Profit Maximization Techniques for Operating Chemical Plants
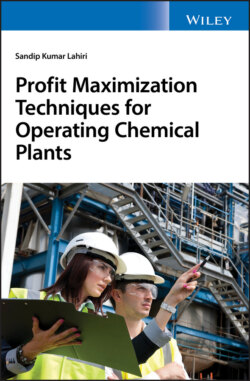
Реклама. ООО «ЛитРес», ИНН: 7719571260.
Оглавление
Sandip K. Lahiri. Profit Maximization Techniques for Operating Chemical Plants
Table of Contents
List of Tables
List of Illustrations
Guide
Pages
Profit Maximization Techniques for Operating Chemical Plants
Figure List
Table List
Preface
Overview of Contents
Concept of Profit Maximization
Big Picture of the Modern Chemical Industry
Profit Maximization Project (PMP) Implementation Steps
Strategy of Profit Maximization
Key Performance Indicators and Targets
Assessment of Current Plant Status
Process Modeling by an Artificial Neural Network
Optimization of Industrial Processes and Process Equipment
Process Monitoring
Fault Diagnosis
Optimization of the Existing Distillation Column
New Design Methodology
Genetic Programing for Modeling of Industrial Reactors
Maximum Capacity Test Run and Debottlenecking Study
Loss Assessment
Advance Process Control
150 Ways and Best Practices to Improve Profit in Running a Chemical Plant
1 Concept of Profit Maximization. 1.1 Introduction
1.2 Who is This Book Written for?
1.3 What is Profit Maximization and Sweating of Assets All About?
1.4 Need for Profit Maximization in Today's Competitive Market
1.5 Data Rich but Information Poor Status of Today's Process Industries
1.6 Emergence of Knowledge‐Based Industries
1.7 How Knowledge and Data Can Be Used to Maximize Profit
References
2 Big Picture of the Modern Chemical Industry. 2.1 New Era of the Chemical Industry
2.2 Transition from a Conventional to an Intelligent Chemical Industry
2.3 How Will Digital Affect the Chemical Industry and Where Can the Biggest Impact Be Expected?
2.3.1 Attaining a New Level of Functional Excellence
2.3.1.1 Manufacturing
2.3.1.2 Supply Chain
2.3.1.3 Sales and Marketing
2.3.1.4 Research and Development
2.4 Using Advanced Analytics to Boost Productivity and Profitability in Chemical Manufacturing
2.4.1 Decreasing Downtime Through Analytics
2.4.2 Increase Profits with Less Resources
2.4.3 Optimizing the Whole Production Process
2.5 Achieving Business Impact with Data
2.5.1 Data's Exponential Growing Importance in Value Creation
2.5.2 Different Links in the Value Chain
2.5.2.1 The Insights Value Chain – Definitions and Considerations (Holger Hürtgen, 2018)
2.6 From Dull Data to Critical Business Insights: The Upstream Processes
2.6.1 Generating and Collecting Relevant Data
2.6.2 Data Refinement is a Two‐Step Iteration
2.7 From Valuable Data Analytics Results to Achieving Business Impact: The Downstream Activities
2.7.1 Turning Insights into Action
2.7.2 Developing Data Culture
2.7.3 Mastering Tasks Concerning Technology and Infrastructure as Well as Organization and Governance
References
3 Profit Maximization Project (PMP) Implementation Steps. 3.1 Implementing a Profit Maximization Project (PMP)
3.1.1 Step 1: Mapping the Whole Plant in Monetary Terms
3.1.2 Step 2: Assessment of Current Plant Conditions
3.1.3 Step 3: Assessment of the Base Control Layer of the Plant
3.1.4 Step 4: Assessment of Loss from the Plant
3.1.5 Step 5: Identification of Improvement Opportunity in Plant and Functional Design of PMP Applications
3.1.6 Step 6: Develop an Advance Process Monitoring Framework by Applying the Latest Data Analytics Tools
3.1.7 Step 7: Develop a Real‐Time Fault Diagnosis System
