Planning and Executing Credible Experiments
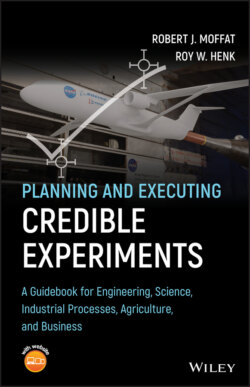
Реклама. ООО «ЛитРес», ИНН: 7719571260.
Оглавление
Robert J. Moffat. Planning and Executing Credible Experiments
Table of Contents
List of Tables
List of Illustrations
Guide
Pages
Planning and Executing Credible Experiments. A Guidebook for Engineering, Science, Industrial Processes, Agriculture, and Business
About the Authors
Preface
Audience
Accompanying Material
Recommended Companion Texts
How Is This Book Used for Teaching?
Acknowledgments
About the Companion Website
1 Choosing Credibility
1.1 The Responsibility of an Experimentalist
1.2 Losses of Credibility
1.3 Recovering Credibility
1.4 Starting with a Sharp Axe
1.5 A Systems View of Experimental Work
1.6 In Defense of Being a Generalist
Panel 1.1 The Bundt Cake Story
The Moral of This Story?
References
Homework
Notes
2 The Nature of Experimental Work
2.1 Tested Guide of Strategy and Tactics
2.2 What Can Be Measured and What Cannot?
2.2.1 Examples Not Measurable
2.2.2 Shapes
2.2.3 Measurable by the Human Sensory System
2.2.4 Identifying and Selecting Measurable Factors
2.2.5 Intrusive Measurements
2.3 Beware Measuring Without Understanding: Warnings from History
2.4 How Does Experimental Work Differ from Theory and Analysis?
2.4.1 Logical Mode
2.4.2 Persistence
2.4.3 Resolution
2.4.4 Dimensionality
2.4.5 Similarity and Dimensional Analysis
2.4.6 Listening to Our Theoretician Compatriots
Panel 2.1 Positive Consequences of the Reproducibility Crisis
Panel 2.2 Selected Invitations to Experimental Research, Insights from Theoreticians
Better Invitation than a Nobel Prize
Einstein's Theory Always Invites Tests
Observations of a Popular Theoretical Physics Field
Another Invitation from Feynman
Extra Invitations to Experiments
Panel 2.3 Prepublishing Your Experiment Plan
2.4.7 Surveys and Polls
2.5 Uncertainty
2.6 Uncertainty Analysis
References
Homework
Notes
3 An Overview of Experiment Planning
3.1 Steps in an Experimental Plan
3.2 Iteration and Refinement
3.3 Risk Assessment/Risk Abatement
3.4 Questions to Guide Planning of an Experiment
Homework
4 Identifying the Motivating Question
4.1 The Prime Need
Panel 4.1 There's a Hole in My Bucket
4.2 An Anchor and a Sieve
4.3 Identifying the Motivating Question Clarifies Thinking
4.3.1 Getting Started
4.3.2 Probe and Focus
4.4 Three Levels of Questions
4.5 Strong Inference
4.6 Agree on the Form of an Acceptable Answer
4.7 Specify the Allowable Uncertainty
4.8 Final Closure
Reference
Homework
Notes
5 Choosing the Approach. 5.1 Laying Groundwork
5.2 Experiment Classifications
5.2.1 Exploratory
5.2.2 Identifying the Important Variables
5.2.3 Demonstration of System Performance
5.2.4 Testing a Hypothesis
5.2.5 Developing Constants for Predetermined Models
5.2.6 Custody Transfer and System Performance Certification Tests
5.2.7 Quality‐Assurance Tests
5.2.8 Summary
5.3 Real or Simplified Conditions?
5.4 Single‐Sample or Multiple‐Sample?
Panel 5.1 A Brief Summary of “Dissertation upon Roast Pig” (with Thanks and Apologies to Charles Lamb [1775–1834])
