Big Data
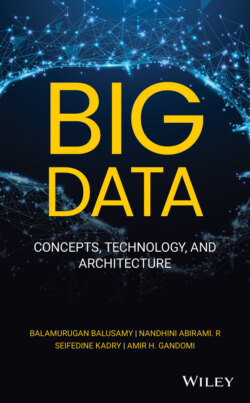
Реклама. ООО «ЛитРес», ИНН: 7719571260.
Оглавление
Seifedine Kadry. Big Data
Table of Contents
List of Tables
List of Illustrations
Guide
Pages
Big Data. Concepts, Technology, and Architecture
Acknowledgments
About the Author
1 Introduction to the World of Big Data. CHAPTER OBJECTIVE
1.1 Understanding Big Data
1.2 Evolution of Big Data
1.3 Failure of Traditional Database in Handling Big Data
1.3.1 Data Mining vs. Big Data
1.4 3 Vs of Big Data
1.4.1 Volume
1.4.2 Velocity
1.4.3 Variety
1.5 Sources of Big Data
1.6 Different Types of Data
1.6.1 Structured Data
1.6.2 Unstructured Data
1.6.3 Semi‐Structured Data
1.7 Big Data Infrastructure
1.8 Big Data Life Cycle
1.8.1 Big Data Generation
1.8.2 Data Aggregation
1.8.3 Data Preprocessing
1.8.3.1 Data Integration
1.8.3.2 Data Cleaning
1.8.3.3 Data Reduction
1.8.3.4 Data Transformation
1.8.4 Big Data Analytics
1.8.5 Visualizing Big Data
1.9 Big Data Technology
1.9.1 Challenges Faced by Big Data Technology
1.9.2 Heterogeneity and Incompleteness
1.9.3 Volume and Velocity of the Data
1.9.4 Data Storage
1.9.5 Data Privacy
1.10 Big Data Applications
1.11 Big Data Use Cases. 1.11.1 Health Care
1.11.2 Telecom
1.11.3 Financial Services
Chapter 1 Refresher
Conceptual Short Questions with Answers
Frequently Asked Interview Questions
2 Big Data Storage Concepts. CHAPTER OBJECTIVE
2.1 Cluster Computing
2.1.1 Types of Cluster
2.1.1.1 High Availability Cluster
2.1.1.2 Load Balancing Cluster
2.1.2 Cluster Structure
2.2 Distribution Models
2.2.1 Sharding
2.2.2 Data Replication
2.2.2.1 Master‐Slave Model
2.2.2.2 Peer‐to‐Peer Model
2.2.3 Sharding and Replication
2.3 Distributed File System
2.4 Relational and Non‐Relational Databases
2.4.1 RDBMS Databases
2.4.2 NoSQL Databases
2.4.3 NewSQL Databases
2.4.3.1 Clustrix
2.4.3.2 NuoDB
2.4.3.3 VoltDB
2.4.3.4 MemSQL
2.5 Scaling Up and Scaling Out Storage
Chapter 2 Refresher
Conceptual Short Questions with Answers
3 NoSQL Database. CHAPTER OBJECTIVE
3.1 Introduction to NoSQL
3.2 Why NoSQL
3.3 CAP Theorem
3.4 ACID
3.5 BASE
3.6 Schemaless Databases
3.7 NoSQL (Not Only SQL)
3.7.1 NoSQL vs. RDBMS
3.7.2 Features of NoSQL Databases
3.7.3 Types of NoSQL Technologies
3.7.3.1 Key‐Value Store Database
3.7.3.1.1 Amazon DynamoDB
3.7.3.1.2 Microsoft Azure Table Storage
3.7.3.2 Column‐Store Database
3.7.3.2.1 Apache Cassandra
3.7.3.3 Document‐Oriented Database
3.7.3.3.1 CouchDB
3.7.3.4 Graph‐Oriented Database
3.7.3.4.1 Neo4J
3.7.3.4.2 Cypher Query Language (CQL)
3.7.4 NoSQL Operations
3.8 Migrating from RDBMS to NoSQL
Chapter 3 Refresher
Conceptual Short Questions with Answers
4 Processing, Management Concepts, and Cloud Computing: Part I: Big Data Processing and Management Concepts. CHAPTER OBJECTIVE