3.1.8 Step 8: Perform a Maximum Capacity Test Run
3.1.9 Step 9: Develop and Implement Real‐Time APC
3.1.10 Step 10: Develop a Data‐Driven Offline Process Model for Critical Process Equipment
3.1.11 Step 11: Optimizing Process Operation with a Developed Model
3.1.12 Step 12: Modeling and Optimization of Industrial Reactors
3.1.13 Step 13: Maximize Throughput of All Running Distillation Columns
3.1.14 Step 14: Apply New Design Methodology for Process Equipment
References
4 Strategy for Profit Maximization. 4.1 Introduction
4.2 How is Operating Profit Defined in CPI?
4.3 Different Ways to Maximize Operating Profit
4.4 Process Cost Intensity. 4.4.1 Definition of Process Cost Intensity
4.4.2 Concept of Cost Equivalent (CE)
4.4.3 Cost Intensity for a Total Site
4.5 Mapping the Whole Process in Monetary Terms and Gain Insights
4.6 Case Study of a Glycol Plant
4.7 Steps to Map the Whole Plant in Monetary Terms and Gain Insights
4.7.1 Step 1: Visualize the Plant as a Black Box
4.7.2 Step 2: Data Collection from a Data Historian and Preparation of Cost Data
4.7.3 Step 3: Calculation of Profit Margin
4.7.4 Step 4: Gain Insights from Plant Cost and Profit Data
4.7.5 Step 5: Generation of Production Cost and a Profit Margin Table for One Full Year
4.7.6 Step 6: Plot Production Cost and Profit Margin for One Full Year and Gain Insights
4.7.7 Step 7: Calculation of Relative Standard Deviations of each Parameter in order to Understand the Cause of Variability
4.7.8 Step 8: Cost Benchmarking
Reference
5 Key Performance Indicators and Targets
5.1 Introduction
5.2 Key Indicators Represent Operation Opportunities
5.2.1 Reaction Optimization
5.2.2 Heat Exchanger Operation Optimization
5.2.3 Furnace Operation
5.2.4 Rotating Equipment Operation
5.2.5 Minimizing Steam Letdown Flows
5.2.6 Turndown Operation
5.2.7 Housekeeping Aspects
5.3 Define Key Indicators
5.3.1 Process Analysis and Economics Analysis
5.3.2 Understand the Constraints
5.3.3 Identify Qualitatively Potential Area of Opportunities
5.4 Case Study of Ethylene Glycol Plant to Identify the Key Performance Indicator. 5.4.1 Methodology
5.4.2 Ethylene Oxide Reaction Section. 5.4.2.1 Understand the Process
5.4.2.2 Understanding the Economics of the Process
5.4.2.3 Factors that can Change the Production Cost and Overall Profit Generated from this Section
5.4.2.4 How is Production Cost Related to Process Parameters from the Standpoint of the Cause and Effect Relationship?
5.4.2.5 Constraints
5.4.2.6 Key Parameter Identifications
5.4.3 Cycle Water System. 5.4.3.1 Main Purpose
5.4.3.2 Economics of the Process
5.4.3.3 Factors that can Change the Production Cost of this Section
5.4.3.4 Constraints
5.4.3.5 Key Performance Parameters
5.4.4 Carbon Dioxide Removal Section. 5.4.4.1 Main Purpose
5.4.4.2 Economics
5.4.4.3 Factors that can Change the Production Cost of this Section
5.4.4.4 Constraints
5.4.4.5 Key Performance Parameters
5.4.5 EG Reaction and Evaporation Section. 5.4.5.1 Main Purpose
5.4.5.2 Economics
5.4.5.3 Factors that can Change the Production Cost of this Section
5.4.5.4 Key Performance Parameters
5.4.6 EG Purification Section. 5.4.6.1 Main Purpose
5.4.6.2 Economics
5.4.6.3 Key Performance Parameters
5.5 Purpose to Develop Key Indicators
5.6 Set up Targets for Key Indicators
5.7 Cost and Profit Dashboard. 5.7.1 Development of Cost and Profit Dashboard to Monitor the Process Performance in Money Terms