Panel 5.2 Consider a Spherical Cow
5.5 Statistical or Parametric Experiment Design?
5.6 Supportive or Refutative?
5.7 The Bottom Line
References
Homework
Notes
6 Mapping for Safety, Operation, and Results. 6.1 Construct Multiple Maps to Illustrate and Guide Experiment Plan
6.2 Mapping Prior Work and Proposed Work
6.3 Mapping the Operable Domain of an Apparatus
6.4 Mapping in Operator's Coordinates
6.5 Mapping the Response Surface
6.5.1 Options for Organizing a Table
6.5.2 Options for Presenting the Response on a Scatter‐Plot‐Type Graph
Homework
7 Refreshing Statistics
7.1 Reviving Key Terms to Quantify Uncertainty. 7.1.1 Population
7.1.2 Sample
7.1.3 Central Value
7.1.4 Mean, μ or Y
7.1.5 Residual
7.1.6 Variance, σ2 or S2
7.1.7 Degrees of Freedom, Df
7.1.8 Standard Deviation, σY or SY
7.1.9 Uncertainty of the Mean, δμ
7.1.10 Chi‐Squared, χ2
7.1.11 p‐Value
7.1.12 Null Hypothesis
7.1.13 F‐value of Fisher Statistic
7.2 The Data Distribution Most Commonly Encountered. The Normal Distribution for Samples of Infinite Size
7.3 Account for Small Samples: The t‐Distribution
7.4 Construct Simple Models by Computer to Explain the Data
7.4.1 Basic Statistical Analysis of Quantitative Data
7.4.2 Model Data Containing Categorical and Quantitative Factors
7.4.3 Display Data Fit to One Categorical Factor and One Quantitative Factor
7.4.4 Quantify How Each Factor Accounts for Variation in the Data
7.5 Gain Confidence and Skill at Statistical Modeling Via the R Language
7.5.1 Model and Plot Results of a Single Variable Using the Example Data diceshoe.csv
7.5.2 Evaluate Alternative Models of the Example Data hiloy.csv (Sections 7.5.3 Through 7.5.8) 7.5.2.1 Inspect the Data
7.5.3 Grand Mean
7.5.4 Model by Groups: Group‐Wise Mean
7.5.5 Model by a Quantitative Factor
7.5.6 Model by Multiple Quantitative Factors
7.5.7 Allow Factors to Interact (So Each Group Gets Its Own Slope)
7.5.8 Include Polynomial Factors (a Statistical Linear Model Can Be Curved)
7.6 Report Uncertainty
7.7 Decrease Uncertainty (Improve Credibility) by Isolating Distinct Groups
7.8 Original Data, Summary, and R
References
Homework
Notes
8 Exploring Statistical Design of Experiments. 8.1 Always Seeking Wiser Strategies
8.2 Evolving from Novice Experiment Design
8.3 Two‐Level and Three‐Level Factorial Experiment Plans
8.4 A Three‐Level, Three‐Factor Design
8.5 The Plackett–Burman 12‐Run Screening Design
8.6 Details About Analysis of Statistically Designed Experiments
8.6.1 Model Main Factors to Original Raw Data
8.6.2 Model Main Factors to Original Data Around Center of Each Factor
8.6.3 Model Including All Interaction Terms
8.6.4 Model Including Only Dominant Interaction Terms
8.6.5 Model Including Dominant Interaction Term Plus Quadratic Term
8.6.6 Model All Factors of Example 2, Centering Each Quantitative Factor
8.6.7 Refine Model of Example 2 Including Only Dominant Terms
8.7 Retrospect of Statistical Design Examples
8.8 Philosophy of Statistical Design
8.9 Statistical Design for Conditions That Challenge Factorial Designs
8.10 A Highly Recommended Tool for Statistical Design of Experiments
8.11 More Tools for Statistical Design of Experiments
8.12 Conclusion
Further Reading
Homework
Notes
9 Selecting the Data Points
9.1 The Three Categories of Data
9.1.1 The Output Data
9.1.2 Peripheral Data
9.1.3 Backup Data
9.1.4 Other Data You May Wish to Acquire
