Error of Estimation
THE EMPIRICAL RULES FOR AN UNBIASED ESTIMATOR
Example 5.4
Example 5.5
5.2 The Sampling Distribution of a Sample Proportion
5.2.1 The Mean and Standard Deviation of the Sampling Distribution of p^
Example 5.6
Example 5.7
5.2.2 Determining the Sample Size for a Prespecified Value of the Bound on the Error Estimation
THE SAMPLE SIZE REQUIRED FOR ESTIMATING A PROPORTION
Example 5.8
Example 5.9
5.2.3 The Central Limit Theorem for p^
CENTRAL LIMIT THEOREM (CLT) FOR p^
Example 5.10
5.2.4 Some Final Notes on the Sampling Distribution of p^
Properties of p^
5.3 The Sampling Distribution of x¯
5.3.1 The Mean and Standard Deviation of the Sampling Distribution of x¯
Example 5.11
Example 5.12
Example 5.13
5.3.2 Determining the Sample Size for a Prespecified Value of the Bound on the Error Estimation
THE SAMPLE SIZE REQUIRED FOR ESTIMATING A MEAN
Example 5.14
5.3.3 The Central Limit Theorem for x¯
THE CENTRAL LIMIT THEOREM FOR x¯
Example 5.15
EMPIRICAL RULES FOR THE SAMPLING DISTRIBUTION OF x¯
5.3.4 The t Distribution
THE t DISTRIBUTION
Example 5.16
5.3.5 Some Final Notes on the Sampling Distribution of x¯
THE KEY PROPERTIES OF x¯
5.4 Two Sample Comparisons
5.4.1 Comparing Two Population Proportions
Example 5.17
CASE 1: MINIMIZING THE VALUE OF B FOR A FIXED SAMPLE SIZE
Example 5.18
CASE 2: MINIMIZING THE SAMPLE SIZE FOR A FIXED VALUE OF B
Example 5.19
Case 3: Minimizing The Value of B for a Fixed Cost
Example 5.20
CASE 4: MINIMIZING THE COST FOR A FIXED VALUE OF B
Example 5.21
5.4.2 Comparing Two Population Means
Example 5.22
CASE 1: MINIMIZING B WHEN N IS FIXED
CASE 2: MINIMIZING N WHEN B IS FIXED
Example 5.23
CASE 3: MINIMIZING B WHEN THE COST IS FIXED
Example 5.24
CASE 4: MINIMIZING THE COST WHEN B IS FIXED
Example 5.25
5.5 Bootstrapping the Sampling Distribution of a Statistic
THE BOOSTRAP ALGORITHM FOR SE (T)
Example 5.26
BOOSTRAPPING SE (TX−TY)
Example 5.27
Glossary
Exercises
CHAPTER 6CONFIDENCE INTERVALS. 6.1 Interval Estimation
AN INTERVAL ESTIMATOR BASED ON B
Example 6.1
LARGE SAMPLE INTERVAL ESTIMATORS BASED ON B
Example 6.2
6.2 Confidence Intervals
CONFIDENCE INTERVAL BOX MODEL
Example 6.3
6.3 Single Sample Confidence Intervals
6.3.1 Confidence Intervals for Proportions
LARGE SAMPLE CONFIDENCE INTERVALS FOR P
Example 6.4
LARGE SAMPLE ONE-SIDED CONFIDENCE INTERVALS FOR P
Example 6.5
DETERMINING N FOR A CONFIDENCE INTERVAL FOR A PROPORTION
Example 6.6
6.3.2 Confidence Intervals for a Mean
6.3.3 Large Sample Confidence Intervals for µ
LARGE SAMPLE CONFIDENCE INTERVALS FOR A MEAN
Example 6.7
6.3.4 Small Sample Confidence Intervals for µ
SMALL SAMPLE CONFIDENCE INTERVALS FOR A MEAN
Example 6.8
Example 6.9
6.3.5 DETERMINING THE SAMPLE SIZE FOR A CONFIDENCE INTERVAL FOR THE MEAN
Determining The Sample Size for a Confidence Interval µ
Example 6.10
6.4 Bootstrap Confidence Intervals
THE BOOTSTRAP CONFIDENCE INTERVAL PROCEDURE
Example 6.11
6.5 Two Sample Comparative Confidence Intervals
6.5.1 Confidence Intervals for Comparing Two Proportions
LARGE SAMPLE CONFIDENCE INTERVALS FOR pX−pY
Example 6.12
LARGE SAMPLE ONE-SIDED CONFIDENCE INTERVALS FOR pX−pY
Example 6.13
DETERMINING THE SAMPLE SIZE FOR A CONFIDENCE INTERVAL FOR pX−pY
Example 6.14
6.5.2 Confidence Intervals for the Relative Risk