5.7.2 Connecting Key Performance Indicators in APC
5.8 It is Crucial to Change the Viewpoints in Terms of Cost or Profit
References
6 Assessment of Current Plant Status
6.1 Introduction
6.1.1 Data Extraction from a Data Historian
6.1.2 Calculate the Economic Performance of the Section
6.2 Monitoring Variations of Economic Process Parameters
6.3 Determination of the Effect of Atmosphere on the Plant Profitability
6.4 Capacity Variations
6.5 Assessment of Plant Reliability
6.6 Assessment of Profit Suckers and Identification of Equipment for Modeling and Optimization
6.7 Assessment of Process Parameters Having a High Impact on Profit
6.8 Comparison of Current Plant Performance Against Its Design
6.9 Assessment of Regulatory Control System Performance
6.9.1 Basic Assessment Procedure
6.10 Assessment of Advance Process Control System Performance
6.11 Assessment of Various Profit Improvement Opportunities
References
7 Process Modeling by the Artificial Neural Network. 7.1 Introduction
7.2 Problems to Develop a Phenomenological Model for Industrial Processes
7.3 Types of Process Model
7.3.1 First Principle‐Based Model
7.3.2 Data‐Driven Models
7.3.3 Grey Model
7.3.4 Hybrid Model
7.4 Emergence of Artificial Neural Networks as One of the Promising Data‐Driven Modeling Techniques
7.5 ANN‐Based Modeling. 7.5.1 How Does ANN Work?
7.5.2 Network Architecture
7.5.3 Back‐Propagation Algorithm (BPA)
7.5.4 Training
7.5.5 Generalizability
7.6 Model Development Methodology
7.6.1 Data Collection and Data Inspection
7.6.2 Data Pre‐processing and Data Conditioning (Lahiri, 2017)
7.6.2.1 Outlier Detection and Replacement (Lahiri, 2017)
7.6.2.2 Univariate Approach to Detect Outliers
7.6.2.3 Multivariate Approach to Detect Outliers (Lin, 2007)
7.6.3 Selection of Relevant Input–Output Variables
7.6.4 Align Data
7.6.5 Model Parameter Selection, Training, and Validation (Kadlec, Gabrys, & Strandt, 2009; Lin, 2007)
7.6.6 Model Acceptance and Model Tuning
7.7 Application of ANN Modeling Techniques in the Chemical Process Industry
7.8 Case Study: Application of the ANN Modeling Technique to Develop an Industrial Ethylene Oxide Reactor Model. 7.8.1 Origin of the Present Case Study
