Sharing Economy and Big Data Analytics
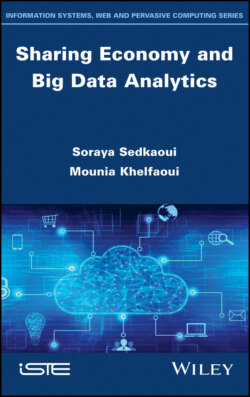
Реклама. ООО «ЛитРес», ИНН: 7719571260.
Оглавление
Soraya Sedkaoui. Sharing Economy and Big Data Analytics
Table of Contents
List of Tables
List of Illustrations
Guide
Pages
Sharing Economy and Big Data Analytics
Preface
Introduction
I.1. Why this book?
I.2. The scope of this book
I.3. The challenge of this book
I.4. How to read this book
Part 1: The shared economy or the emergence of a new business model
Part 2: Big Data analytics at the service of the sharing economy
Part 3: The sharing economy? Not without the Big Data algorithms
1. The Sharing Economy: A Concept Under Construction
1.1. Introduction
1.2. From simple sharing to the sharing economy
1.2.1. The genesis of the sharing economy and the break with “consumer” society
1.2.2. The sharing economy: which economy?
1.3. The foundations of the sharing economy
1.3.1. Peer-to-peer (P2P): a revolution in computer networks
Box 1.1.The social web
Box 1.2.IP4 address
1.3.2. The gift: the abstract aspect of the sharing economy
Box 1.3.The gift according to Mauss
1.3.3. The service economy and the offer of use
Box 1.4.The service economy (ADEME 2017)
Box 1.5.Xerox and Michelin’s service economy
1.4. Conclusion
2. An Opportunity for the Business World
2.1. Introduction
2.2. Prosumption: a new sharing economy trend for the consumer
Box 2.1.“McDonalization” phenomenon of society
2.3. Poverty: a target in the spotlight of the shared economy
Box 2.2.Poverty’s two perspectives
2.4. Controversies on economic opportunities of the sharing economy
Box 2.3.Strong points of Internet use
Box 2.4.Meaning of the different transaction methods
Box 2.5.Lean start-up
2.5. Conclusion
3. Risks and Issues of the Sharing Economy
3.1. Introduction
3.2. Uberization: a white grain or just a summer breeze?
Box 3.1.Creative destruction (Raies 2012)
Box 3.2.A brief history of Uber (Lechien and Tinel 2016; O’toole and Matherne 2017)
3.3. The sharing economy: a disruptive model
Box 3.3.Examples of companies swept away by the wave of disruptive technologies (Joseph and Christensen 1995)
Box 3.4.The main definitions of the “uberization” concept (Lechien and Tinel 2016)
3.4. Major issues of the sharing economy
Box 3.5.The 19 proposals on the development of the sharing economy
3.5. Conclusion
4. Digital Platforms and the Sharing Mechanism
4.1. Introduction
4.2. Digital platforms: “What growth!”
Box 4.1.Bitcoin2
4.3. Digital platforms or technology at the service of the economy
Box 4.2.Coase’s transaction cost theory (2005)
4.4. From the sharing economy to the sharing platform economy
Box 4.3.Evolution of the turnover of collaborative platforms (PwC 2016)
4.5. Conclusion
5. Beyond the Word “Big”: The Changes
5.1. Introduction
5.2. The 3 Vs and much more: volume, variety, velocity
5.2.1. Volume
5.2.2. The variety
5.2.3. Velocity
5.2.4. What else?
5.3. The growth of computing and storage capacities
5.3.1. Big Data versus Big Computing
Box 5.1.Examples of Big Data technologies used
5.3.2. Big Data storage
5.3.3. Updating Moore’s Law
5.4. Business context change in the era of Big Data
5.4.1. The decision-making process and the dynamics of value creation
Box 5.2.Data in Porter’s value chain
5.4.2. The emergence of new data-driven business models
5.5. Conclusion
6. The Art of Analytics
6.1. Introduction
6.2. From simple analysis to Big Data analytics
Box 6.1.Analytics: from version 1.0 to version 3.0
6.2.1. Descriptive analysis: learning from past behavior to influence future outcomes
6.2.2. Predictive analysis: analyzing data to predict future outcomes
Box 6.2.Sentiment analysis: a common type of predictive analysis
6.2.3. Prescriptive analysis: recommending one or more action plan(s)
6.2.4. From descriptive analysis to prescriptive analysis: an example
Box 6.3.A solution for optimization problems
6.3. The process of Big Data analytics: from the data source to its analysis
Box 6.4.Knowledge discovery in databases (KDD)
6.3.1. Definition of objectives and requirements
6.3.2. Data collection
6.3.3. Data preparation
6.3.3.1. Missing values
6.3.3.2. Outliers
6.3.3.3. Errors
6.3.4. Exploration and interpretation
6.3.5. Modeling
6.3.5.1. Testing the model’s performance
6.3.5.2. Model optimization
6.3.6. Deployment
6.4. Conclusion
7. Data and Platforms in the Sharing Context
7.1. Introduction
7.2. Pioneers in Big Data
Box 7.1.Datacenter1
7.2.1. Big Data on Walmart’s shelves
7.2.2. The Big Data behind Netflix’s success story
7.2.3. The Amazon version of Big Data
7.2.4. Big data and social networks: the case of Facebook
Box 7.2.Cookies
7.2.5. IBM and data analysis in the health sector