A LARGE SAMPLE CONFIDENCE INTERVAL FOR THE RELATIVE RISK
Example 6.15
6.5.3 Confidence Intervals for the Odds Ratio
A LARGE SAMPLE CONFIDENCE INTERVAL FOR THE ODDS RATIO
Example 6.16
Glossary
Exercises
CHAPTER 7TESTING STATISTICAL HYPOTHESES. 7.1 Hypothesis Testing
Example 7.1
7.1.1 The Components of a Hypothesis Test
Example 7.2
HYPOTHESIS TESTS FOR θ
Example 7.3
Example 7.4
TESTING ERRORS
Example 7.5
Example 7.6
7.1.2 P-Values and Significance Testing
P-VALUES
Example 7.7
DECISION RULES BASED ON P-VALUES
Example 7.8
GENERAL GUIDELINES FOR INTERPRETING P-VALUES
7.2 Testing Hypotheses about Proportions
7.2.1 Single Sample Tests of a Population Proportion
HYPOTHESIS TESTS FOR PROPORTIONS
Example 7.9
P-VALUES FOR THE LARGE SAMPLE Z-TEST
Example 7.10
Example 7.11
Example 7.12
7.2.2 Comparing Two Population Proportions
HYPOTHESIS TESTS FOR COMPARING TWO PROPORTIONS
P-VALUES FOR A Z-TEST
7.2.3 Tests of Independence
REJECTION REGION FOR A TEST OF INDEPENDENCE FOR DICHOTOMOUS VARIABLES
REJECTION REGION FOR A TEST OF INDEPENDENCE OF POLYTOMOUS VARIABLES
7.3 Testing Hypotheses About Means
7.3.1 t-Tests
P-VALUES FOR A T-TEST
7.3.2 t-Tests for the Mean of a Population
7.3.3 Paired Comparison t-Tests
7.3.4 Two Independent Sample t-Tests
THE TWO SAMPLE t STATISTIC
7.4 Some Final Comments on Hypothesis Testing
Glossary
Exercises
CHAPTER 8SIMPLE LINEAR REGRESSION
8.1 Bivariate Data, Scatterplots, and Correlation
8.1.1 Scatterplots
8.1.2 Correlation
8.2 The Simple Linear Regression Model
THE SIMPLE LINEAR REGRESSION PROCEDURE
8.2.1 The Simple Linear Regression Model
8.2.2 Assumptions of the Simple Linear Regression Model
ASSUMPTIONS FOR A SIMPLE LINEAR REGRESSION MODEL
THE DISTRIBUTION OF Y = β0 + β1X + ϵ
8.3 Fitting a Simple Linear Regression Model
8.4 Assessing the Assumptions and Fit of a Simple Linear Regression Model
8.4.1 Residuals
8.4.2 Residual Diagnostics
8.4.3 Estimating σ and Assessing the Strength of the Linear Relationship
8.5 Statistical Inferences based on a Fitted Model
8.5.1 Inferences About β0
8.5.2 Inferences About β1
8.6 Inferences about the Response Variable
8.6.1 Inferences About μY|X
8.6.2 Inferences for Predicting Values of Y
8.7 Model Validation
8.7.1 Selecting the Training and Validation Data Sets
8.7.2 Validating a Fitted Model
8.8 Some Final Comments on Simple Linear Regression
SIMPLE LINEAR REGRESSION ANALYSIS
Glossary
Exercises
CHAPTER 9MULTIPLE REGRESSION
THE MULTIPLE REGRESSION PROCEDURE
9.1 Investigating Multivariate Relationships
9.2 The Multiple Linear Regression Model
THE BASIC COMPONENTS OF A MULTIPLE REGRESSION MODEL
9.2.1 The Assumptions of a Multiple Regression Model
THE ASSUMPTIONS FOR A MULTIPLE LINEAR REGRESSION MODEL
9.3 Fitting a Multiple Linear Regression Model
9.4 Assessing the Assumptions of a Multiple Linear Regression Model
IDENTIFYING AND CURING A COLLINEARITY PROBLEM
9.4.1 Residual Diagnostics
MODELING CURVILINEAR RELATIONSHIPS
9.4.2 Detecting Multivariate Outliers and Influential Observations
9.5 Assessing the Adequacy of Fit of a Multiple Regression Model
9.5.1 Estimating σ
9.5.2 The Coefficient of Determination
9.5.3 Multiple Regression Analysis of Variance
MULTIPLE REGRESSION SUM OF SQUARES
9.6 Statistical Inferences-Based Multiple Regression Model