9.2 Populating the Operating Volume. 9.2.1 Locating the Data Points Within the Operating Volume
9.2.2 Estimating the Topography of the Response Surface
9.3 Example from Velocimetry
9.3.1 Sharpen Our Approach
9.3.2 Lessons Learned from Velocimetry Example
9.4 Organize the Data. 9.4.1 Keep a Laboratory Notebook
9.4.2 Plan for Data Security
9.4.3 Decide Data Format
9.4.4 Overview Data Guidelines
9.4.5 Reasoning Through Data Guidelines
9.5 Strategies to Select Next Data Points
9.5.1 Overview of Option 1: Default Strategy with Intensive Experimenter Involvement
9.5.1.1 Choosing the Data Trajectory
9.5.1.2 The Default Strategy: Be Bold
9.5.1.2.1 Halve Rather than Nibble
9.5.1.3 Anticipate, Check, Course Correct
9.5.1.4 Other Aspects to Keep in Mind
9.5.1.5 Endpoints
9.5.2 Reintroducing Gosset
9.5.3 Practice Gosset Examples (from Gosset User Manual)
9.6 Demonstrate Gosset for Selecting Data
9.6.1 Status Quo of Experiment Planning and Execution (Prior to Selecting More Samples)
9.6.1.1 Specified Motivating Question
9.6.1.2 Identified Pertinent Candidate Factors
9.6.1.3 Selected Initial Sample Points Using Plackett–Burman
9.6.1.4 Executed the First 12 Runs at the PB Sample Conditions
9.6.1.5 Analyzed Results. Identified Dominant First‐Order Factors. Estimated First‐Order Uncertainties of Factors
9.6.1.6 Generated Draft Predictive Equation
9.6.2 Use Gosset to Select Additional Data Samples
9.6.2.1 Example Gosset Session: User Input to Select Next Points
9.6.2.2 Example Gosset Session: How We Chose User Input
9.6.2.3 Example Gosset Session: User Input Along with Gosset Output
9.6.2.4 Example Gosset Session: Convert the Gosset Design to Operator Values
9.6.2.5 Results of Example Gosset Session: Operator Plots of Total Experiment Plan
9.6.2.6 Execute Stage Two of the Experiment Plan: User Plus Gosset Sample Points
9.7 Use Gosset to Analyze Results
9.8 Other Options and Features of Gosset
9.9 Using Gosset to Find Local Extrema in a Function of Several Variables
9.10 Summary
Further Reading
Homework
10. Analyzing Measurement Uncertainty. 10.1 Clarifying Uncertainty Analysis
10.1.1 Distinguish Error and Uncertainty
10.1.1.1 Single‐Sample vs. Multiple‐Sample
10.1.2 Uncertainty as a Diagnostic Tool
10.1.2.1 What Can Uncertainty Analysis Tell You?
10.1.2.2 What Is Uncertainty Analysis Good For?
10.1.2.3 Uncertainty Analysis Can Redirect a Poorly Conceived Experiment
10.1.2.4 Uncertainty Analysis Improves the Quality of Your Work
10.1.2.5 Slow Sampling and “Randomness”
10.1.2.6 Uncertainty Analysis Makes Results Believable
10.1.3 Uncertainty Analysis Aids Management Decision‐Making
10.1.3.1 Management's Task: Dealing with Warranty Issues
10.1.4 The Design Group's Task: Setting Tolerances on Performance Test Repeatability
10.1.5 The Performance Test Group's Task: Setting the Tolerances on Measurements
10.2 Definitions
10.2.1 True Value
10.2.2 Corrected Value
10.2.3 Data Reduction Program
10.2.4 Accuracy
10.2.5 Error
10.2.6 XXXX Error
10.2.7 Fixed Error
10.2.8 Residual Fixed Error
10.2.9 Random Error
10.2.10 Variable (but Deterministic) Error
10.2.11 Uncertainty
10.2.12 Odds
10.2.13 Absolute Uncertainty
10.2.14 Relative Uncertainty
10.3 The Sources and Types of Errors
10.3.1 Types of Errors: Fixed, Random, and Variable
10.3.2 Sources of Errors: The Measurement Chain
10.3.2.1 The Undisturbed Value
10.3.2.2 The Available Value