7.3. Data, essential for sharing
7.3.1. Data and platforms at the heart of the sharing economy
7.3.2. The data of sharing economy companies
Box 7.3.Blockchain technology (Zahadat and Partridge 2018)
7.3.3. Privacy and data security in a sharing economy
7.3.4. Open Data and platform data sharing
7.4. Conclusion
8. Big Data Analytics Applied to the Sharing Economy
8.1. Introduction
8.2. Big Data and Machine Learning algorithms serving the sharing economy
8.2.1. Machine Learning algorithms
8.2.2. Algorithmic applications in the sharing economy context
8.3. Big Data technologies: the sharing economy companies’ toolbox
8.3.1. The appearance of a new concept and the creation of new technologies
8.3.1.1. The Hadoop ecosystem
8.3.1.2. Apache Spark
8.3.1.3. NoSQL databases
8.3.1.4. In-memory databases
8.3.1.5. Keep in mind
8.4. Big Data on the agenda of sharing economy companies
8.4.1. Uber
8.4.2. Airbnb
8.4.3. BlaBlaCar
8.4.4. Lyft
8.4.5. Yelp
8.4.6. Other cases
8.4.6.1. TaskRabbit
8.4.6.2. LaZooz
8.4.6.3. Mobike and Ofo
8.4.6.4. Other models based on data analysis
8.5. Conclusion
9. Linear Regression
9.1. Introduction
9.2. Linear regression: an advanced analysis algorithm
9.2.1. How are regression problems identified?
9.2.2. The linear regression model
Box 9.1.Gradient Descent
9.2.3. Minimizing modeling error
9.3. Other regression methods
9.3.1. Logistic regression
9.3.2. Additional regression models: regularized regression
9.3.2.1. Ridge regression
9.3.2.2. Lasso regression
9.4. Building your first predictive model: a use case
9.4.1. What variables help set a rental price on Airbnb?
9.4.1.1. Data preparation
9.4.1.2. Exploratory analysis
Box 9.2.The Pearson correlation coefficient
9.4.1.3. Modeling
Box 9.3.RMSE (Root Mean Squared Error)
9.5. Conclusion
10. Classification Algorithms
10.1. Introduction
10.2. A tour of classification algorithms
10.2.1. Decision trees
10.2.1.1. The structure of the decision tree
10.2.1.2. How the algorithm works
10.2.2. Naïve Bayes
10.2.2.1. The applications of the algorithm
10.2.2.2. Operation of the Naïve Bayes algorithm
Box 10.1.Bayes’ theorem
10.2.3. Support Vector Machine (SVM)
10.2.3.1. Definition of SVM
10.2.3.2. SVM: how it works
10.2.4. Other classification algorithms
10.2.4.1. The k-nearest neighbors (kNN)
10.2.4.2. Random Forest
Box 10.2.Bagging or bootstrap aggregation
10.2.4.3. Neural networks
Box 10.3.Deep Learning
10.3. Modeling Airbnb prices with classification algorithms
10.3.1. The work that’s already been done: overview
10.3.2. Models based on trees: decision tree versus Random Forest
10.3.2.1. Decision trees
10.3.2.2. Modeling using Random Forest
10.3.3. Price prediction with kNN
10.4. Conclusion
11. Cluster Analysis
11.1. Introduction
11.2. Cluster analysis: general framework
11.2.1. Cluster analysis applications
11.2.2. The clustering algorithm and the similarity measure
11.3. Grouping similar objects using k-means
11.3.1. The k-means algorithm
Box 11.1.Cluster analysis based on a prototype
11.3.1.1. Choosing the value of k
11.3.1.2. Assign each data group to a centroid
11.3.1.3. Assign each point to a class
11.3.1.4. Update the representatives for each class
11.3.2. Determine the number of clusters
11.3.2.1. Categorical data
11.3.2.2. The definition of the number of clusters
11.4. Hierarchical classification
11.4.1. The hierarchical model approach
11.4.2. Dendrograms
Box 11.2.Ward criterion
11.5. Discovering hidden structures with clustering algorithms
11.5.1. Illustration of the classification of prices based on different characteristics using the k-means algorithm
11.5.2. Identify the number of clusters k
Box 11.3.The elbow criterion
11.6. Conclusion
Conclusion
References
Index. A
B
C
D
E
F
G
H, I
J, K
L
M, N
O
Q, R
S
T
U, V
W
X, Y, Z
WILEY END USER LICENSE AGREEMENT
Отрывок из книги
Series Editor
Jean-Charles Pomerol
.....
Most of the time, gifting is not approached from an economic perspective, but rather from a socio-philosophical point of view. Thus, “a gift is a privileged object of anthropology and economic sociology since the essay on gifting by M. Mauss”5 (Athané 2008).
“[…] You will then have a fairly good idea of the kind of economy that is at present laboriously in gestation. We see it already functioning in certain economic groupings, and in the hearts of the masses, who possess very often better than their leaders, a sense of their own interests, and of the common interest. Perhaps by studying these obscure aspects of social life, we shall succeed in throwing a little light upon the path that our nations must follow, both in their morality and in their economy.”
.....