9.6.1 Inferences about the Regression Coefficients
9.6.2 Inferences About the Response Variable
9.7 Comparing Multiple Regression Models
THE EXTRA SUM OF SQUARES F-TEST
9.8 Multiple Regression Models with Categorical Variables
9.8.1 Regression Models with Dummy Variables
9.8.2 Testing the Importance of Categorical Variables
COMPARING DUMMY VARIABLE MODELS
9.9 Variable Selection Techniques
THE GENERAL VARIABLE SELECTION PROCEDURE
9.9.1 Model Selection Using Maximum Radj2
THE MAXIMUM Radj2 VARIABLE SELECTION PROCEDURE
9.9.2 Model Selection using BIC
THE BIC VARIABLE SELECTION PROCEDURE
9.10 Model Validation
9.10.1 Selecting the Training and Validation Data Sets
9.10.2 Validating a Fitted Model
9.11 Some Final Comments on Multiple Regression
THE MULTIPLE REGRESSION PROCEDURE
Glossary
Exercises
CHAPTER 10LOGISTIC REGRESSION
10.1 The Logistic Regression Model
Example 10.2
Example 10.3
10.1.1 Assumptions of the Logistic Regression Model
ASSUMPTIONS OF A LOGISTIC REGRESSION MODEL
10.2 Fitting a Logistic Regression Model
Example 10.4
10.3 Assessing the Fit of a Logistic Regression Model
10.3.1 Checking the Assumptions of a Logistic Regression Model
Detecting Collinearities in Logistic Regression
Example 10.5
10.3.2 Testing for the Goodness of Fit of a Logistic Regression Model
Example 10.6
Example 10.7
10.3.3 Model Diagnostics
Example 10.8
Example 10.9
Example 10.10
Example 10.11
Example 10.12
10.4 Statistical Inferences Based on a Logistic Regression Model
10.4.1 Inferences about the Logistic Regression Coefficients
Example 10.13
Example 10.14
10.4.2 Comparing Models
BoxDrop-in-Deviance Test
Example 10.15
Example 10.16
10.5 Variable Selection
VARIABLE SELECTION IN LOGISTIC REGRESSION
THE BIC VARIABLE SELECTION PROCEDURE FOR LOGISTIC REGRESSION
Example 10.17
10.6 Classification with Logistic Regression
10.6.1 The Logistic Classifier
THE LOGISTIC REGRESSION CLASSIFIER
Example 10.18
10.6.2 Misclassification Errors
Example 10.19
10.7 Some Final Comments on Logistic Regression
THE LOGISTIC REGRESSION PROCEDURE
Glossary
Exercises
CHAPTER 11DESIGN OF EXPERIMENTS
11.1 Experiments Versus Observational Studies
Example 11.1
Example 11.2
Example 11.3
EXPERIMENTS VERSUS OBSERVATION STUDIES
11.2 The Basic Principles of Experimental Design
11.2.1 Terminology
Example 11.4
11.2.2 Designing an Experiment
ADVANTAGES OF RANDOMIZATION
11.3 Experimental Designs
Example 11.5
Example 11.6
11.3.1 The Completely Randomized Design
CRD RANDOMIZATION METHOD I
CRD RANDOMIZATION METHOD II
Example 11.7
Example 11.8
Example 11.9
11.3.2 The Randomized Block Design
RBD RANDOMIZATION
Example 11.10
Example 11.11
11.4 Factorial Experiments
Example 11.12
Example 11.13
Example 11.14
11.4.1 Two-Factor Experiments
Example 11.15
Example 11.16
11.4.2 Three-Factor Experiments
Example 11.17
Example 11.18
11.5 Models for Designed Experiments
11.5.1 The Model for a Completely Randomized Design
THE LINEAR MODEL FOR A COMPLETELY RANDOMIZED DESIGN
Example 11.19
11.5.2 The Model for a Randomized Block Design
THE LINEAR MODEL FOR A RANDOMIZED BLOCK DESIGN
Example 11.20
11.5.3 Models for Experimental Designs with a Factorial Treatment Structure
THE LINEAR MODEL FOR A TWO-FACTOR TREATMENT STRUCTURE
THE LINEAR MODEL FOR A THREE-FACTOR TREATMENT STRUCTURE
