Elementary Regression Modeling
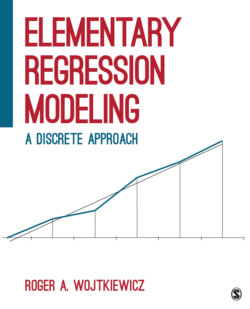
Реклама. ООО «ЛитРес», ИНН: 7719571260.
Оглавление
Roger A. Wojtkiewicz. Elementary Regression Modeling
Elementary Regression Modeling
Brief Contents
Detailed Contents
Preface. Purpose of the Book
Intended Audience
Unique Contributions
Special Features
Acknowledgments
About the Author
1 Introductory Ideas
Regression Modeling
Control Modeling
Modeling Interactions
Modeling Linearity with Splines
Testing Research Hypotheses
Classical Approach to Regression
Disadvantages of Classical Approach
Discrete Approach to Regression
Summary
Key Concepts
2 Basic Statistical Procedures
Individual Units and Groups
Measurement
Level of Measurement
Examples for Level of Measurement
Count, Sum, and Transformations
Mean
Proportion and Percentage
Odds and Log Odds
Examples of Means and Log Odds
Differences
Summary
Key Concepts
Chapter Exercises
3 Regression Modeling Basics
Difference Between Means: The t Test
Linear Regression With a Two-Category Independent Variable
Logistic Regression With a Two-Category Independent Variable
Linear Regression With a Four-Category Independent Variable
Logistic Regression With a Four-Category Independent Variable
Modeling Linear Effect With Dummy Variables
Linear Coefficient in Linear Regression
Linear Coefficient in Logistic Regression
Using Dummy Variables for a Continuous Variable
Summary
Key Concepts
Chapter Exercises
4 Key Regression Modeling Concepts
Unit Vector: Estimating the Intercept
Nestedness
Higher Order Differences
Constraints
Summary
Key Concepts
Chapter Exercises
5 Control Modeling
Elementary Control Modeling
Elaboration for Controlling
Demographic Standardization for Controlling
Small and Big Models
Allocating Influence With Multiple Control Variables
One-at-a-Time Without Controls
Step Approach
One-at-a-Time With Controls
Hybrid Approach
Nestedness and Constraints
Example Using Logistic Regression
Summary
Key Concepts
Chapter Exercises
6 Modeling Interactions
Interactions as Conditional Differences
Interactions Between Dummy Variables
Interactions Between Dummy Variables and an Interval Variable
Three-Way Interactions
Estimating Separate Models
Example Using Logistic Regression
Summary
Key Concepts
Chapter Exercises
7 Modeling Linearity With Splines
Dummy Variables Nested in Interval Variable
Introduction to Knotted Spline Variables
Spline Variables Nested in an Interval Variable
Regression Modeling Using Spline Variables
Working With a Continuous Independent Variable
Example Using Logistic Regression
Summary
Key Concepts
Chapter Exercises
8 Conclusion Testing Research Hypotheses
Bivariate Hypothesis/No Controls
Bivariate Hypothesis/Unanalyzed Controls
Bivariate Hypothesis/Analyzed Controls
Hypothesis Involving Interactions
Hypothesis Involving Nonlinearity
Final Comments
Summary
Key Concepts
Chapter Exercises
Appendix A: Creating the Hsls Data File. Accessing and Downloading the HSLS data
Creating the HSLS Extract
Access helpful study tools and resources—all in one place!
Appendix B: Codebook for Hsls Variables
Appendix C: Creating Hsls Variables
Chapter 2. Basic Statistical Procedures
Chapter 3. Regression Modeling Basics
Chapter 4. Key Regression Modeling Concepts
Chapter 5. Control Modeling
Chapter 6. Modeling Interactions
Chapter 7. Modeling Linearity With Splines
Answers to Chapter Exercises. Chapter 2. Basic Statistical Procedures
Chapter 3. Regression Modeling Basics
Chapter 4. Key Regression Modeling Concepts
Chapter 5. Control Modeling
Chapter 6. Modeling Interactions
Chapter 7. Modeling Linearity With Splines
Chapter 8. Testing Research Hypotheses
References
Index
Отрывок из книги
To Elaine.
My second purpose was to provide a source on how to do regression modeling in social science research. I define regression modeling in my book as the use of one or more regression equations to examine a particular coefficient in greater depth. To do that, we need to address research hypotheses. The many books on regression analysis cover well the standard continuous, cloud-of-points approach to explaining regression analysis, technical issues in regard to estimating appropriate regression models given the data at hand, and advanced methods for doing regression analysis. However, what has not been addressed thoroughly is how to use a series of multiple regression equations to examine the contributions of control variables, different approaches to estimating interaction effects, and methods for examining linearity in regression effects.
.....
The odds ratio in horse racing describes a horse’s chances of losing. An odds of 4:1 indicates that a horse is expected to lose four times for each win.
However, the dependent variable in logistic regression is not the odds, which would be difficult enough to interpret, but the dependent variable is the log odds, where the log is taken to the base e and is thus the natural logarithm (ln):
.....