Probability and Statistical Inference
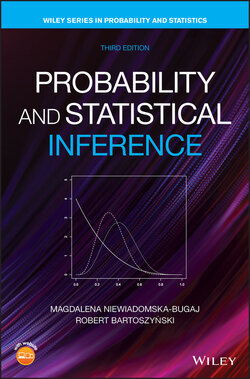
Реклама. ООО «ЛитРес», ИНН: 7719571260.
Оглавление
Robert Bartoszynski. Probability and Statistical Inference
Table of Contents
List of Tables
List of Illustrations
Guide
Pages
Wiley Series in Probability and Statistics
Probability and Statistical Inference
Preface to Third Edition
Preface to Second Edition
About the Companion Website
Chapter 1 Experiments, Sample Spaces, and Events. 1.1 Introduction
1.2 Sample Space
Example 1.1
Example 1.2
Example 1.3
Example 1.4
Example 1.5
Example 1.6
Example 1.7
Example 1.8 Selection
Example 1.9 Randomized Response
Problems
1.3 Algebra of Events
Example 1.10
Example 1.11 *2
Example 1.12
Example 1.13
Example 1.14
Example 1.15
Problems
1.4 Infinite Operations on Events
Example 1.16
Example 1.17
Example 1.18
Example 1.19
Example 1.20
Example 1.21
Example 1.22
Problems
Notes
Chapter 2 Probability
2.1 Introduction
2.2 Probability as a Frequency
2.3 Axioms of Probability
Example 2.1 Geometric Probability
Example 2.2 Bertrand's Paradox
Solution 1
Solution 2
Solution 3
Example 2.3
Problems
2.4 Consequences of the Axioms
Example 2.5
Solution
Problems
2.5 Classical Probability
Example 2.6
Solution
Problems
2.6 Necessity of the Axioms*
Example 2.7 Densities
2.7 Subjective Probability*
Problems
Note
Chapter 3 Counting. 3.1 Introduction
3.2 Product Sets, Orderings, and Permutations
Example 3.1 Cartesian Products
Example 3.2
Example 3.3 License Plates
Solution
Example 3.4
Solution
Example 3.5 Birthday Problem
Solution
Problems
3.3 Binomial Coefficients
Example 3.6
Corollary 3.3.6
Example 3.7
Solution
Example 3.8
Example 3.9
Example 3.10
Solution
Example 3.11 Matching Problem
Solution
Example 3.12 Ballot Problem
Solution
Example 3.13 Poker
Problems
3.4 Multinomial Coefficients
Example 3.14
Example 3.15
Solution
Problems
Notes
Chapter 4 Conditional Probability, Independence, and Markov Chains. 4.1 Introduction
Example 4.1
Problems
4.2 Conditional Probability
Example 4.2
Solution
Example 4.3
Solution
Example 4.4
Solution
Example 4.5
Solution
Problems
4.3 Partitions; Total Probability Formula
Definition 4.3.1
Example 4.6
Example 4.7
Example 4.8
Solution
Example 4.9
Problems
4.4 Bayes' Formula
Example 4.10
Example 4.11
Solution
Example 4.12
Problems
4.5 Independence
Example 4.13
Solution
Example 4.14
Example 4.15
Example 4.16
Problems
4.6 Exchangeability; Conditional Independence
Example 4.17
Problems
4.7 Markov Chains*
Example 4.18
Example 4.19
Example 4.20 Gambler's Ruin
Example 4.21 Division of Stake in Gambler's Ruin
Example 4.22
Example 4.23
Solution
Example 4.24
Example 4.25
Problems
Note
Chapter 5 Random Variables: Univariate Case. 5.1 Introduction
5.2 Distributions of Random Variables
Example 5.1
Solution
Example 5.2
Example 5.3
Example 5.4
Example 5.5
Problems
5.3 Discrete and Continuous Random Variables
Example 5.6
Example 5.7 Binomial Distribution
Example 5.8
Example 5.9 Poisson Distribution
Example 5.10
Example 5.11
Example 5.12
Example 5.13
Solution
Example 5.14 Uniform Distribution
Example 5.15
Example 5.16
Solution
Example 5.17 Normal Distribution
Example 5.18
Solution
Example 5.19
Example 5.20
PROBLEMS
5.4 Functions of Random Variables
Example 5.21
Example 5.22
Example 5.23
Solution
Example 5.24 Linear Transformations
Example 5.25
Example 5.26
Example 5.27 Square of a Normal Random Variable
Example 5.28 Folded Normal Distribution
PROBLEMS
5.5 Survival and Hazard Functions
Example 5.29
Example 5.30
PROBLEMS
Notes
Chapter 6 Random Variables: Multivariate Case. 6.1 Bivariate Distributions
Example 6.1
Example 6.2
Solution
Example 6.3
Problems
6.2 Marginal Distributions; Independence