11.6 Some Final Comments of Designed Experiments
Glossary
Exercises
CHAPTER 12ANALYSIS OF VARIANCE
12.1 Single-Factor Analysis of Variance
ASSUMPTIONS OF THE SINGLE-FACTOR LINEAR MODEL
12.1.1 Partitioning the Total Experimental Variation
12.1.2 The Model Assumptions
Example 12.2
Example 12.3
12.1.3 The F-test
Example 12.4
12.1.4 Comparing Treatment Means
Example 12.5
HAND CALCULATIONS FOR THE BONFERRONI PROCEDURE
Example 12.6
Example 12.7
12.2 Randomized Block Analysis of Variance
12.2.1 The ANOV Table for the Randomized Block Design
SS’S IN A RANDOMIZED BLOCK ANALYSIS OF VARIANCE
12.2.2 The Model Assumptions
Example 12.8
12.2.3 The F-test
Example 12.9
12.2.4 Separating the Treatment Means
Example 12.10
Example 12.11
Example 12.12
12.3 Multi factor Analysis of Variance
12.3.1 Two-Factor Analysis of Variance
THE TESTING PROCEDURE IN A TWO-FACTOR ANOV
Example 12.13
THE BONFERRONI CRITICAL DISTANCE D
Example 12.14
Example 12.15
12.3.2 Three-Factor Analysis of Variance
THE TESTING PROCEDURE IN A THREE-FACTOR ANOV
Example 12.16
12.4 Selecting the Number of Replicates in Analysis of Variance
12.4.1 Determining the Number of Replicates from the Power
Example 12.17
Example 12.18
12.4.2 Determining the Number of Replicates from D
Example 12.19
12.5 Some Final Comments on Analysis of Variance
Glossary
Exercises
CHAPTER 13SURVIVAL ANALYSIS
13.1 The Kaplan–Meier Estimate of the Survival Function
Example 13.1
Example 13.2
13.2 The Proportional Hazards Model
THE ASSUMPTIONS OF A PROPORTIONAL HAZARDS MODEL
Example 13.3
Example 13.4
13.3 Logistic Regression and Survival Analysis
Example 13.5
13.4 Some Final Comments on Survival Analysis
Glossary
Exercises
REFERENCES
APPENDIX A
Problem Solutions. Solutions for Chapter 1
Solutions for Chapter 2
Solutions for Chapter 3
Solutions for Chapter 4
Solutions for Chapter 5
Chapter 6 – Solutions
Solutions for Chapter 7
Solutions for Chapter 8
Solutions for Chapter 9
Solutions for Chapter 10
Solutions for Chapter 11
Solutions for Chapter 12
Solutions for Chapter 13
INDEX
WILEY END USER LICENSE AGREEMENT
Отрывок из книги
Second Edition
RICHARD J. ROSSI
.....
A probability is a number between 0 and 1 that measures how likely it is for an event to occur. Probabilities are associated with tasks or experiment where the outcome cannot be determined without actually carrying out the task. A task where the outcome cannot be predetermined is called a random experiment or a chance experiment. For example, prior to treatment it cannot be determined whether chemotherapy will improve a cancer patient’s health. Thus, the result of a chemotherapy treatment can be treated as a chance experiment before chemotherapy is started. Similarly, when drawing a random sample from the target population, the actual values of the sample will not be known until the sample is actually collected. Hence, drawing a random sample from the target population is a chance experiment.
Because statistical inferences are based on a sample from the population rather than a census of the population, the statistical inferences will have a degree of uncertainty associated with them. The measures of reliability for statistical inferences drawn from a sample are based on the underlying probabilities associated with the target population.
.....