4.1 Data Processing
4.2 Shared Everything Architecture
4.2.1 Symmetric Multiprocessing Architecture
4.2.2 Distributed Shared Memory
4.3 Shared‐Nothing Architecture
4.4 Batch Processing
4.5 Real‐Time Data Processing
4.6 Parallel Computing
4.7 Distributed Computing
4.8 Big Data Virtualization
4.8.1 Attributes of Virtualization
4.8.1.1 Encapsulation
4.8.1.2 Partitioning
4.8.1.3 Isolation
4.8.2 Big Data Server Virtualization
Part II: Managing and Processing Big Data in Cloud Computing. 4.9 Introduction
4.10 Cloud Computing Types
4.11 Cloud Services
4.12 Cloud Storage
4.12.1 Architecture of GFS
4.12.1.1 Master
4.12.1.2 Client
4.12.1.3 Chunk
4.12.1.4 Read Algorithm
4.12.1.5 Write Algorithm
4.13 Cloud Architecture
4.13.1 Cloud Challenges
Chapter 4 Refresher
Conceptual Short Questions with Answers
Cloud Computing Interview Questions
Chapter 5 Driving Big Data with Hadoop Tools and Technologies. CHAPTER OBJECTIVE
5.1 Apache Hadoop
5.1.1 Architecture of Apache Hadoop
5.1.2 Hadoop Ecosystem Components Overview
5.2 Hadoop Storage. 5.2.1 HDFS (Hadoop Distributed File System)
5.2.2 Why HDFS?
5.2.3 HDFS Architecture
5.2.4 HDFS Read/Write Operation
5.2.5 Rack Awareness
5.2.6 Features of HDFS. 5.2.6.1 Cost‐Effective
5.2.6.2 Distributed Storage
5.2.6.3 Data Replication
5.3 Hadoop Computation. 5.3.1 MapReduce
5.3.1.1 Mapper
5.3.1.2 Combiner
5.3.1.3 Reducer
5.3.1.4 JobTracker and TaskTracker
5.3.2 MapReduce Input Formats
5.3.3 MapReduce Example
5.3.4 MapReduce Processing
5.3.5 MapReduce Algorithm
5.3.6 Limitations of MapReduce
5.4 Hadoop 2.0
5.4.1 Hadoop 1.0 Limitations
5.4.2 Features of Hadoop 2.0
5.4.3 Yet Another Resource Negotiator (YARN)
5.4.4 Core Components of YARN
5.4.4.1 ResourceManager
5.4.4.2 NodeManager
5.4.5 YARN Scheduler
5.4.5.1 FIFO Scheduler
5.4.5.2 Capacity Scheduler
5.4.5.3 Fair Scheduler
5.4.6 Failures in YARN
5.4.6.1 ResourceManager Failure
5.4.6.2 ApplicationMaster Failure
5.4.6.3 NodeManager Failure
5.4.6.4 Container Failure
5.5 HBASE
5.5.1 Features of HBase
5.6 Apache Cassandra
5.7 SQOOP
5.8 Flume
5.8.1 Flume Architecture
5.8.1.1 Event
5.8.1.2 Agent
5.9 Apache Avro
5.10 Apache Pig
5.11 Apache Mahout
5.12 Apache Oozie
5.12.1 Oozie Workflow
5.12.2 Oozie Coordinators
5.12.3 Oozie Bundles
5.13 Apache Hive
5.14 Hive Architecture
5.15 Hadoop Distributions
Chapter 5 Refresher
Conceptual Short Questions with Answers
Frequently Asked Interview Questions
6 Big Data Analytics. CHAPTER OBJECTIVE
6.1 Terminology of Big Data Analytics. 6.1.1 Data Warehouse
6.1.2 Business Intelligence
6.1.3 Analytics
6.2 Big Data Analytics
6.2.1 Descriptive Analytics
6.2.2 Diagnostic Analytics
6.2.3 Predictive Analytics
6.2.4 Prescriptive Analytics
6.3 Data Analytics Life Cycle
6.3.1 Business Case Evaluation and Identification of the Source Data
6.3.2 Data Preparation
6.3.3 Data Extraction and Transformation
6.3.4 Data Analysis and Visualization
6.3.5 Analytics Application
6.4 Big Data Analytics Techniques
6.4.1 Quantitative Analysis
6.4.2 Qualitative Analysis
6.4.3 Statistical Analysis
6.4.3.1 A/B Testing
6.4.3.2 Correlation
6.4.3.3 Regression
6.5 Semantic Analysis
6.5.1 Natural Language Processing
6.5.2 Text Analytics
6.5.3 Sentiment Analysis
6.6 Visual analysis
6.7 Big Data Business Intelligence
6.7.1 Online Transaction Processing (OLTP)
6.7.2 Online Analytical Processing (OLAP)
6.7.3 Real‐Time Analytics Platform (RTAP)
6.8 Big Data Real‐Time Analytics Processing
6.9 Enterprise Data Warehouse
Chapter 6 Refresher
Conceptual Short Questions with Answers
7 Big Data Analytics with Machine Learning. CHAPTER OBJECTIVE
7.1 Introduction to Machine Learning
7.2 Machine Learning Use Cases
7.3 Types of Machine Learning
7.3.1 Supervised Machine Learning Algorithm
7.3.1.1 Classification
7.3.1.2 Regression
Linear Regression
7.3.1.2.1 Logistic Regression
7.3.2 Support Vector Machines (SVM)
7.3.3 Unsupervised Machine Learning
7.3.4 Clustering
Chapter 7 Refresher
Conceptual Short Questions with Answers