Example 6.4
Example 6.5
Solution
Example 6.6
Example 6.7
Example 6.8
Solution
Example 6.9
Example 6.10
Example 6.11
Solution
Problems
6.3 Conditional Distributions
Example 6.12
Example 6.13
Example 6.14
Solution
Example 6.15
Solution
Example 6.16
Problems
6.4 Bivariate Transformations
Example 6.17 Sum of Random Variables
Example 6.18 Sum of Exponential Random Variables
Example 6.19 Sum of Two Uniform Random Variables
Example 6.20
Example 6.21 Product of Two Random Variables
Example 6.22 Borel–Kolmogorov Paradox
Solution 1
Problems
6.5 Multidimensional Distributions
Example 6.23 Trinomial Distribution
Example 6.24
Example 6.25 Model of Grinding
Problems
Chapter 7 Expectation. 7.1 Introduction
7.2 Expected Value
Example 7.1
Example 7.2
Example 7.3 Expected Value of Binomial Distribution
Example 7.4 Expectation of Geometric Distribution
Solution
Example 7.5
Example 7.6 Petersburg Paradox
Solution
Example 7.7
Example 7.8
Solution
Example 7.9
Solution
Example 7.10
Example 7.11
Problems
7.3 Expectation as an Integral*
Riemann Integral
Lebesque Integral
Example 7.12
Riemann–Stieltjes Integral
Example 7.13
Example 7.14
Lebesque–Stieltjes Integral
Lebesque Integral: General Case
7.4 Properties of Expectation
Example 7.15
Example 7.16
Example 7.17
Example 7.18
Example 7.19
Problems
7.5 Moments
Example 7.20
Example 7.21
Example 7.22
Example 7.23
Example 7.24
Example 7.25
Example 7.26
Example 7.27
Example 7.28
Example 7.29
Example 7.30
Example 7.31
Problems
7.6 Variance
Example 7.32
Example 7.33
Example 7.34
Example 7.35
Example 7.36
Example 7.37
Example 7.38 Averaging
Example 7.39 A Foot
Example 7.40 Problem of Design
Solution
Example 7.41
Solution
Example 7.42
Example 7.43
Solution
Example 7.44 Moving Averages
Problems
7.7 Conditional Expectation
Example 7.45
Example 7.46
Example 7.47
Example 7.48
Example 7.49
Example 7.50
Example 7.51
Problems
7.8 Inequalities
Example 7.52
Example 7.53
Example 7.54
Example 7.55 Binomial Distribution
Problems
Chapter 8 Selected Families of Distributions
8.1 Bernoulli Trials and Related Distributions
Binomial Distribution
Example 8.1
Solution
Example 8.2 A Warning
Solution
Example 8.3
Solution
Geometric Distribution
Example 8.4
Example 8.5 Family Planning
Negative Binomial Distribution
Example 8.6
Solution
Problems
8.2 Hypergeometric Distribution
Example 8.7
Solution
Problems
8.3 Poisson Distribution and Poisson Process
Definition 8.3.1
Example 8.8
Solution
Example 8.9
Solution
Example 8.10
Example 8.11
Solution
Example 8.12
Solution
Example 8.13
Solution
Example 8.14
Example 8.15
Solution
Problems
8.4 Exponential, Gamma, and Related Distributions
Problems
8.5 Normal Distribution
Example 8.16
Solution
Definition 8.5.2
Example 8.17
Solution
Example 8.18 Sequential Formation
Example 8.19
Problems
8.6 Beta Distribution
Example 8.20
Example 8.21
Solution
Problems
Notes
Chapter 9 Random Samples. 9.1 Statistics and Sampling Distributions
Example 9.1
Solution
Problems
9.2 Distributions Related to Normal
Problems
9.3 Order Statistics
Example 9.2 Distribution of the Range
Example 9.3
Solution
Example 9.4 Theory of Outliers
Problems
9.4 Generating Random Samples
Example 9.5
Example 9.6
Example 9.7
Problems
9.5 Convergence
Example 9.8
Example 9.9 Laws of Large Numbers
Example 9.10
Example 9.11
Weak Laws of Large Numbers
Strong Laws of Large Numbers
Problems
9.6 Central Limit Theorem
Example 9.12
Solution
Example 9.13
Solution
Example 9.14 Decision Problem
Solution
Example 9.15
Example 9.16
Solution
Problems
Notes
Chapter 10 Introduction to Statistical Inference. 10.1 Overview
Example 10.1
Example 10.2
Example 10.3
Example 10.4
Example 10.5