10.3.2.3 The Achieved Value
10.3.2.4 The Observed Value
10.3.2.5 The Corrected Value
10.3.3 Specifying the True Value
10.3.3.1 If the Achieved Value Is Taken as the True Value
10.3.3.2 If the Available Value Is Taken as the True Value
10.3.3.3 If the Undisturbed Value Is Taken as the True Value
10.3.3.4 If the Mixed Mean Gas Temperature Is Taken as the True Value
10.3.4 The Role of the End User
10.3.4.1 The End‐Use Equations Implicitly Define the True Value
10.3.5 Calibration
10.4 The Basic Mathematics
10.4.1 The RSS Combination
10.4.2 The Fixed Error in a Measurement
10.4.3 The Random Error in a Measurement
10.4.4 The Uncertainty in a Measurement
10.4.5 The Uncertainty in a Calculated Result
10.4.5.1 The Relative Uncertainty in a Result
10.5 Automating the Uncertainty Analysis
10.5.1 The Mathematical Basis
10.5.2 Example of Uncertainty Analysis by Spreadsheet
10.6 Single‐Sample Uncertainty Analysis
10.6.1 Assembling the Necessary Inputs
10.6.2 Calculating the Uncertainty in the Result
10.6.3 The Three Levels of Uncertainty: Zeroth‐, First‐, and Nth‐Order
10.6.3.1 Zeroth‐Order Replication
10.6.3.2 First‐Order Replication
10.6.3.3 Nth‐Order Replication
10.6.4 Fractional‐Order Replication for Special Cases
10.6.5 Summary of Single‐Sample Uncertainty Levels. 10.6.5.1 Zeroth‐Order
10.6.5.2 First‐Order
10.6.5.3 Nth‐Order
References
Further Reading
Homework
11 Using Uncertainty Analysis in Planning and Execution
11.1 Using Uncertainty Analysis in Planning
11.1.1 The Physical Situation and Energy Analysis
11.1.2 The Steady‐State Method
11.1.3 The Transient Method
11.1.4 Reflecting on Assumptions Made During DRE Derivations
11.2 Perform Uncertainty Analysis on the DREs. 11.2.1 Uncertainty Analysis: General Form
11.2.2 Uncertainty Analysis of the Steady‐State Method
11.2.3 Uncertainty Analysis – Transient Method
11.2.4 Compare the Results of Uncertainty Analysis of the Methods
Planning Decisions
11.2.5 What Does the Calculated Uncertainty Interval Mean?
11.2.6 Cross‐Checking the Experiment
11.2.7 Conclusions
11.3 Using Uncertainty Analysis in Selecting Instruments
11.4 Using Uncertainty Analysis in Debugging an Experiment
11.4.1 Handling Overall Scatter
11.4.2 Sources of Scatter
11.4.3 Advancing Toward Calibration
11.4.4 Selecting Thresholds
11.4.5 Iterating Analysis
11.4.6 Rechecking Situational Uncertainty
11.5 Reporting the Uncertainties in an Experiment
11.5.1 Progress in Uncertainty Reporting
11.6 Multiple‐Sample Uncertainty Analysis. 11.6.1 Revisiting Single‐Sample and Multiple‐Sample Uncertainty Analysis
11.6.2 Examples of Multiple‐Sample Uncertainty Analysis
11.6.3 Fixed Error and Random Error
11.7 Coordinate with Uncertainty Analysis Standards
11.7.1 Describing Fixed and Random Errors in a Measurement
11.7.2 The Bias Limit
11.7.2.1 Fossilization
11.7.2.2 Bias Limits
11.7.3 The Precision Index
11.7.4 The Number of Degrees of Freedom
11.8 Describing the Overall Uncertainty in a Single Measurement
11.8.1 Adjusting for a Single Measurement
11.8.2 Describing the Overall Uncertainty in a Result
11.8.3 Adding the Overall Uncertainty to Predictive Models
11.9 Additional Statistical Tools and Elements
11.9.1 Pooled Variance
11.9.1.1 Student's t‐Distribution – Pooled Examples
11.9.2 Estimating the Standard Deviation of a Population from the Standard Deviation of a Small Sample: The Chi‐Squared χ2 Distribution
