Bayesian Risk Management
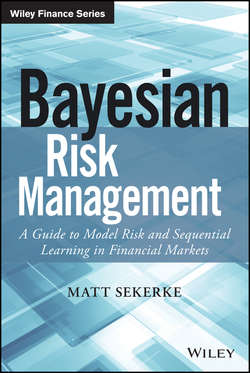
Реклама. ООО «ЛитРес», ИНН: 7719571260.
Оглавление
Sekerke Matt. Bayesian Risk Management
Preface
Acknowledgments
Chapter 1. Models for Discontinuous Markets
Risk Models and Model Risk
Time-Invariant Models and Crisis
Bayesian Probability as a Means of Handling Discontinuity
Time-Invariance and Objectivity
Part One. Capturing Uncertainty in Statistical Models
Chapter 2. Prior Knowledge, Parameter Uncertainty, and Estimation
Estimation with Prior Knowledge: The Beta-Bernoulli Model
Prior Parameter Distributions as Hypotheses: The Normal Linear Regression Model
Decisions after Observing the Data: The Choice of Estimators
Chapter 3. Model Uncertainty
Bayesian Model Comparison
Models as Nuisance Parameters
Uncertainty in Pricing Models
A Note on Backtesting
Part Two. Sequential Learning with Adaptive Statistical Models
Chapter 4. Introduction to Sequential Modeling
Sequential Bayesian Inference
Achieving Adaptivity via Discounting
Accounting for Uncertainty in Sequential Models
Chapter 5. Bayesian Inference in State-Space Time Series Models
State-Space Models of Time Series
Dynamic Linear Models
Recursive Relationships in the DLM
Variance Estimation
Sequential Model Comparison
Chapter 6. Sequential Monte Carlo Inference
Nonlinear and Non-Normal Models
State Learning with Particle Filters
Joint Learning of Parameters and States
Sequential Model Comparison
Part Three. Sequential Models of Financial Risk
Chapter 7. Volatility Modeling
Single-Asset Volatility
Volatility for Multiple Assets
Chapter 8. Asset-Pricing Models and Hedging
Derivative Pricing in the Schwartz Model
Online State-Space Model Estimates of Derivative Prices
Models for Portfolios of Assets
Part Four. Bayesian Risk Management
Chapter 9. From Risk Measurement to Risk Management
Results
Prior Information as an Instrument of Corporate Governance
References
Отрывок из книги
MATT SEKERKE
Uncertainty about the future stems from our limited ability to specify risk models, estimate their parameters from data, and be assured of the continuity between today's markets and tomorrow's markets. Ignoring any of these dimensions of model risk creates an illusion of mastery and fosters erroneous decision making. It is typical for financial firms to ignore all of these sources of uncertainty. Because they measure too little risk, they take on too much risk.
.....
The second experience was my involvement in the early stages of litigation related to the credit crisis. In these lawsuits, a few questions were on everyone's mind. Could the actors in question have seen significant changes in the market coming? If so, at what point could they have known that a collapse was imminent? If not, what would have led them to believe that the future was either benign or unknowable? The opportunity to review confidential information obtained in the discovery phase of these litigations provided innumerable insights into the inner workings of the key actors with respect to risk measurement, risk management, and financial instrument valuation. I saw two main things. First, there was an overwhelming dependence on front-office information – bid sheets, a few consummated secondary-market trades, and an overwhelming amount of “market color,” the industry term for the best rumor and innuendo on offer – and almost no dependence on middle-office modeling. Whereas certain middle-office modeling efforts could have reacted to changes in market conditions, the traders on the front lines would not act until they saw changes in traded prices. Second, there were interminable discussions about how to weigh new data on early-stage delinquencies, default rates, and home prices against historical data. Instead of asking whether the new data falsified earlier premises on which expectations were built, discussions took place within the bounds of the worst-known outcomes from history, with the unstated assurance that housing market phenomena were stable and mean-reverting overall. Whatever these observations might imply about the capacity of the actors involved, it seemed that a better balance could be struck between middle-office risk managers and front-office traders, and that gains could be had by making the expectations of all involved explicit in the context of models grounded in the relevant fundamentals.
However, it was not until I began my studies at the University of Chicago that these themes converged around the technical means necessary to make them concrete. Nick Polson's course in probability theory was a revelation, introducing the Bayesian approach to probability within the context of financial markets. Two quarters of independent study with him followed immediately in which he introduced me to the vanguard of Bayesian thinking about time series. A capstone elective on Bayesian econometrics with Hedibert Lopes provided further perspective and rigor. His teaching was a worthy continuation of a tradition at the University of Chicago going back to Arnold Zellner.
.....