7.8.2 Problem Definition of the Present Case Study
7.8.3 Developing the ANN‐Based Reactor Model (Lahiri & Khalfe, 2008, 2009b, 2010)
7.8.4 Identifying Input and Output Parameters
7.8.5 Data Collection
7.8.6 Neural Regression
7.8.7 Results and Discussions
7.9 Matlab Code to Generate the Best ANN Model
References
Appendix 7.1 Matlab Code to Generate the Best ANN Model
8 Optimization of Industrial Processes and Process Equipment. 8.1 Meaning of Optimization in an Industrial Context
8.2 How Can Optimization Increase Profit?
8.3 Types of Optimization
8.3.1 Steady‐State Optimization
8.3.2 Dynamic Optimization
8.4 Different Methods of Optimization. 8.4.1 Classical Method
8.4.2 Gradient‐Based Methods of Optimization
8.4.3 Non‐traditional Optimization Techniques
8.5 Brief Historical Perspective of Heuristic‐based Non‐traditional Optimization Techniques
8.6 Genetic Algorithm. 8.6.1 What is Genetic Algorithm?
8.6.2 Foundation of Genetic Algorithms
8.6.3 Five Phases of Genetic Algorithms
8.6.3.1 Initial Population
8.6.3.2 Fitness Function
8.6.3.3 Selection
8.6.3.4 Crossover
8.6.3.5 Termination
8.6.4 The Problem Definition
8.6.5 Calculation Steps of GA (Babu, 2004)
8.6.5.1 Step 1: Generating Initial Population by Creating Binary Coding
8.6.5.2 Step 2: Evaluation of Fitness
8.6.5.3 Step 3: Selecting the Next Generation's Population
8.6.6 Advantages of GA Against Classical Optimization Techniques
8.7 Differential Evolution. 8.7.1 What is Differential Evolution (DE)?
8.7.2 Working Principle of DE (Babu, 2004)
8.7.3 Calculation Steps Performed in DE
8.7.4 Choice of DE Key Parameters (NP, F, and CR)
8.7.5 Stepwise Calculation Procedure for DE implementation (Babu, 2004)
8.8 Simulated Annealing. 8.8.1 What is Simulated Annealing?
8.8.2 Procedure (Babu, 2004)
8.8.3 Algorithm
8.9 Case Study: Application of the Genetic Algorithm Technique to Optimize the Industrial Ethylene Oxide Reactor
8.9.1 Conclusion of the Case Study
8.10 Strategy to Utilize Data‐Driven Modeling and Optimization Techniques to Solve Various Industrial Problems and Increase Profit
References
Appendix 8.1 Matlab Code for GA Optimization of an EO Reactor Case Study
9 Process Monitoring. 9.1 Need for Advance Process Monitoring
9.2 Current Approaches to Process Monitoring and Diagnosis
9.3 Development of an Online Intelligent Monitoring System
9.4 Development of KPI‐Based Process Monitoring
9.5 Development of a Cause and Effect‐Based Monitoring System
9.6 Development of Potential Opportunity‐Based Dash Board
9.6.1 Development of Loss and Waste Monitoring Systems
9.6.2 Development of a Cost‐Based Monitoring System
9.6.3 Development of a Constraints‐Based Monitoring System
9.7 Development of Business Intelligent Dashboards
9.8 Development of Process Monitoring System Based on Principal Component Analysis
9.8.1 What is a Principal Component Analysis?
9.8.2 Why Do We Need to Rotate the Data?
9.8.3 How Do We Generate Principal Components?
9.8.4 Steps to Calculating the Principal Components
9.9 Case Study for Operational State Identification and Monitoring Using PCA
9.9.1 Case Study 1: Monitoring a Reciprocating Reclaim Compressor
References
10 Fault Diagnosis. 10.1 Challenges to the Chemical Industry
10.2 What is Fault Diagnosis?
10.3 Benefit of a Fault Diagnosis System
10.3.1 Characteristic of an Automated Fault Diagnosis System
10.4 Decreasing Downtime Through a Fault Diagnosis Type Data Analytics
10.5 User Perspective to Make an Effective Fault Diagnosis System
10.6 How Are Fault Diagnosis Systems Made?
10.6.1 Principal Component‐Based Approach
10.6.2 Artificial Neural Network‐Based Approach
10.7 A Case Study to Build a Robust Fault Diagnosis System
10.7.1 Challenges to a Build Fault Diagnosis of an Ethylene Oxide Reactor System
10.7.2 PCA‐Based Fault Diagnosis of an EO Reactor System
10.7.3 Acquiring Historic Process Data Sets to Build a PCA Model
10.7.4 Criteria of Selection of Input Parameters for PCA
10.7.5 How PCA Input Data is Captured in Real Time
10.7.6 Building the Model
10.7.6.1 Calculations of the Principal Components
10.7.6.2 Calculations of Hotelling's T2
10.7.6.3 Calculations of the Residual
10.7.7 Creation of a PCA Plot for Training Data
10.7.8 Creation of Hotelling's T2 Plot for the Training Data
10.7.9 Creation of a Residual Plot for the Training Data
10.7.10 Creation of an Abnormal Zone in the PCA Plot
10.7.11 Implementing the PCA Model in Real Time
10.7.12 Detecting Whether the Plant is Running Normally or Abnormally on a Real ‐Time Basis
10.7.13 Use of a PCA Plot During Corrective Action in Real Time
10.7.14 Validity of a PCA Model. 10.7.14.1 Time‐Varying Characteristic of an EO Catalyst
10.7.14.2 Capturing the Efficiency of the PCA Model Using the Residual Plot
10.7.15 Quantitive Decision Criteria Implemented for Retraining of an Ethylene Oxide (EO) Reactor PCA Model
10.7.16 How Retraining is Practically Executed
10.8 Building an ANN Model for Fault Diagnosis of an EO Reactor. 10.8.1 Acquiring Historic Process Data Sets to Build an ANN Model