References
Homework
12 Debugging an Experiment, Shakedown, and Validation. 12.1 Introduction
12.2 Classes of Error
12.3 Using Time‐Series Analysis in Debugging
12.4 Examples
12.4.1 Gas Temperature Measurement
12.4.2 Calibration of a Strain Gauge
12.4.3 Lessons Learned from Examples
12.5 Process Unsteadiness
12.6 The Effect of Time‐Constant Mismatching
12.7 Using Uncertainty Analysis in Debugging an Experiment
12.7.1 Calibration and Repeatability
12.7.2 Stability and Baselining
12.8 Debugging the Experiment via the Data Interpretation Program
12.8.1 Debug the Experiment via the DIP
12.8.2 Debug the Interface of the DIP
12.8.3 Debug Routines in the DIP
12.9 Situational Uncertainty
13 Trimming Uncertainty. 13.1 Focusing on the Goal
13.2 A Mlotivating Question for Industrial Production
13.2.1 Agreed Motivating Questions for Industrial Example
13.2.2 Quick Answers to Motivating Questions
13.2.3 Challenge: Precheck Analysis and Answers
13.3 Plackett–Burman 12‐Run Results and Motivating Question #3
13.4 PB 12‐Run Results and Motivating Question #1
13.4.1 Building a Predictive Model Equation from R‐Language Linear Model
13.4.2 Parsing the Dual Predictive Model Equation
13.4.3 Uncertainty of the Intercept in the Dual Predictive Model Equation
13.4.4 Mapping an Answer to Motivating Question #1
13.4.5 Tentative Answers to Motivating Question #1
13.5 Uncertainty Analysis of Dual Predictive Model and Motivating Question #2
13.5.1 Uncertainty of the Constant in the Dual Predictive Model Equation
13.5.2 Uncertainty of Other Factors in the Dual Predictive Model Equation
13.5.3 Include All Coefficient Uncertainties in the Dual Predictive Model Equation
13.5.4 Overall Uncertainty from All Factors in the Predictive Model Equation
13.5.5 Improved Tentative Answers to Motivating Questions, Including Uncertainties
13.5.6 Search for Improved Predictive Models
13.6 The PB 12‐Run Results and Individual Machine Models
13.6.1 Individual Machine Predictive Model Equations
13.6.2 Uncertainty of the Intercept in the Individual Predictive Model Equations
13.6.3 Uncertainty of the Constant in the Individual Predictive Model Equations
13.6.4 Uncertainty of Other Factors in the Individual Predictive Model Equation
13.6.4.1 Uncertainties of Machine 1
13.6.4.2 Uncertainties of Machine 2
13.6.4.3 Including Instrument and Measurement Uncertainties
13.6.5 Include All Coefficient Uncertainties in the Individual Predictive Model Equations
13.6.6 Overall Uncertainty from All Factors in the Individual Predictive Model Equations
13.6.7 Quick Overview of Individual Machine Performance Over the Operating Map
13.7 Final Answers to All Motivating Questions for the PB Example Experiment
13.7.1 Answers to Motivating Question #1
13.7.2 Answers to Motivating Question #2
13.7.3 Answers to Motivating Question #3 (Expanded from Section 13.3)
13.7.4 Answers to Motivating Question #4
13.7.5 Other Recommendations (to Our Client)
13.8 Conclusions
Homework
Notes
14 Documenting the Experiment : Report Writing
14.1 The Logbook
14.2 Report Writing
14.2.1 Organization of the Reports
14.2.2 Who Reads What?
14.2.3 Picking a Viewpoint
14.2.4 What Goes Where?
14.2.4.1 What Goes in the Abstract?
14.2.4.2 What Goes in the Foreword?
14.2.4.3 What Goes in the Objective?