10.2 Basic Models
Example 10.6
10.3 Sampling
Example 10.7
Example 10.8
Example 10.9 Siblings
Solution
Example 10.10 Renewal Paradox
10.4 Measurement Scales
Example 10.11 Scale of Hardness
Example 10.12
Definition 10.4.1
Notes
Chapter 11 Estimation. 11.1 Introduction
Example 11.1
Example 11.2
Example 11.3
Example 11.4
Solution
11.2 Consistency
Example 11.5
Example 11.6
Example 11.7
Example 11.8
Example 11.9
Problems
11.3 Loss, Risk, and Admissibility
Example 11.10
Example 11.11
Example 11.12
Example 11.13
Example 11.14
Problems
11.4 Efficiency
Example 11.15
Example 11.16
Example 11.17
Example 11.18
Example 11.19
Example 11.20
Problems
11.5 Methods of Obtaining Estimators
Method of Moments Estimators
Example 11.21
Example 11.22
Example 11.23
Solution
Example 11.24
Example 11.25
Maximum Likelihood Estimators
Example 11.26
Example 11.27
Example 11.28
Example 11.29
Example 11.30
Example 11.31
Example 11.32
Example 11.33
Example 11.34
Solution
Example 11.35
Least Squares Estimators
Example 11.36
Example 11.37
Example 11.38 Linear Regression
Robust Estimators
Problems
11.6 Sufficiency
Example 11.39
Example 11.40
Example 11.41
Example 11.42
Example 11.43
Example 11.44
Example 11.45
Example 11.46
Example 11.47
Example 11.48
Solution
Example 11.49
Example 11.50
Example 11.51
Example 11.52
Example 11.53
Example 11.54
Example 11.55
Problems
11.7 Interval Estimation
Confidence Intervals
Example 11.56
Example 11.57
Example 11.58
Example 11.59
Example 11.60
Example 11.61
Example 11.62 Confidence Intervals for Variance
Example 11.63
Example 11.64
Example 11.65
Example 11.66
Bootstrap Intervals
Example 11.67
Problems
Notes
Chapter 12 Testing Statistical Hypotheses. 12.1 Introduction
Example 12.1
Example 12.2
Example 12.3
12.2 Intuitive Background
Example 12.4
Example 12.5
Example 12.6
Example 12.7 Randomization
Example 12.8
Example 12.9
Problems
12.3 Most Powerful Tests
Example 12.10
Example 12.11
Example 12.12
Example 12.13
Example 12.14
Problems
12.4 Uniformly Most Powerful Tests
Example 12.15
Example 12.16
Example 12.17
Example 12.18
Example 12.19
Example 12.20 Bernoulli Trials
Problems
12.5 Unbiased Tests
Example 12.21
Example 12.22
Problems
12.6 Generalized Likelihood Ratio Tests
Example 12.23
Example 12.24
Example 12.25 Paired Observations
Example 12.26
Example 12.27
Problems
12.7 Conditional Tests
Example 12.28
Example 12.29
Example 12.30
Example 12.31
Problems
12.8 Tests and Confidence Intervals
12.9 Review of Tests for Normal Distributions
One‐Sample Procedures
Hypotheses About the Mean, Variance Known
Example 12.32 Generic Problem
Example 12.33
12.9.1.2 Hypotheses About the Mean, Variance Unknown
Example 12.34
Hypotheses About the Variance, Mean Known
Hypotheses About the Variance, Mean Unknown
Example 12.35
12.9.2 Two‐Sample Procedures
Hypotheses About the Means, Variances Known
Hypotheses About the Means, Variances Unknown, but
Hypotheses About the Variances, Means Unknown
Hypotheses About the Variances; One or Both Means Known
Large Sample Tests for Binomial Distribution
Example 12.36
Solution
Example 12.37
Solution
12.10 Monte Carlo, Bootstrap, and Permutation Tests
Example 12.38
Monte Carlo Tests
Example 12.39
Bootstrap Tests
Example 12.40
Permutation Tests
Example 12.41
Problems
Notes
Chapter 13 Linear Models. 13.1 Introduction
Example 13.1
Example 13.2 Analysis of Variance or ANOVA
13.2 Regression of the First and Second Kind
Example 13.3
Example 13.4
Example 13.5
Example 13.6
Example 13.7
Example 13.8
PROBLEMS
13.3 Distributional Assumptions
Example 13.9 Logistic Regression
PROBLEMS
13.4 Linear Regression in the Normal Case
Example 13.10 Is It Good to Be a Royal Prince?