10.8.2 Identification of Input and Output Parameters
10.8.3 Building of an ANN‐Based EO Reactor Model. 10.8.3.1 Complexity of EO Reactor Modeling
10.8.3.2 Model Building
10.8.4 Prediction Performance of an ANN Model
10.8.5 Utilization of an ANN Model for Fault Detection
10.8.6 How Do PCA Input Data Relate to ANN Input/Output Data?
10.8.7 Retraining of an ANN Model
10.9 Integrated Robust Fault Diagnosis System
10.10 Advantages of a Fault Diagnosis System
References
11 Optimization of an Existing Distillation Column. 11.1 Strategy to Optimize the Running Distillation Column
11.1.1 Strategy
11.2 Increase the Capacity of a Running Distillation Column
11.3 Capacity Diagram
11.4 Capacity Limitations of Distillation Columns
11.5 Vapour Handling Limitations. 11.5.1 Flow Regimes – Spray and Froth
11.5.2 Entrainment
11.5.3 Tray Flooding (Zhu, 2013)
11.5.4 Ultimate Capacity
11.6 Liquid Handling Limitations. 11.6.1 Downcomer Flood
11.6.2 Downcomer Residence Time
11.6.3 Downcomer Froth Back‐Up%
11.6.4 Downcomer Inlet Velocity
11.6.5 Weir liquid loading (Zhu, 2013)
11.6.6 Downcomer Sizing Criteria
11.7 Other Limitations and Considerations. 11.7.1 Weeping (Zhu, 2013)
11.7.2 Dumping
11.7.3 Tray Turndown
11.7.4 Foaming
11.8 Understanding the Stable Operation Zone (Zhu, 2013)
11.9 Case Study to Develop a Capacity Diagram
11.9.1 Calculation of Capacity Limits (Zhu, 2013) 11.9.1.1 Spray Limit
11.9.1.2 Vapor Flooding Limit (Zhu, 2013)
11.9.1.3 Downcomer Backup Limit (Zhu, 2013)
11.9.1.4 Maximum Liquid Loading Limit (Zhu, 2013)
11.9.1.5 Minimum Liquid Loading Limit
11.9.1.6 Minimum Vapor Loading Limit
11.9.2 Plotting a Capacity Diagram (Zhu, 2013)
11.9.3 Insights from the Capacity Diagram
11.9.4 How Can the Capacity Diagram Be Used for Profit Maximization?
References
12 New Design Methodology. 12.1 Need for New Design Methodology
12.2 Case Study of the New Design Methodology for a Distillation Column. 12.2.1 Traditional Way to Design a Distillation Column
12.2.2 Background of the Distillation Column Design
12.3 New Intelligent Methodology for Designing a Distillation Column
12.4 Problem Description of the Case Study
12.5 Solution Procedure Using the New Design Methodology
12.6 Calculations of the Total Cost
12.7 Search Optimization Variables
12.8 Operational and Hydraulic Constraints
12.9 Particle Swarm Optimization
12.9.1 PSO Algorithm
12.10 Simulation and PSO Implementation
12.11 Results and Analysis
12.12 Advantages of PSO
12.13 Advantages of New Methodology over the Traditional Approach (Lahiri, 2014)
12.14 Conclusion
Nomenclature
References
Appendix 12.1
13 Genetic Programing for Modeling of Industrial Reactors. 13.1 Potential Impact of Reactor Optimization on Overall Profit