14.2.4.4 What Goes in the Results and Conclusions?
14.2.4.5 What Goes in the Discussion?
14.2.4.6 References
14.2.4.7 Figures
14.2.4.8 Tables
14.2.4.9 Appendices
14.2.5 The Mechanics of Report Writing
14.2.6 Clear Language Versus “JARGON”
Panel 14.1 The Turbo‐Encabulator
14.2.7 “Gobbledygook”: Structural Jargon
Panel 14.2 U.S. Code, Title 18, No. 793 *
14.2.8 Quantitative Writing
14.2.8.1 Substantive Versus Descriptive Writing
Panel 14.3 The Descriptive Bank Statement
Abstract
14.2.8.2 Zero‐Information Statements
14.2.8.3 Change
14.3 International Organization for Standardization, ISO 9000 and other Standards
14.4 Never Forget. Always Remember
Notes
Appendix A Distributing Variation and Pooled Variance. A.1 Inescapable Distributions. A.1.1 The Normal Distribution for Samples of Infinite Size
A.1.2 Adjust Normal Distributions with Few Data: The Student’s t‐Distribution
A.2 Other Common Distributions
A.2.1.1 Discrete Distributions
A.2.1.2 Continuous Distributions
A.3 Pooled Variance (Advanced Topic)
Note
Appendix B Illustrative Tables for Statistical Design. B.1 Useful Tables for Statistical Design of Experiments. B.1.1 Ready‐made Ordering for Randomized Trials
B.1.2 Exhausting Sets of Two‐Level Factorial Designs (≤ Five Factors)
B.2 The Plackett–Burman (PB) Screening Designs
Appendix C Hand Analysis of Two‐Level Factorial Designs
C.1 The General Two‐Level Factorial Design
C.2 Estimating the Significance of the Apparent Factor Effects
C.3 Hand Analysis of a Plackett–Burman (PB) 12‐Run Design
C.4 Illustrative Practice Example for the PB 12‐Run Pattern
C.4.1 Assignment: Find Factor Effects and the Linear Coefficients Absent Noise
C.4.2 Assignment: Find Factor Effects and the Linear Coefficients with Noise
C.5 Answer Key: Compare Your Hand Calculations. C.5.1 Expected Results Absent Noise (compare C.4.1)
C.5.2 Expected Results with Random Gaussian Noise (cf. C.4.2)
C.6 Equations for Hand Calculations
Appendix D Free Recommended Software: Obtain Recommended Free, Open‐Source Software for Your Computer. D.1 Instructions to Obtain the R Language for Statistics
D.2 Instructions to Obtain LibreOffice
D.3 Instructions to Obtain Gosset
D.4 Possible Use of RStudio
Index. a
b
c
d
e
f
g
h
i
j
k
l
m
n
o
p
q
r
s
t
u
v
w
WILEY END USER LICENSE AGREEMENT
Отрывок из книги
Robert J. Moffat
.....
NASA provides a good example of the interdependence of theory and experiment. The National Advisory Council on Aeronautics (NACA) was the precursor of NASA; “Aeronautics” is the first A of NASA. As airplane designs rapidly advanced during the 1900s, NASA deliberately adopted a four‐pronged approach: theory, scale‐model testing (wind‐tunnel experiments), full‐scale testing (in‐flight experiments), and numerical simulation (computational models verified by experiment). Each of the first three prongs have always been essential (Baals and Corliss 1981). Since the 1980s, numerical simulation has aided theory. Theory and experiment need each other. Since our numerical colleagues often refer to their “numerical experiments,” we do advocate an appropriate way to report the uncertainties of their results, just as we experimentalists do.
The science of fluid flow remains important, as another quote (from a personal letter) from Feynman makes clear:
.....