Example 13.11
Example 13.12
PROBLEMS
13.5 Testing Linearity
Example 13.13
PROBLEMS
13.6 Prediction
PROBLEMS
13.7 Inverse Regression
Example 13.14
Solution
PROBLEMS
13.8 BLUE
PROBLEMS
13.9 Regression Toward the Mean
Example 13.15 Psychological Test Scores
13.10 Analysis of Variance
13.11 One‐Way Layout
PROBLEMS
13.12 Two‐Way Layout
PROBLEMS
13.13 ANOVA Models with Interaction
Example 13.16
PROBLEMS
13.14 Further Extensions
Notes
Chapter 14 Rank Methods. 14.1 Introduction
Example 14.1
14.2 Glivenko–Cantelli Theorem
Theorem 14.2.1 (Glivenko–Cantelli)
Proof
Problems
14.3 Kolmogorov–Smirnov Tests. One‐Sample Kolmogorov–Smirnov Test
Theorem 14.3.1 (Kolmogorov and Smirnov)
Example 14.2
Solution
Example 14.3
Two‐Sample Kolmogorov–Smirnov Test
Theorem 14.3.2
Example 14.4
Solution
Problems
14.4 One‐Sample Rank Tests
Example 14.5
Example 14.6 Paired Data
Example 14.7
Theorem 14.4.1
Example 14.8
Problems
14.5 Two‐Sample Rank Tests
Example 14.9
Problems
14.6 Kruskal–Wallis Test
Theorem 14.6.1
Problems
Note
Chapter 15 Analysis of Categorical Data. 15.1 Introduction
Example 15.1
Example 15.2
Example 15.3
15.2 Chi‐Square Tests
Example 15.4
Solution
Example 15.5
Example 15.6
Example 15.7
Example 15.8
Problems
15.3 Homogeneity and Independence
Example 15.9
Example 15.10 Prospective and Retrospective Studies
Problems
15.4 Consistency and Power
Example 15.11
Example 15.12
PROBLEMS
15.5 2 x 2 Contingency Tables
Example 15.13
Solution
Example 15.14
Example 15.15
Solution
Example 15.16
Solution
PROBLEMS
15.6 R x C Contingency Tables
Example 15.17
Example 15.18
Example 15.19
PROBLEMS
Chapter 16 Basics of Bayesian Statistics. 16.1 Introduction
Example 16.1
Example 16.2
16.2 Prior and Posterior Distributions
Example 16.3
Example 16.4
Example 16.5
Solution
Example 16.6
Definition 16.2.1
Example 16.7
Example 16.8
Definition 16.2.2
Example 16.9
Example 16.10
Definition 16.2.3
Example 16.11
Example 16.12
PROBLEMS
16.3 Bayesian Inference
Predictive Distribution
Example 16.13
Point Estimation
Definition 16.3.1
Example 16.14
Example 16.15
Example 16.16
Example 16.17
Example 16.18
Solution
Example 16.19
Example 16.20 Are Birds Bayesians?
Bayesian Intervals
Example 16.21
Example 16.22
Bayesian Hypotheses Testing
Definition 16.3.2
Example 16.23
Example 16.24
Example 16.25
Example 16.26
PROBLEMS
16.4 Final Comments
Notes
APPENDIX A Supporting R Code
Example A.1
Example A.2 Obtaining a Histogram with Fitted Normal Density
Example A.3
Example A.4 The randomization test
APPENDIX B Statistical Tables
Bibliography
Answers to Odd‐Numbered Problems. CHAPTER 1
CHAPTER 2
CHAPTER 3
CHAPTER 4
CHAPTER 5
CHAPTER 6
CHAPTER 7
CHAPTER 8
CHAPTER 9
CHAPTER 11
CHAPTER 12
CHAPTER 13
CHAPTER 14
CHAPTER 15
CHAPTER 16
Index
WILEY END USER LICENSE AGREEMENT
Отрывок из книги
Established by Walter A. Shewhart and Samuel S. Wilks
Editors: David J. Balding, Noel A. C. Cressie, Garrett M. Fitzmaurice, Geof H. Givens, Harvey Goldstein, Geert Molenberghs, David W. Scott, Adrian F. M. Smith, Ruey S. Tsay
.....
As already mentioned, the operations of union and intersection can be extended to infinitely many events. Let be an infinite sequence of events. Then,
.....