13.2 Poor Knowledge of Reaction Kinetics of Industrial Reactors
13.3 ANN as a Tool for Reactor Kinetic Modeling
13.4 Conventional Methods for Evaluating Kinetics
13.5 What is Genetic Programming?
13.6 Background of Genetic Programming (Searson et al., 2011)
13.7 Genetic Programming at a Glance (Koza, 1992; Koza and Rice, 1992; Koza et al., 1999)
13.7.1 Preparatory Steps of Genetic Programming
13.7.2 Executional Steps of Genetic Programming
13.7.3 Creating an Individual
13.7.4 Fitness Test
13.7.5 The Genetic Operations
13.7.6 User Decisions
13.7.7 Computing Resources
13.8 Example Genetic Programming Run
13.8.1 Preparatory Steps
13.8.2 Step‐by‐Step Sample Run
13.8.3 Selection, Crossover, and Mutation
13.9 Case Studies
13.9.1 Case Study 1
13.9.2 Case Study 2
13.9.3 Case Study 3
13.9.4 Case Study 4
References
14 Maximum Capacity Test Run and Debottlenecking Study. 14.1 Introduction
14.2 Understanding Different Safety Margins in Process Equipment
14.3 Strategies to Exploit the Safety Margin
14.4 Capacity Expansion versus Efficiency Reduction
14.5 Maximum Capacity Test Run: What is it All About?
14.6 Objective of a Maximum Capacity Test Run
14.7 Bottlenecks of Different Process Equipment
14.7.1 Functional Bottleneck
14.7.2 Reliability Bottleneck
14.7.3 Safety Interlock Bottleneck
14.8 Key Steps to Carry Out a Maximum Capacity Test Run in a Commercial Running Plant
14.8.1 Planning
14.8.2 Discussion with Technical People
14.8.3 Risk and Opportunity
14.8.4 Dos and Don'ts
14.8.5 Simulations
14.8.6 Preparations
14.8.7 Management of Change
14.8.8 Execution
14.8.9 Data Collections
14.8.10 Critical Observations
14.8.11 Report Preparations
14.8.12 Detailed Simulations and Assembly of All Observations
14.8.13 Final Report Preparation
14.9 Scope and Phases of a Detailed Improvement Study
14.9.1 Improvement Scoping Study
14.9.2 Detail Feasibility Study
14.9.3 Retrofit Design Phase
14.10 Scope and Limitations of MCTR. 14.10.1 Scope
14.10.2 Two Big Benefits of Doing MCTR
14.10.3 Limitations of MCTR
15 Loss Assessment. 15.1 Different Losses from the System
15.2 Strategy to Reduce the Losses and Wastages
15.3 Money Loss Audit
15.4 Product or Utility Losses
15.4.1 Loss in the Drain
15.4.2 Loss Due to Vent and Flaring
15.4.3 Utility Loss
15.4.4 Heat Loss Assessment for the Fired Heater
15.4.5 Heat Loss Assessment for the Distillation Column
15.4.6 Heat Loss Assessment for Steam Leakage
15.4.7 Heat Loss Assessment for Condensate Loss
16 Advance Process Control. 16.1 What is Advance Process Control?
16.2 Why is APC Necessary to Improve Profit?
16.3 Why APC is Preferred over Normal PID Regulatory Control (Lahiri, 2017c)
16.4 Position of APC in the Control Hierarchy (Lahiri, 2017c)
16.5 Which are the Plants where Implementations of APC were Proven Very Profitable?
16.6 How do Implementations of APC Increase Profit?
16.7 How does APC Extract Benefits?
16.8 Application of APC in Oil Refinery, Petrochemical, Fertilizer and Chemical Plants and Related Benefits
16.9 Steps to Execute an APC Project (Lahiri, 2017d)
16.9.1 Step 1: Preliminary Cost –Benefit Analysis
16.9.2 Step 2: Assessment of Base Control Loops
16.9.3 Step 3: Functional Design of the Controller
16.9.4 Step 4: Conduct the Plant Step Test
16.9.5 Step 5: Generate a Process Model
16.9.6 Step 6: Commission the Online Controller
16.9.7 Step 7: Online APC Controller Tuning
16.10 How Can an Effective Functional Design Be Done?
16.10.1 Step 1: Define Process Control Objectives
16.10.2 Step 2: Identification of Process Constraints
16.10.3 Step 3: Define Controller Scope
16.10.4 Step 4: Variable Selection
16.10.5 Step 5: Rectify Regulatory Control Issues
16.10.6 Step 6: Explore the Scope of Inclusions of Inferential Calculations
16.10.7 Step 7: Evaluate Potential Optimization Opportunity
16.10.8 Step 8: Define LP or QP Objective Function
References
17 150 Ways and Best Practices to Improve Profit in Running Chemical Plant. 17.1 Best Practices Followed in Leading Process Industries Around the World
17.2 Best Practices Followed in a Steam and Condensate System
17.3 Best Practices Followed in Furnaces and Boilers
17.4 Best Practices Followed in Pumps, Fans, and Compressor
17.5 Best Practices Followed in Illumination Optimization
17.6 Best Practices in Operational Improvement
17.7 Best Practices Followed in Air and Nitrogen Header
17.8 Best Practices Followed in Cooling Tower and Cooling Water
17.9 Best Practices Followed in Water Conservation
17.10 Best Practices Followed in Distillation Column and Heat Exchanger
17.11 Best Practices in Process Improvement
17.12 Best Practices in Flare Gas Reduction
17.13 Best Practices in Product or Energy Loss Reduction
17.14 Best Practices to Monitor Process Control System Performance
17.15 Best Practices to Enhance Plant Reliability
17.16 Best Practices to Enhance Human Resource
17.17 Best Practices to Enhance Safety, Health, and the Environment
17.18 Best Practices to Use New Generation Digital Technology
17.19 Best Practices to Focus a Detailed Study and R&D Effort
Index
WILEY END USER LICENSE AGREEMENT
Отрывок из книги
Sandip Kumar Lahiri
National Institute Of Technology, Durgapur, India
.....
Running the plant at maximum capacity does not mean to run it at its nameplate capacity, i.e. process flow diagram (PFD) capacity. That is the bare minimum target. All over the world, good companies are running at 125–150% of their nameplate design capacity. Normally they follow three basic steps to increase plant capacity:
All good plants follow these three steps in order and continuously improve themselves so that with the same plant they can run 25–50% extra capacity. This is one of the surest ways to increase profit.
.....