8 Mining Data Streams and Frequent Itemset. CHAPTER OBJECTIVE
8.1 Itemset Mining
Exercise 1: Frequent Itemset Mining Using R
8.2 Association Rules
Exercise 8.1
8.3 Frequent Itemset Generation
8.4 Itemset Mining Algorithms
8.4.1 Apriori Algorithm
Exercise—Implementation of Apriori Algorithm Using R
Exercise 8.1
8.4.1.1 Frequent Itemset Generation Using the Apriori Algorithm
8.4.2 The Eclat Algorithm—Equivalence Class Transformation Algorithm
Exercise‐ Eclat Algorithm Implementation Using R
8.4.3 The FP Growth Algorithm
8.5 Maximal and Closed Frequent Itemset
Exercise 8.2
8.6 Mining Maximal Frequent Itemsets: the GenMax Algorithm
8.7 Mining Closed Frequent Itemsets: the Charm Algorithm
8.8 CHARM Algorithm Implementation
8.9 Data Mining Methods
8.10 Prediction
8.10.1 Classification Techniques
8.10.1.1 Bayesian Network
8.11 Important Terms Used in Bayesian Network. 8.11.1 Random Variable
8.11.2 Probability Distribution
8.11.3 Joint Probability Distribution
8.11.4 Conditional Probability
Exercise Problem:
8.11.5 Independence
8.11.6 Bayes Rule
8.11.6.1 K‐Nearest Neighbor Algorithm
8.11.6.1.1 The Distance Metric
8.11.6.1.2 The Parameter Selection – Cross Validation
8.11.6.2 Decision Tree Classifier
8.12 Density Based Clustering Algorithm
8.13 DBSCAN
8.14 Kernel Density Estimation
8.14.1 Artificial Neural Network
8.14.2 The Biological Neural Network
8.15 Mining Data Streams
8.16 Time Series Forecasting
9 Cluster Analysis. 9.1 Clustering
9.2 Distance Measurement Techniques
9.3 Hierarchical Clustering
9.3.1 Application of Hierarchical Methods
9.4 Analysis of Protein Patterns in the Human Cancer‐Associated Liver
9.5 Recognition Using Biometrics of Hands. 9.5.1 Partitional Clustering
9.5.2 K‐Means Algorithm
9.5.3 Kernel K‐Means Clustering
9.6 Expectation Maximization Clustering Algorithm
9.7 Representative‐Based Clustering
9.8 Methods of Determining the Number of Clusters. 9.8.1 Outlier Detection
9.8.2 Types of Outliers
9.8.3 Outlier Detection Techniques
9.8.4 Training Dataset–Based Outlier Detection
9.8.5 Assumption‐Based Outlier Detection
9.8.6 Applications of Outlier Detection
9.9 Optimization Algorithm
9.10 Choosing the Number of Clusters
9.11 Bayesian Analysis of Mixtures
9.12 Fuzzy Clustering
9.13 Fuzzy C‐Means Clustering
10 Big Data Visualization. CHAPTER OBJECTIVE
10.1 Big Data Visualization
10.2 Conventional Data Visualization Techniques
10.2.1 Line Chart
10.2.2 Bar Chart
10.2.3 Pie Chart
10.2.4 Scatterplot
10.2.5 Bubble Plot
10.3 Tableau
10.3.1 Connecting to Data
10.3.2 Connecting to Data in the Cloud
10.3.3 Connect to a File
10.3.4 Scatterplot in Tableau
10.3.5 Histogram Using Tableau
10.4 Bar Chart in Tableau
10.5 Line Chart
10.6 Pie Chart
10.7 Bubble Chart
10.8 Box Plot
10.9 Tableau Use Cases. 10.9.1 Airlines
10.9.2 Office Supplies
10.9.3 Sports
10.9.4 Science – Earthquake Analysis
10.10 Installing R and Getting Ready
10.10.1 R Basic Commands
10.10.2 Assigning Value to a Variable
10.11 Data Structures in R
10.11.1 Vector
10.11.2 Coercion
10.11.3 Length, Mean, and Median
10.11.4 Matrix
10.11.5 Arrays
10.11.6 Naming the Arrays
10.11.7 Data Frames
10.11.8 Lists
10.12 Importing Data from a File
10.13 Importing Data from a Delimited Text File
10.14 Control Structures in R
10.14.1 If‐else
10.14.2 Nested if‐Else
10.14.3 For Loops
10.14.4 While Loops
10.14.5 Break
10.15 Basic Graphs in R
10.15.1 Pie Charts
10.15.2 3D – Pie Charts
10.15.3 Bar Charts
10.15.4 Boxplots
10.15.5 Histograms
10.15.6 Line Charts
10.15.7 Scatterplots
Index. a
b
c
d
e
f
g
h
i
j
k
l
m
n
o
p
q
r
s
t
u
v
w
y
WILEY END USER LICENSE AGREEMENT
Отрывок из книги
Balamurugan Balusamy, Nandhini Abirami. R, Seifedine Kadry, and Amir H. Gandomi
.....
The first phase of the life cycle of big data is the data generation. The scale of data generated from diversified sources is gradually expanding. Sources of this large volume of data were discussed under the Section 1.5, “Sources of Big Data.”
Figure 1.10 Big data life cycle.
.....